Diversity of Generated Unlabeled Data Matters for Few-shot Hypothesis Adaptation
ICLR 2023(2023)
摘要
Generating unlabeled data has been recently shown to help address the few-shot hypothesis adaptation (FHA) problem, where we aim to train a classifier for the target domain with a few labeled target-domain data and a well-trained source-domain classifier (i.e., a source hypothesis), for the additional information of the highly-compatible unlabeled data. However, the generated data of the existing methods are extremely similar or even the same. The strong dependency among the generated data will lead the learning to fail. In this paper, we propose a diversity-enhancing generative network (DEG-Net) for the FHA problem, which can generate diverse unlabeled data with the help of a kernel independence measure: the Hilbert-Schmidt independence criterion (HSIC). Specifically, DEG-Net will generate data via minimizing the HSIC value (i.e., maximizing the independence) among the semantic features of the generated data. By DEG-Net, the generated unlabeled data are more diverse and more effective for addressing the FHA problem. Experimental results show that the DEG-Net outperforms existing FHA baselines and further verifies that generating diverse data plays an important role in addressing the FHA problem.
更多查看译文
关键词
Hypothesis Adaptation
AI 理解论文
溯源树
样例
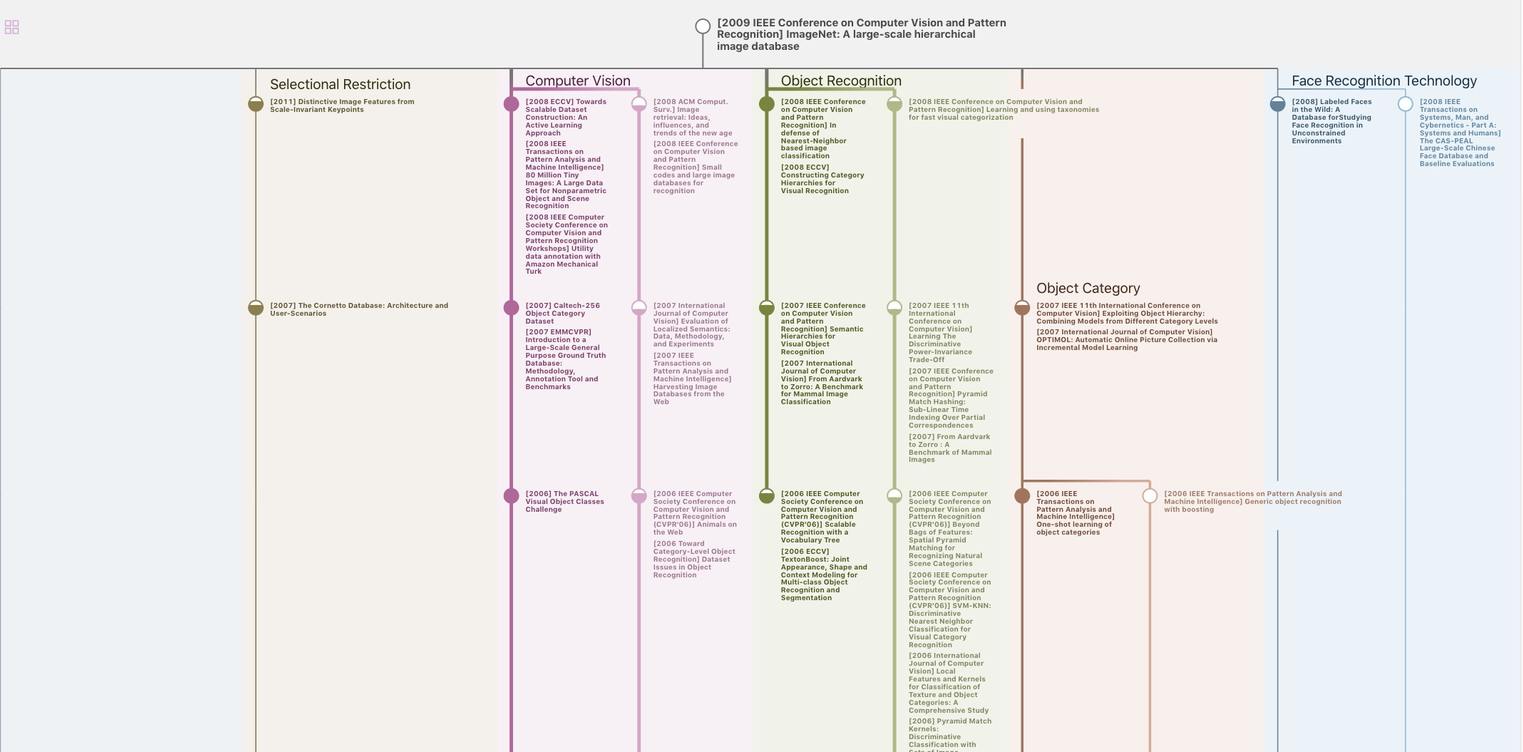
生成溯源树,研究论文发展脉络
Chat Paper
正在生成论文摘要