Tackling Imbalanced Class in Federated Learning via Class Distribution Estimation
ICLR 2023(2023)
摘要
Federated Learning (FL) has become an upsurging machine learning method due to its applicability in large-scale distributed system and its privacy-preserving property. However, in real-world applications, the presence of class imbalance issue, especially the mismatch between local and global class distribution, greatly degrades the performance of FL. Moreover, due to the privacy constrain, the class distribution information of clients can not be accessed directly. To tackle class imbalance issue under FL setting, a novel algorithm, FedRE, is proposed in this paper. We propose a new class distribution estimation method for the FedRE algorithm, which requires no extra client data information and thus has no privacy concern. Both experimental results and theoretical analysis are provided to support the validity of our distribution estimation method. The proposed algorithm is verified with several experiment, including different datasets with the presence of class imbalance and local-global distribution mismatch. The experimental results show that FedRE is effective and it outperforms other related methods in terms of both overall and minority class classification accuracy.
更多查看译文
关键词
Federated Learning,class imbalance,class distribution estimation
AI 理解论文
溯源树
样例
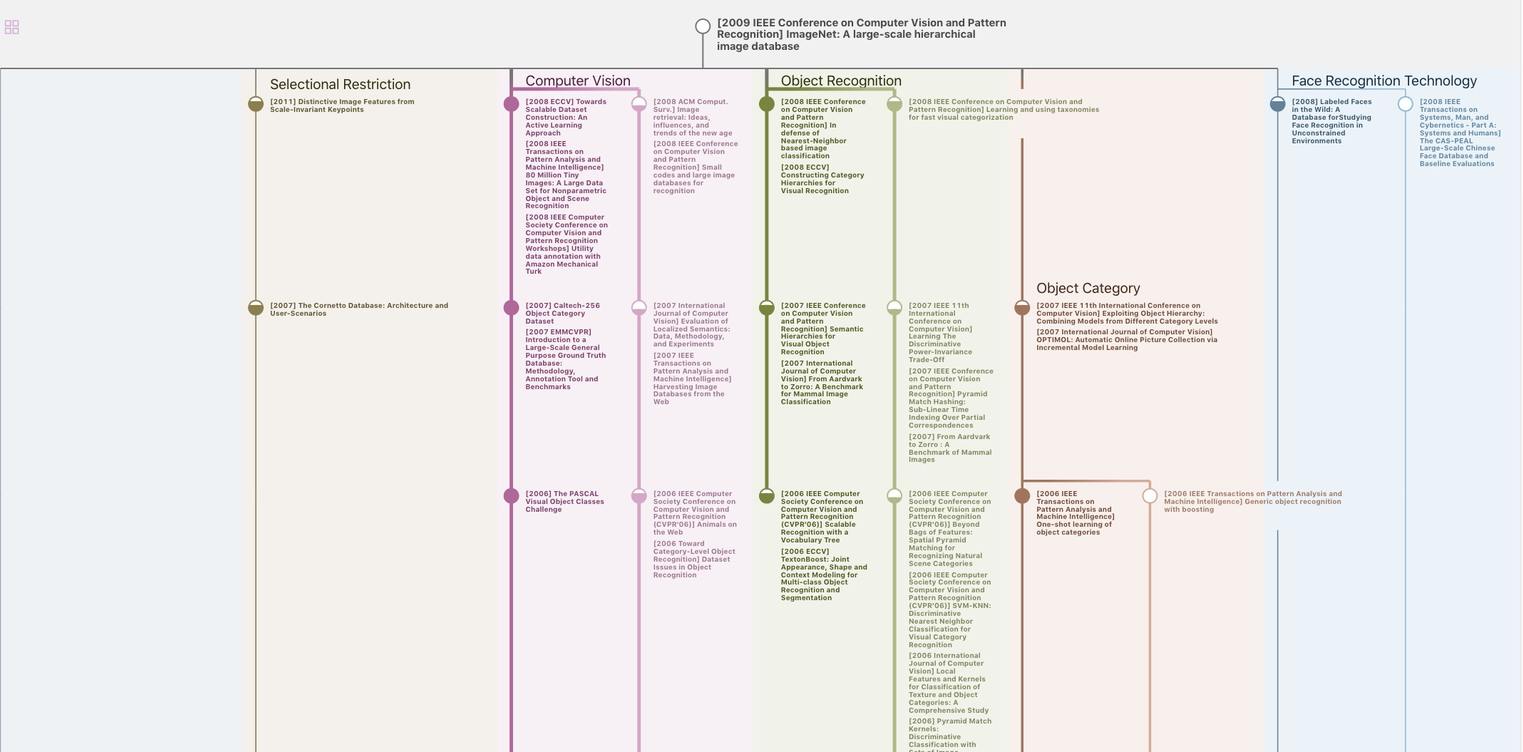
生成溯源树,研究论文发展脉络
Chat Paper
正在生成论文摘要