Multi-Head State Space Model for Sequence Modeling
ICLR 2023(2023)
摘要
Recently, state space models (SSMs) have shown promising results on sequence modeling tasks. However, a potential challenge of existing works is that SSMs are usually introduced or initialized in a homogeneous way, encouraging the model to only capture similar temporal dynamics on different features. In this paper, we propose a multi-head state space model (MSSM), in which parallel heads are introduced to learn different temporal dynamics on sequence data. Furthermore, we propose a novel variant of the Transformer, referred to as the Stateformer, which combines MSSMs with attention. Experiments on large-scale automatic speech recognition (ASR) and language modeling tasks show the MSSM outperforming a range of attention-based baselines. The Stateformer further improves performance, achieving the state-of-the-art performance on the LibriSpeech ASR task.
更多查看译文
关键词
multi-head,state space,transformer,stateformer,sequence model,rnn
AI 理解论文
溯源树
样例
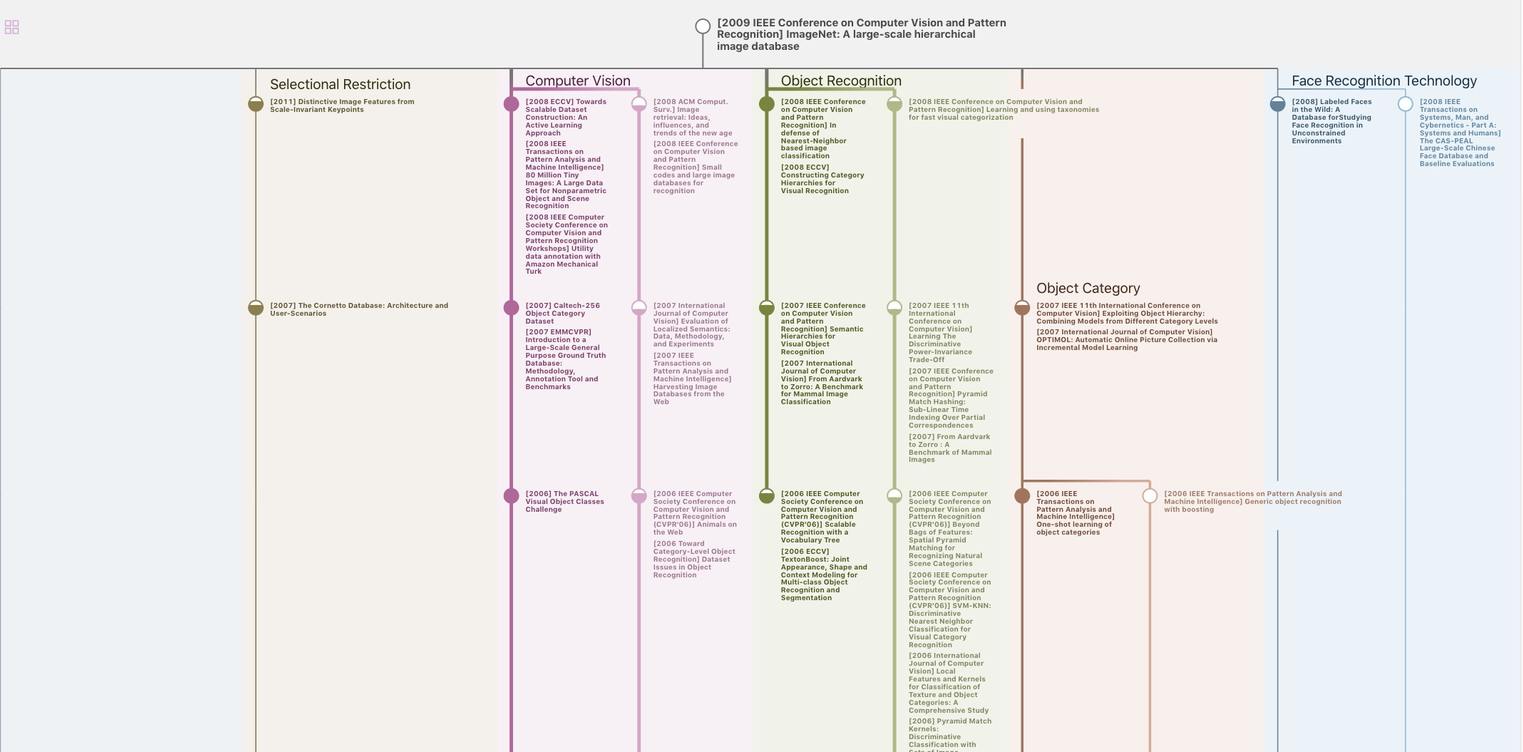
生成溯源树,研究论文发展脉络
Chat Paper
正在生成论文摘要