Poisoning Generative Models to Promote Catastrophic Forgetting
ICLR 2023(2023)
摘要
Generative models have grown into the workhorse of many state-of-the-art machine learning methods. However, their vulnerability under poisoning attacks has been largely understudied. In this work, we investigate this issue in the context of continual learning, where generative replayers are utilized to tackle catastrophic forgetting. By developing a novel customization of dirty-label input-aware backdoor to the online setting, our attacker manages to stealthily promote forgetting while retaining high accuracy at the current task and sustaining strong defenders. Our approach taps into an intriguing property of generative models, namely that they cannot well capture input-dependent triggers. Experiments on four standard datasets corroborate the poisoner’s effectiveness.
更多查看译文
关键词
poisoning attacks,backdoor attacks
AI 理解论文
溯源树
样例
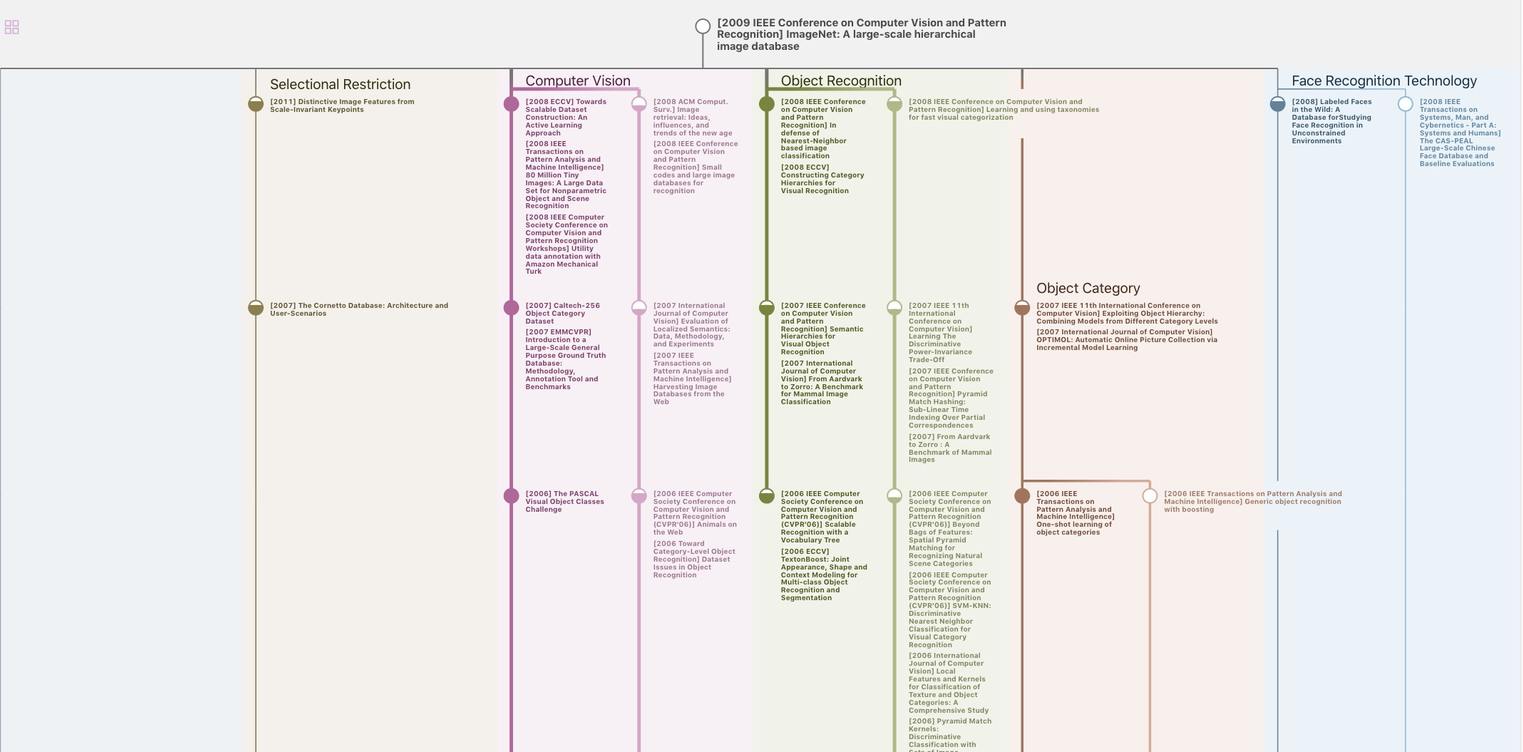
生成溯源树,研究论文发展脉络
Chat Paper
正在生成论文摘要