VEHICLE-INFRASTRUCTURE COOPERATIVE 3D DETECTION VIA FEATURE FLOW PREDICTION
ICLR 2023(2023)
摘要
Effectively utilizing data from infrastructure could greatly improve autonomous driving safety. Vehicle-Infrastructure Cooperative 3D Object Detection (VIC3D) is an important task to localize and recognize objects surrounding the ego-vehicle by combining the sensor data from both ego-vehicle and roadside infrastructure. However, there are serious temporal asynchrony problems between vehicle and infrastructure data. To the best of our knowledge, no existing work in the literature could effectively solve the asynchrony problem with limited communication bandwidth and computational resources on vehicle-infrastructure devices. This work proposes a novel approach for VIC3D, called Feature Flow Network(FFNet), to effectively address the problem of temporal asynchrony caused by different sensor initialization and latency. Compared with previous feature fusion approaches that only use the current static feature, FFNet transmits the feature flow and generates the future features on-the-fly, aligned with the ego-vehicle timestamp. We propose a self-supervised method to train the feature flow generation model, and use the pre-trained infrastructure model to extract features from randomly assigned future frames as ground truth. Extensive experiments on the DAIR-V2X dataset (a large-scale real-world V2X dataset) show that FFNet establishes a new state of the art, surpassing SOTA methods by up to 5% mAP while with comparable transmission cost. In particularly, FFNet can even make up for almost all the performance drop caused by the temporal asynchrony in 200ms delay.
更多查看译文
AI 理解论文
溯源树
样例
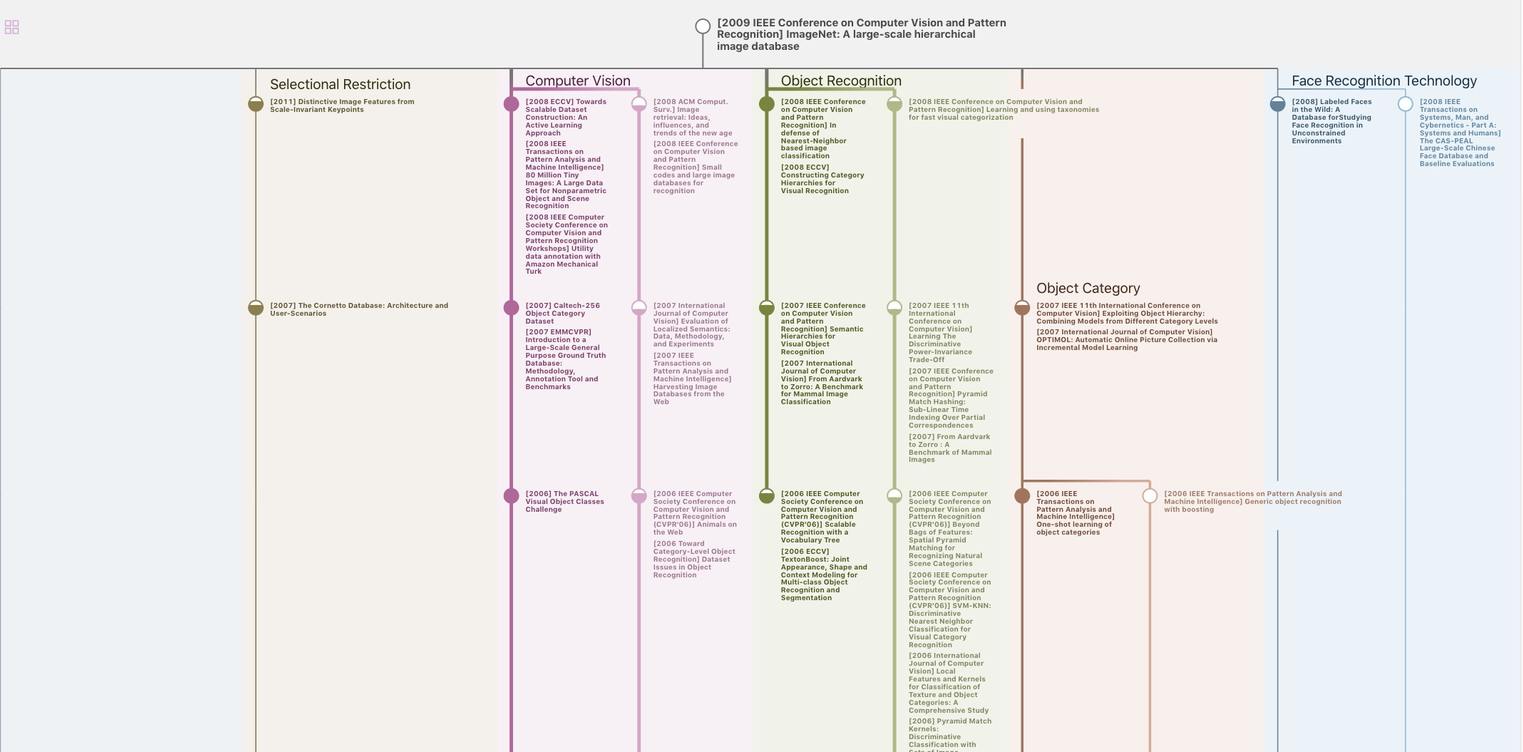
生成溯源树,研究论文发展脉络
Chat Paper
正在生成论文摘要