Safer Reinforcement Learning with Counterexample-guided Offline Training
ICLR 2023(2023)
摘要
Safe reinforcement learning (RL) aims at addressing the limitation of reinforcement learning in safety-critical scenarios, where failures during learning may incur high costs. Several methods exist to incorporate external knowledge or to use proximal sensor data to limit the exploration of unsafe states. However, dealing with (partially) unknown environments and dynamics, where an agent must discover safety threats during exploration, remains challenging. In this paper, we propose a method to abstract hybrid continuous-discrete systems into compact surrogate models representing the safety-relevant knowledge acquired by the agent at any time during exploration. We exploit probabilistic counterexamples generation to synthesise minimal, partial simulation environments from the surrogate model where the agent can train offline to produce heuristic strategies to minimise the risk of visiting unsafe states during subsequent online exploration. We demonstrate our method's effectiveness in increasing the agent's exploration safety on a selection of OpenAI Gym benchmarks.
更多查看译文
AI 理解论文
溯源树
样例
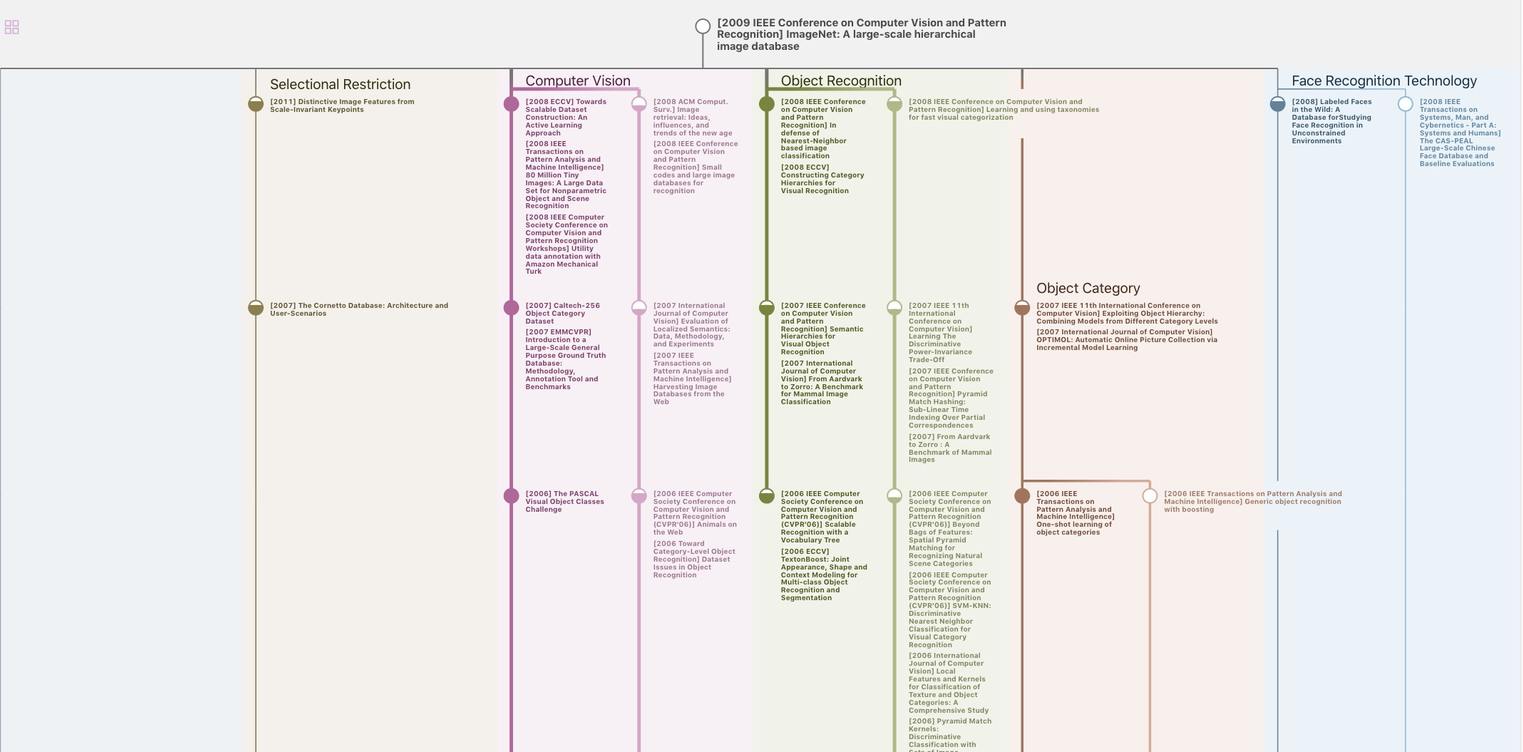
生成溯源树,研究论文发展脉络
Chat Paper
正在生成论文摘要