A Quasistatic Derivation of Optimization Algorithms' Exploration on Minima Manifolds
ICLR 2023(2023)
摘要
A quasistatic approach is proposed to derive the optimization algorithms' effective dynamics on the manifold of minima when the iterator oscillates around the manifold. Compared with existing strict analysis, our derivation method is simple and intuitive, has wide applicability, and produces easy-to-interpret results. As examples, we derive the manifold dynamics for SGD, SGD with momentum (SGDm) and Adam with different noise covariances, and justify the closeness of the derived manifold dynamics with the true dynamics through numerical experiments. We then use minima manifold dynamics to study and compare the properties of optimization algorithms. For SGDm, we show that scaling up learning rate and batch size simultaneously accelerates exploration without affecting generalization, which confirms a benefit of large batch training. For Adam, we show that the speed of its manifold dynamics changes with the direction of the manifold, because Adam is not rotationally invariant. This may cause slow exploration in high dimensional parameter spaces.
更多查看译文
关键词
Implicit bias,minima manifold,time-scale separation,Adam
AI 理解论文
溯源树
样例
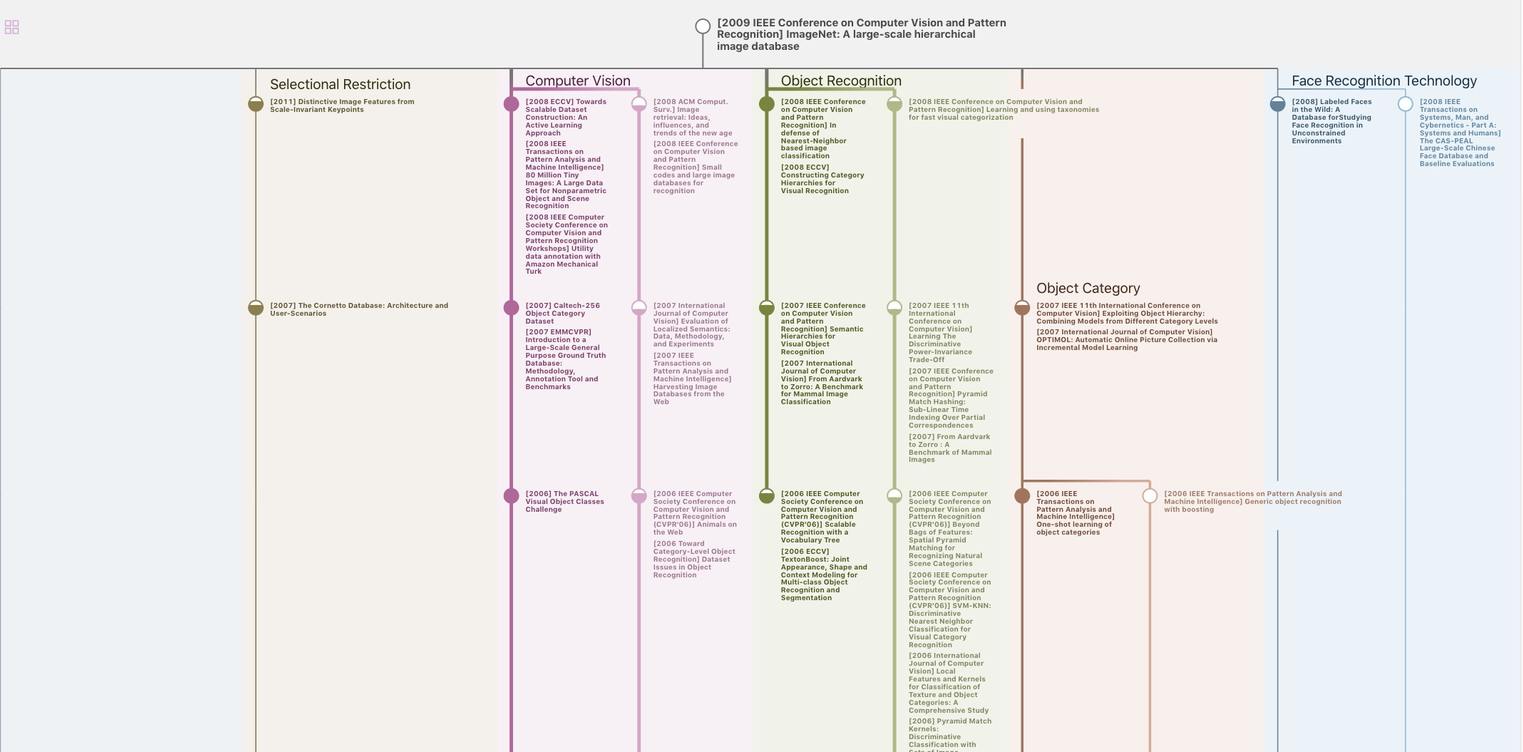
生成溯源树,研究论文发展脉络
Chat Paper
正在生成论文摘要