Improving the Estimation of Instance-dependent Transition Matrix by using Self-supervised Learning
ICLR 2023(2023)
摘要
The transition matrix reveals the transition relationship between clean labels and noisy labels. It plays an important role in building statistically consistent classifiers. In real-world applications, the transition matrix is usually unknown and has to be estimated. It is a challenging task to accurately estimate the transition matrix, especially when it depends on the instance. Given that both instances and noisy labels are available, the major difficulty of learning the transition matrix comes from the missing of clean information. A lot of methods have been proposed to infer clean information. The self-supervised learning has demonstrated great success. These methods could even achieve comparable performance with supervised learning on some datasets but without requiring any labels during the training. It implies that these methods can efficiently infer clean labels.
Motivated by this, in this paper, we have proposed a practical method that leverages self-supervised learning to help learn the instance-dependent transition matrix. Empirically, the proposed method has achieved state-of-the-art performance on different datasets.
更多查看译文
AI 理解论文
溯源树
样例
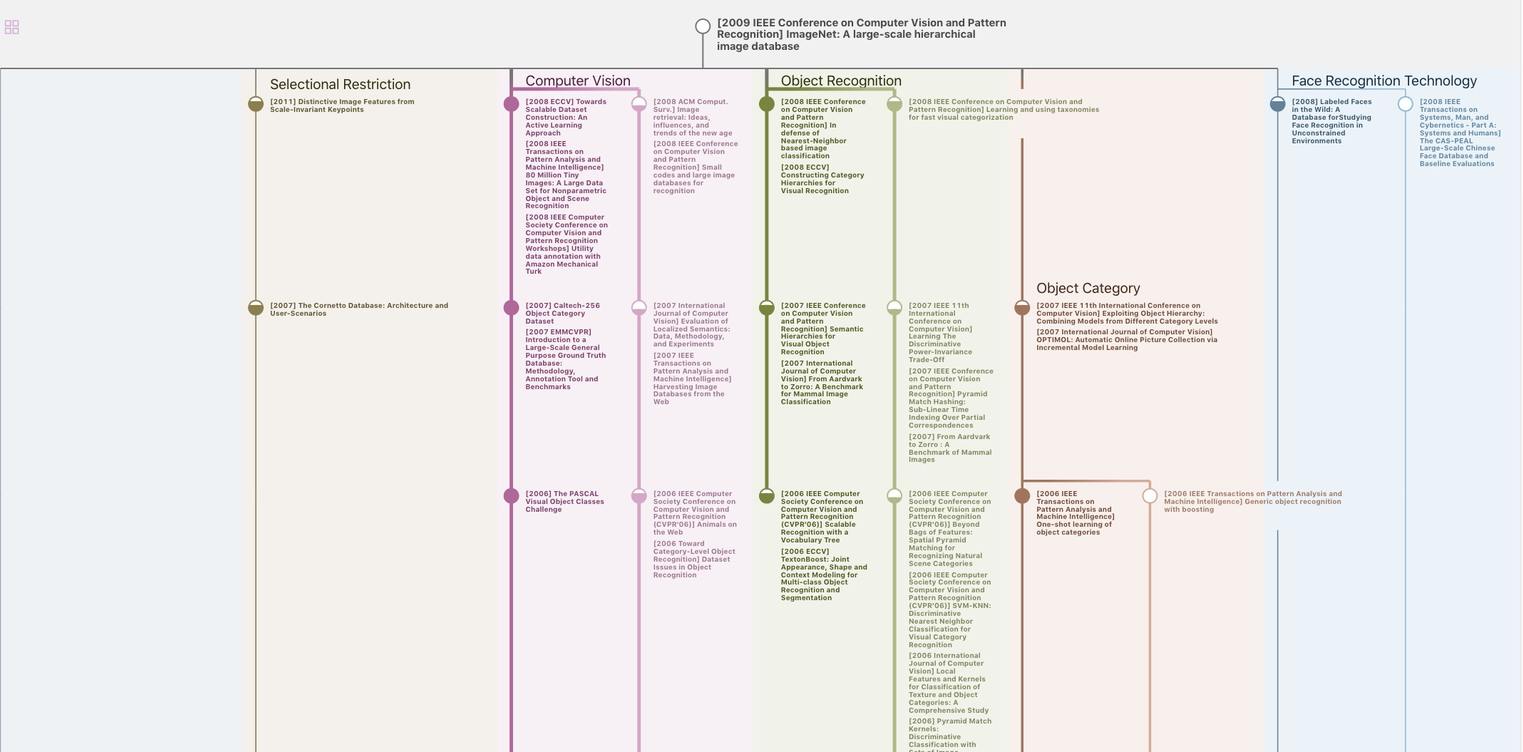
生成溯源树,研究论文发展脉络
Chat Paper
正在生成论文摘要