Iteratively Learning Novel Strategies with Diversity Measured in State Distances
ICLR 2023(2023)
摘要
In complex reinforcement learning (RL) problems, policies with similar rewards may have substantially different behaviors. Yet, to not only optimize rewards but also discover as many diverse strategies as possible remains a challenging problem. A natural approach to this task is constrained population-based training (PBT), which simultaneously learns a collection of policies subject to diversity constraints. However, due to the unaffordable computation cost of PBT, we adopt an alternative approach, iterative learning (IL), which repeatedly learns a single novel policy that is sufficiently different from previous ones. We first analyze these two frameworks and prove that, for any policy pool derived by PBT, we can always use IL to obtain another policy pool of the same rewards and competitive diversity scores. In addition, we also present a novel state-based diversity measure with two tractable realizations. Such a metric can impose a stronger and much smoother diversity constraint than existing action-based metrics. Combining IL and the state-based diversity measure, we develop a powerful diversity-driven RL algorithm, State-based Intrinsic-reward Policy Optimization (SIPO), with provable convergence properties. We empirically examine our algorithm in complex multi-agent environments including StarCraft Multi-Agent Challenge and Google Research Football. SIPO is able to consistently derive strategically diverse and human-interpretable policies that cannot be discovered by existing baselines.
更多查看译文
关键词
diverse behavior,multi-agent reinforcement learning,deep reinforcement learning
AI 理解论文
溯源树
样例
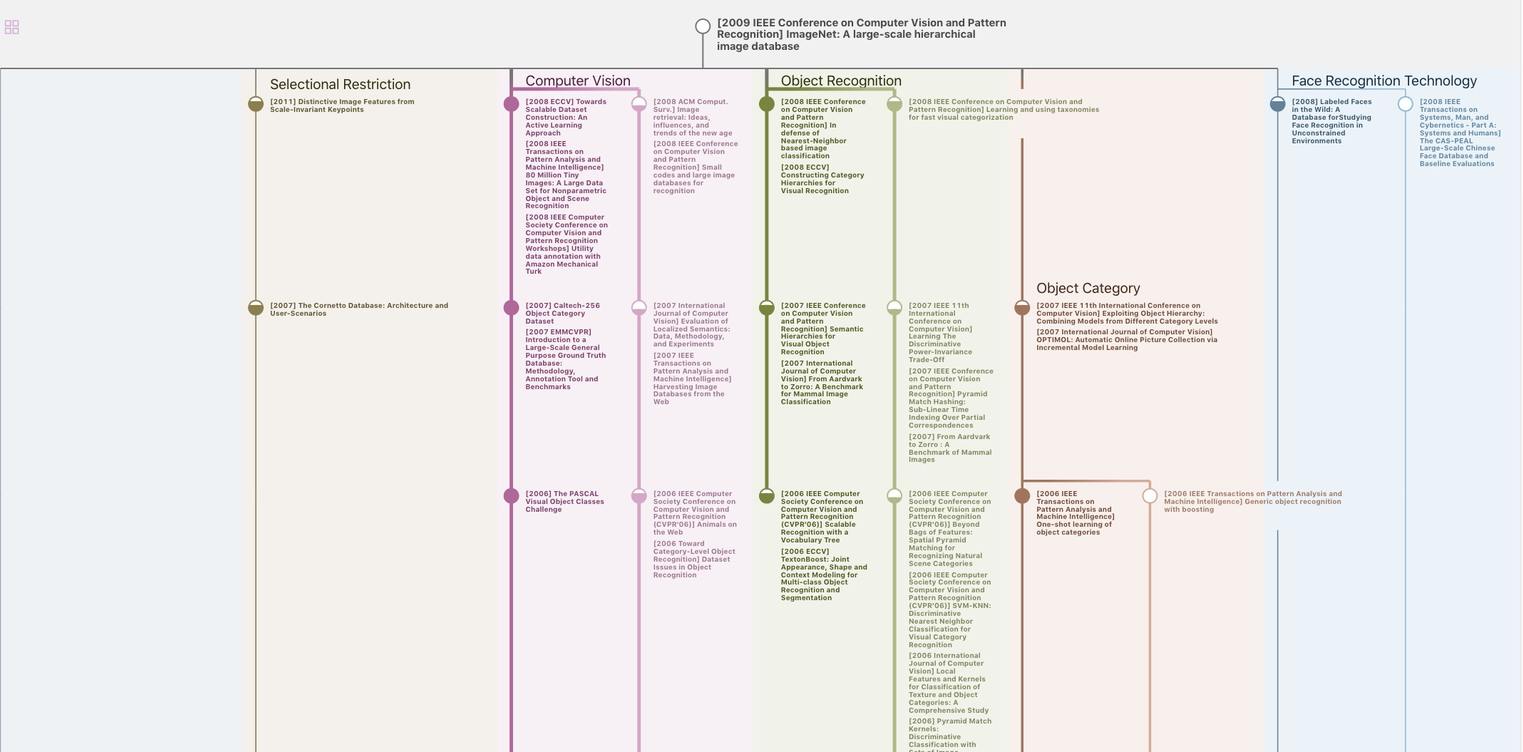
生成溯源树,研究论文发展脉络
Chat Paper
正在生成论文摘要