Self-Supervised Logit Adjustment
ICLR 2023(2023)
摘要
Self-supervised learning (SSL) has achieved tremendous success on various well curated datasets in computer vision and natural language processing. Nevertheless, it is hard for existing works to capture transferable and robust features, when facing the long-tailed distribution in the real-world scenarios. The attribution is that plain SSL methods to pursue sample-level uniformity easily leads to the distorted embedding space, where head classes with the huge sample number dominate the feature regime and tail classes passively collapse. To tackle this problem, we propose a novel Self-Supervised Logit Adjustment ($S^2LA$) method to achieve the category-level uniformity from a geometric perspective. Specially, we measure the geometric statistics of the embedding space to construct the calibration, and jointly learn a surrogate label allocation to constrain the space expansion of head classes and avoid the passive collapse of tail classes. Our proposal does not alter the setting of SSL and can be easily integrated into existing works in an end-to-end and low-cost manner. Extensive results on a range of benchmark datasets show the effectiveness of $S^2LA$ with high tolerance to the distribution skewness.
更多查看译文
关键词
Long-tailed learning,self-supervised learning,logit adjustment,optimal transport
AI 理解论文
溯源树
样例
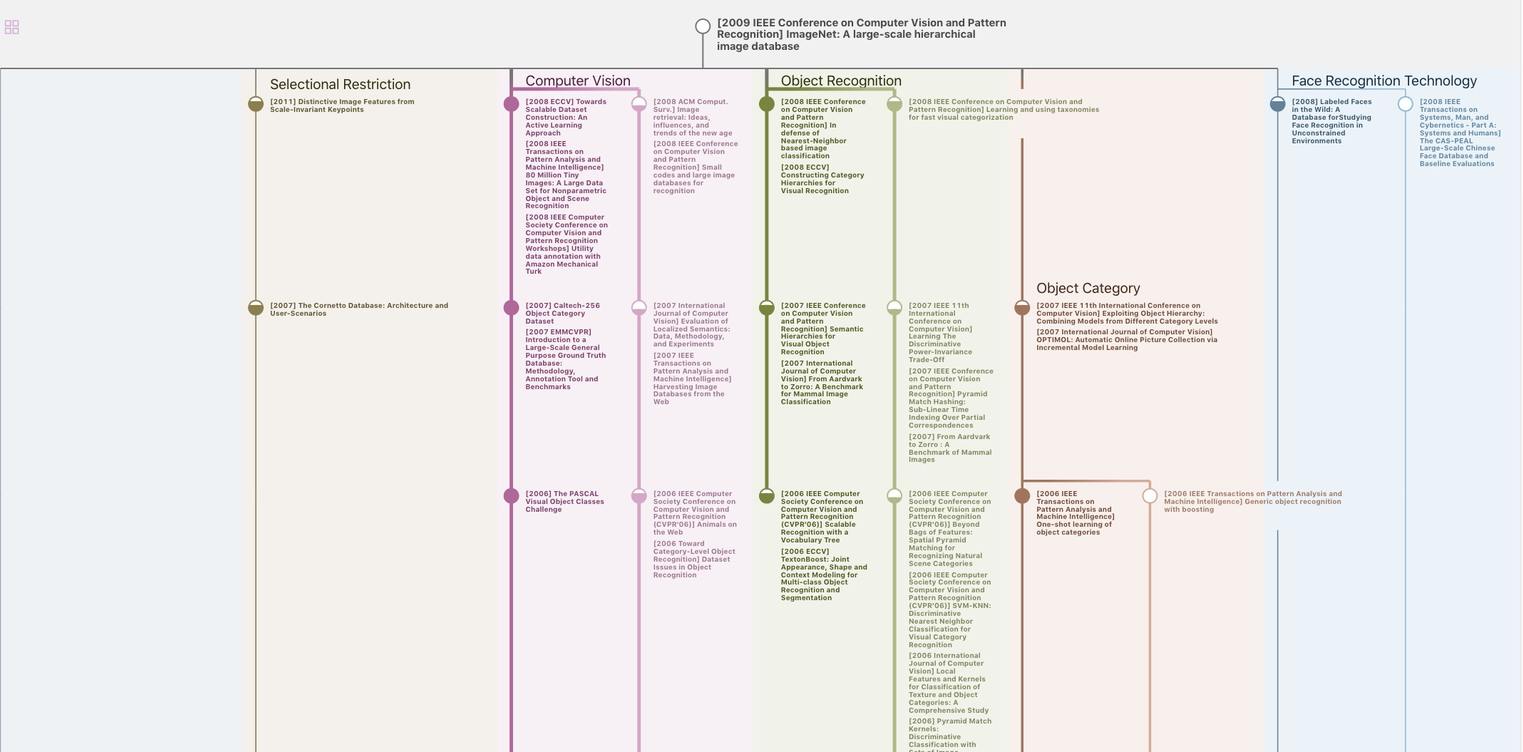
生成溯源树,研究论文发展脉络
Chat Paper
正在生成论文摘要