SaMoE: Parameter Efficient MoE Language Models via Self-Adaptive Expert Combination
ICLR 2023(2023)
摘要
Recently, Mixture-of-Experts (MoE) has demonstrated success in scaling models to have large amounts of parameters without significant increases in computational cost. However, MoEs have been also reported to be parameter inefficient such that larger models do not always lead to better performance.
In this work, we study how to build parameter-efficient MoE models. Our analysis identifies that MoE layers exhibit poor gradient flow as the number of experts increases, leading to insufficient training of experts. To overcome this issue, we propose a new MoE architecture design (SaMoE), which improves the parameter efficiency of MoE models by learning a soft combination of a global set of expert layers for each MoE layer. Such a scheme enables substantial parameter savings on MoE while achieving comparable or better accuracy than the standard MoE training baseline. Extensive experiments on billion-scale GPT-3 style autoregressive MoE language models demonstrate that SaMoE significantly improves the parameter efficiency of MoE models by reducing up to 5.2X total parameters while obtaining superior pre-training and zero-shot generalization results as compared to baseline.
更多查看译文
关键词
Mixture-of-Expert,Autoregressive language model,Parameter efficiency.
AI 理解论文
溯源树
样例
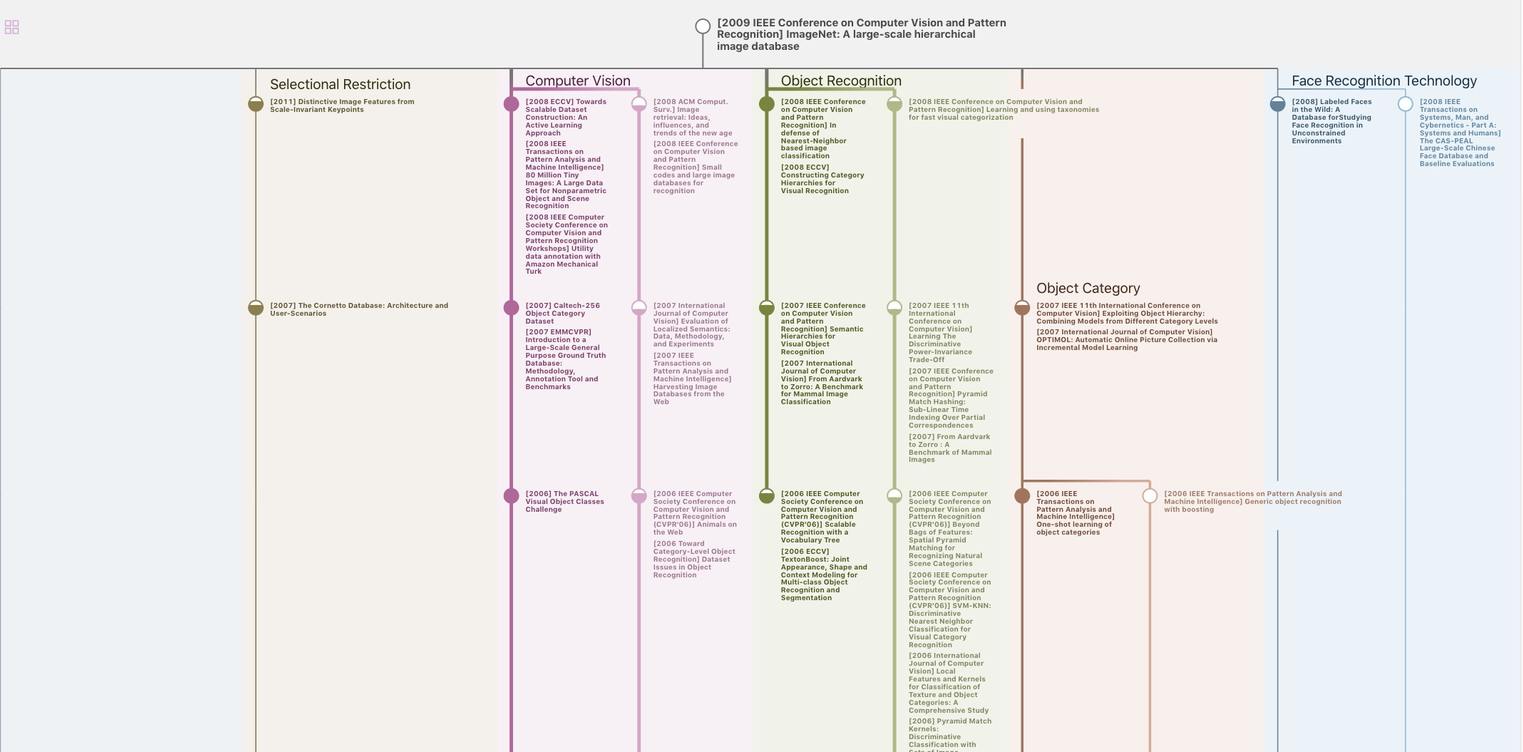
生成溯源树,研究论文发展脉络
Chat Paper
正在生成论文摘要