Hidden Markov Mixture of Gaussian Process Functional Regression: Utilizing Multi-Scale Structure for Time Series Forecasting
ICLR 2023(2023)
摘要
The mixture of Gaussian process functional regressions (GPFRs) assumes that there is a batch of time series or sample curves that are generated by independent random processes with different temporal structures. However, in real situations, these structures are actually transferred in a random manner from a long time scale. Therefore, the assumption of independent curves is not true in practice. In order to get rid of this limitation, we propose the hidden-Markov-based GPFR mixture model (HM-GPFR) by describing these curves with both fine- and coarse-level temporal structures. Specifically, the temporal structure is described by the Gaussian process model at the fine level and the hidden Markov process at the coarse level. The whole model can be regarded as a random process with state switching dynamics. To further enhance the robustness of the model, we also give a priori parameters to the model and develop a Bayesian-hidden-Markov-based GPFR mixture model (BHM-GPFR). The experimental results demonstrated that the proposed methods have both high prediction accuracy and good interpretability.
更多查看译文
关键词
Gaussian processes,functional data analysis,time series forecasting,hidden Markov model,EM algorithm
AI 理解论文
溯源树
样例
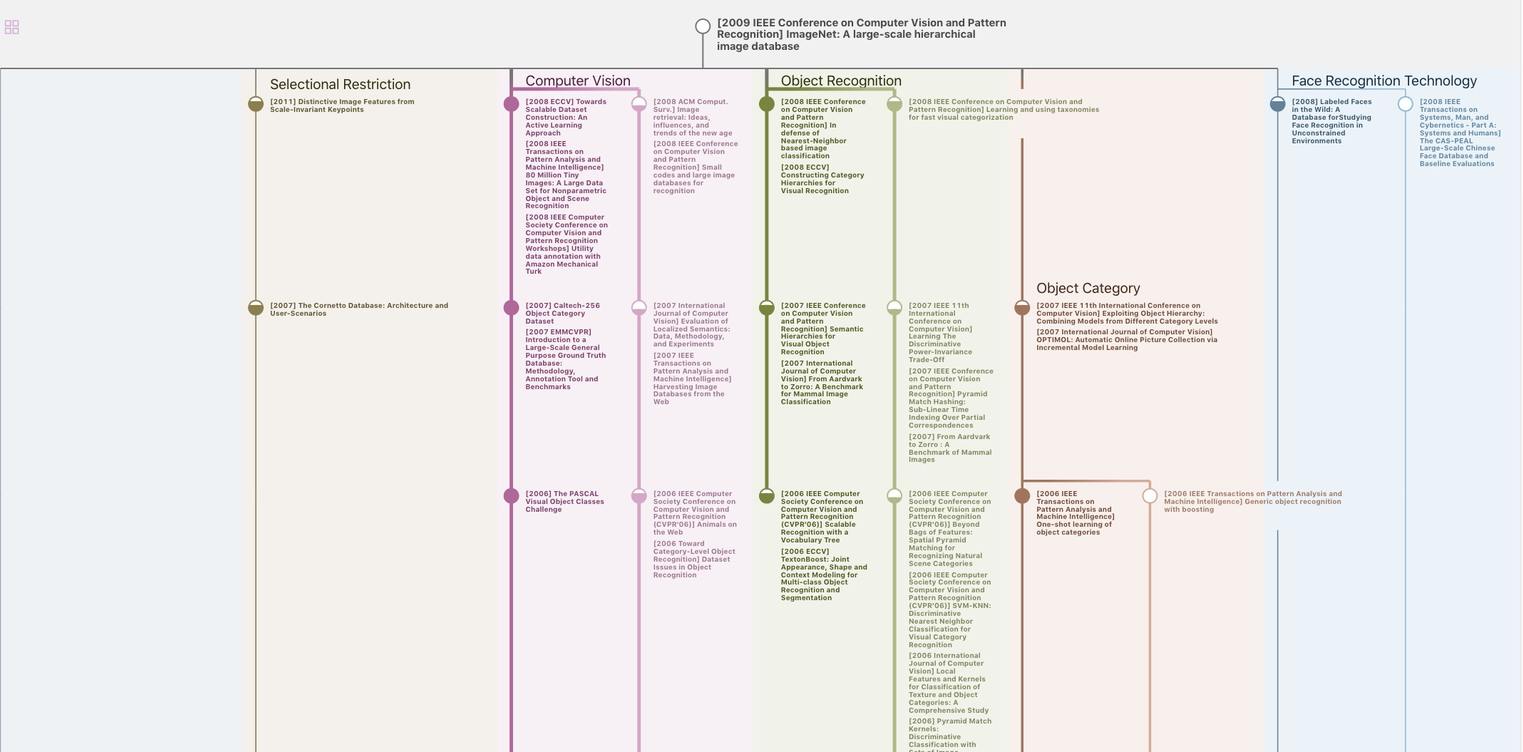
生成溯源树,研究论文发展脉络
Chat Paper
正在生成论文摘要