Self-supervised Continual Learning based on Batch-mode Novelty Detection
ICLR 2023(2023)
摘要
Continual learning (CL) plays a key role in dynamic systems in order to adapt to new tasks, while preserving previous knowledge. Most existing CL approaches focus on learning new knowledge in a supervised manner, while leaving the data gathering phase to the novelty detection (ND) algorithm. Such presumption limits the practical usage where new data needs to be quickly learned without being labeled. In this paper, we propose a unified approach of CL and ND, in which each new class of the out-of-distribution (ODD) data is first detected and then added to previous knowledge. Our method has three unique features: (1) a unified framework seamlessly tackling both ND and CL problems; (2) a self-supervised method for model adaptation, without the requirement of new data annotation; (3) batch-mode data feeding that maximizes the separation of new knowledge vs. previous learning, which in turn enables high accuracy in continual learning. By learning one class at each step, the new method achieves robust continual learning and consistently outperforms state-of-the-art CL methods in the single-head evaluation on MNIST, CIFAR-10, CIFAR-100 and TinyImageNet datasets.
更多查看译文
关键词
Continual Learning,Gradients-based,Mahalanobis Distance,Novelty Detection,out-of-distribution,self-supervised
AI 理解论文
溯源树
样例
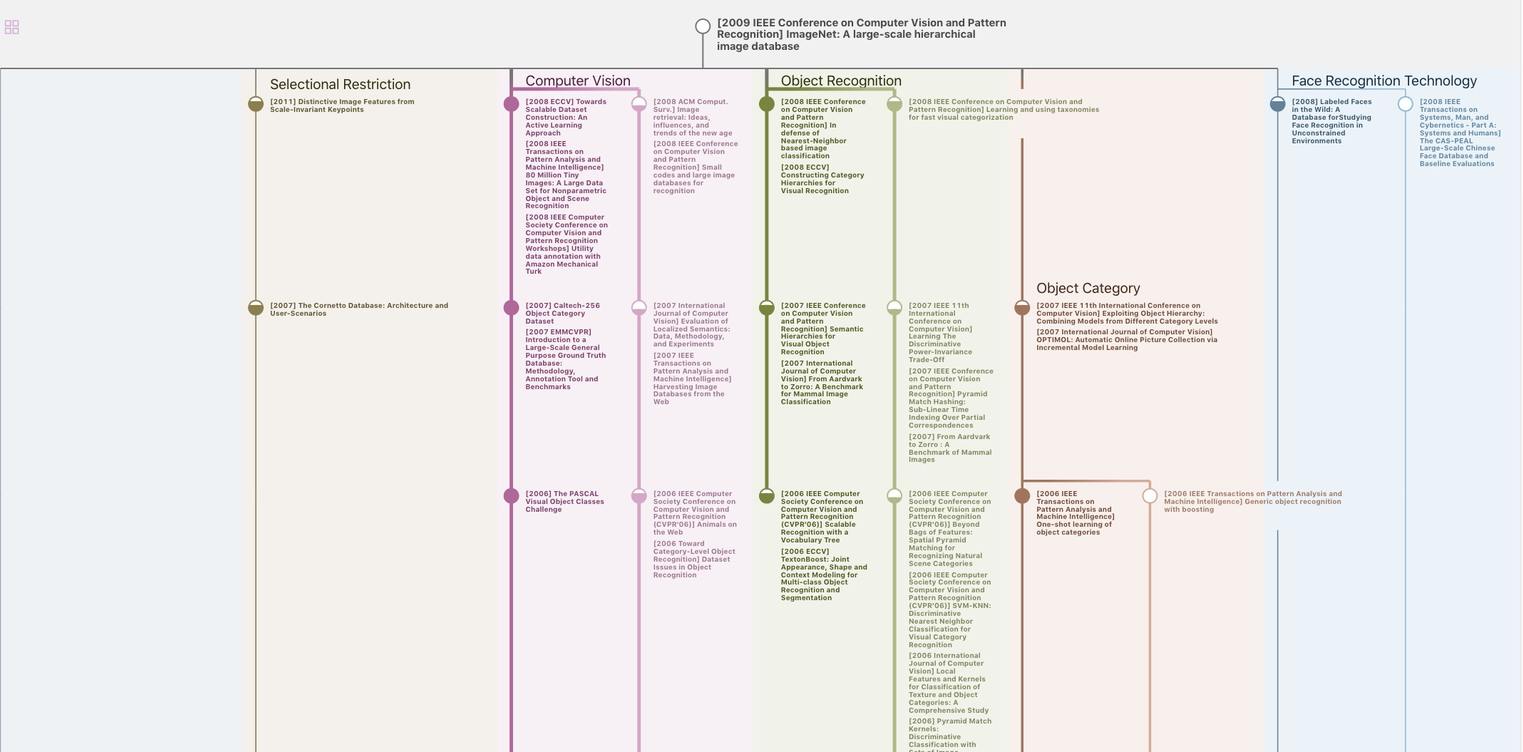
生成溯源树,研究论文发展脉络
Chat Paper
正在生成论文摘要