VQ-TR: Vector Quantized Attention for Time Series Forecasting
ICLR 2023(2023)
摘要
Modern time series datasets can easily contain hundreds or thousands of temporal time points, however, Transformer based models scale poorly to the size of the sequence length constraining their context size in the seq-to-seq setting. In this work, we introduce VQ-TR which maps large sequences to a discrete set of latents representations as part of the Attention module. This allows us to attend over larger context windows with linear complexity with respect to the sequence length. We compare this method with other competitive deep learning and classical univariate probabilistic models and highlight its performance using both probabilistic and point forecasting metrics on a variety of open datasets from different domains.
更多查看译文
关键词
deep learning,time series forecasting,latent variable models,transformer
AI 理解论文
溯源树
样例
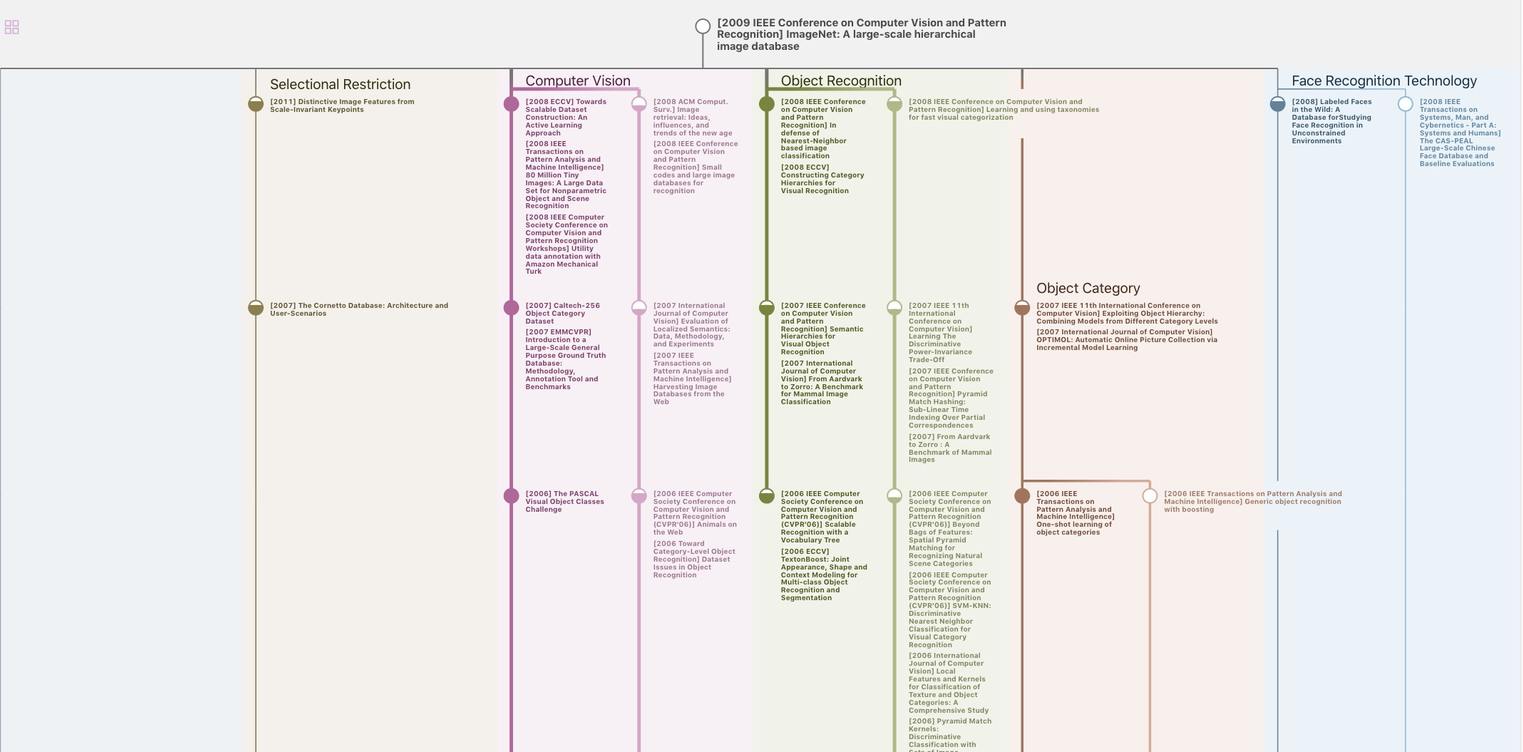
生成溯源树,研究论文发展脉络
Chat Paper
正在生成论文摘要