Lightweight Equivariant Graph Representation Learning for Protein Engineering
ICLR 2023(2023)
摘要
This work tackles the issue of directed evolution in computational protein design that makes accurate predictions of the function of a protein mutant. We design a lightweight pre-training graph neural network model for multi-task protein representation learning from its 3D structure. Rather than reconstructing and optimizing the protein structure, the trained model recovers the amino acid types and key properties of the central residues from a given noisy three-dimensional local environment. On the prediction task for the higher-order mutants, where many amino acid sites of the protein are mutated, the proposed training strategy achieves remarkably higher performance by 20% improvement at the cost of requiring less than 1% of computational resources that are required by popular transformer-based state-of-the-art deep learning models for protein design.
更多查看译文
关键词
graph neural networks
AI 理解论文
溯源树
样例
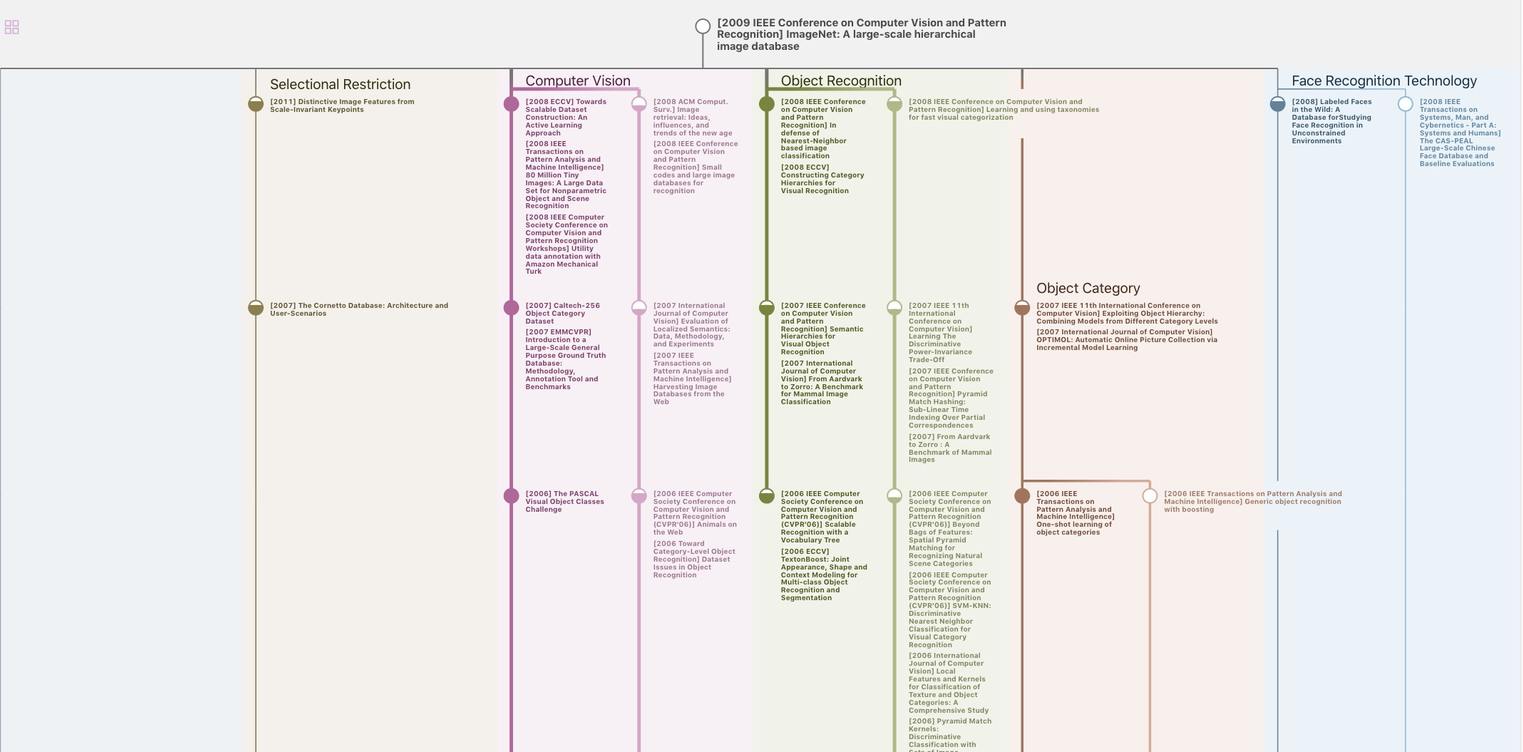
生成溯源树,研究论文发展脉络
Chat Paper
正在生成论文摘要