Would decentralization hurt generalization?
ICLR 2023(2023)
摘要
Decentralized stochastic gradient descent (D-SGD) allows collaborative learning on massive devices without controlling of a central server. Existing theory suggests that the decentralization degrades the generalizability, which conflicts with experimental results in the large-batch settings that D-SGD generalize better than centralized SGD (C-SGD). This work presents new theory that reconciles the conflict between the two perspectives. We prove that D-SGD introduces an implicit regularization that simultaneously penalizes (1) the sharpness of the learned minima and (2) the consensus distance between the consensus model and local models. We then prove that the implicit regularization is amplified in the large-batch settings when the linear scaling rule is applied. We further analyze the escaping efficiency of D-SGD, which suggests that D-SGD favors super-quadratic flat minima. Experiments are in full agreement with our theory. The code will be released publicly. To our best knowledge, this is the first work on the implicit regularization and escaping efficiency of D-SGD.
更多查看译文
关键词
decentralized SGD,flat minima,generalization,implicit regularization,large batch training
AI 理解论文
溯源树
样例
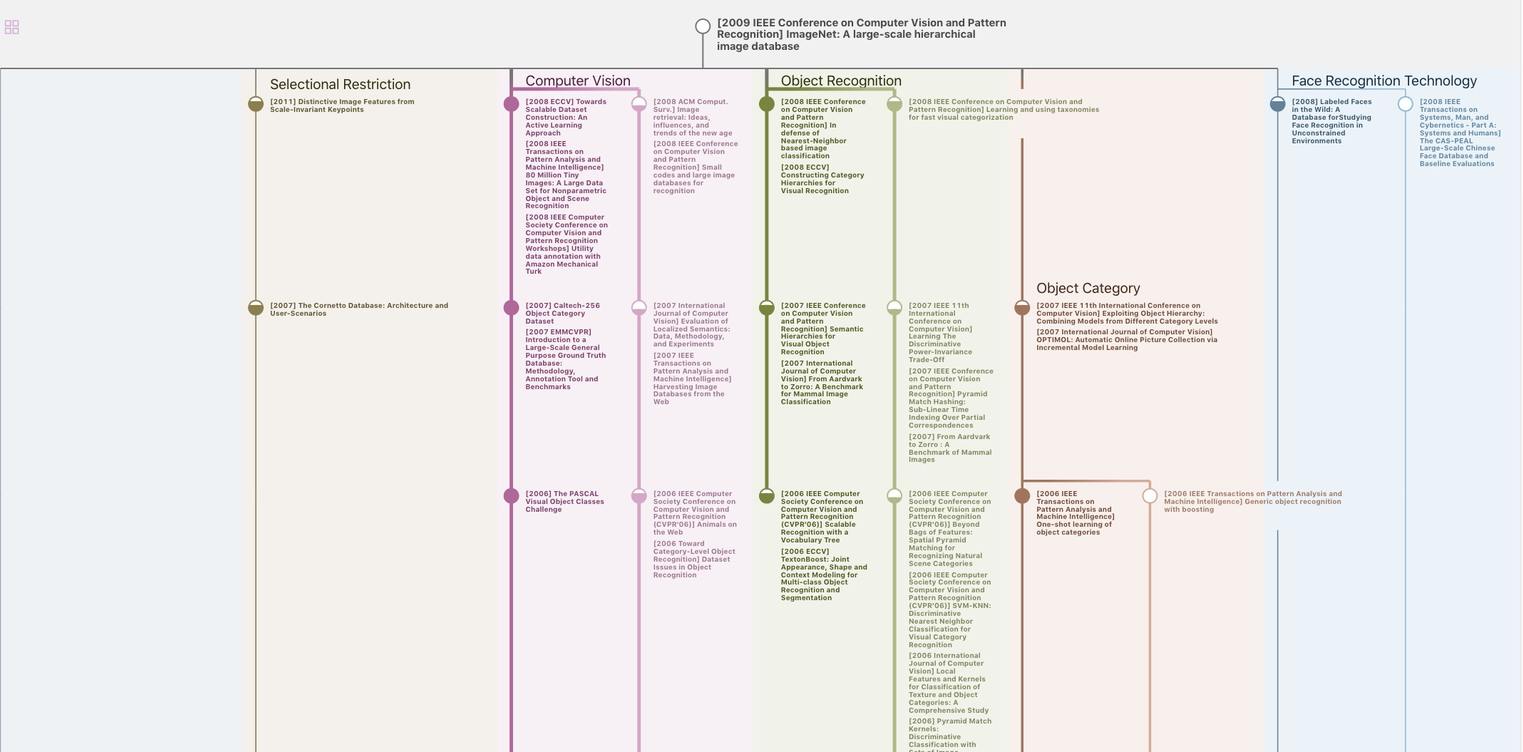
生成溯源树,研究论文发展脉络
Chat Paper
正在生成论文摘要