Robust Exploration via Clustering-based Online Density Estimation
ICLR 2023(2023)
摘要
Intrinsic motivation is a critical ingredient in reinforcement learning to enable progress when rewards are sparse. However, many existing approaches that measure the novelty of observations are brittle, or rely on restrictive assumptions about the environment which limit generality. We propose to decompose the exploration problem into two orthogonal sub-problems: (i) finding the right representation (metric) for exploration (ii) estimating densities in this representation space.
To address (ii), we introduce Robust Exploration via Clustering-based Online Density Estimation (RECODE), a non-parametric method that estimates visitation counts for clusters of states that are similar according to the metric induced by any arbitrary representation learning technique. We adapt classical clustering algorithms to design a new type of memory that allows RECODE to keep track of the history of interactions over thousands of episodes, thus effectively tracking global visitation counts. This is in contrast to existing non-parametric approaches, that can only store the recent history, typically the current episode.
The generality of RECODE allows us to easily address (i) by leveraging both off-the-shelf and novel representation learning techniques. In particular, we introduce a novel generalization of the action-prediction representation that leverages multi-step predictions and that we find to be better suited to a suite of challenging 3D-exploration tasks in DM-HARD-8. We show experimentally that our approach can work with a variety of RL agents, and obtain state-of-the-art performance on Atari and DM-HARD-8.
更多查看译文
关键词
exploration,representation learning,density estimation,reinforcement learning
AI 理解论文
溯源树
样例
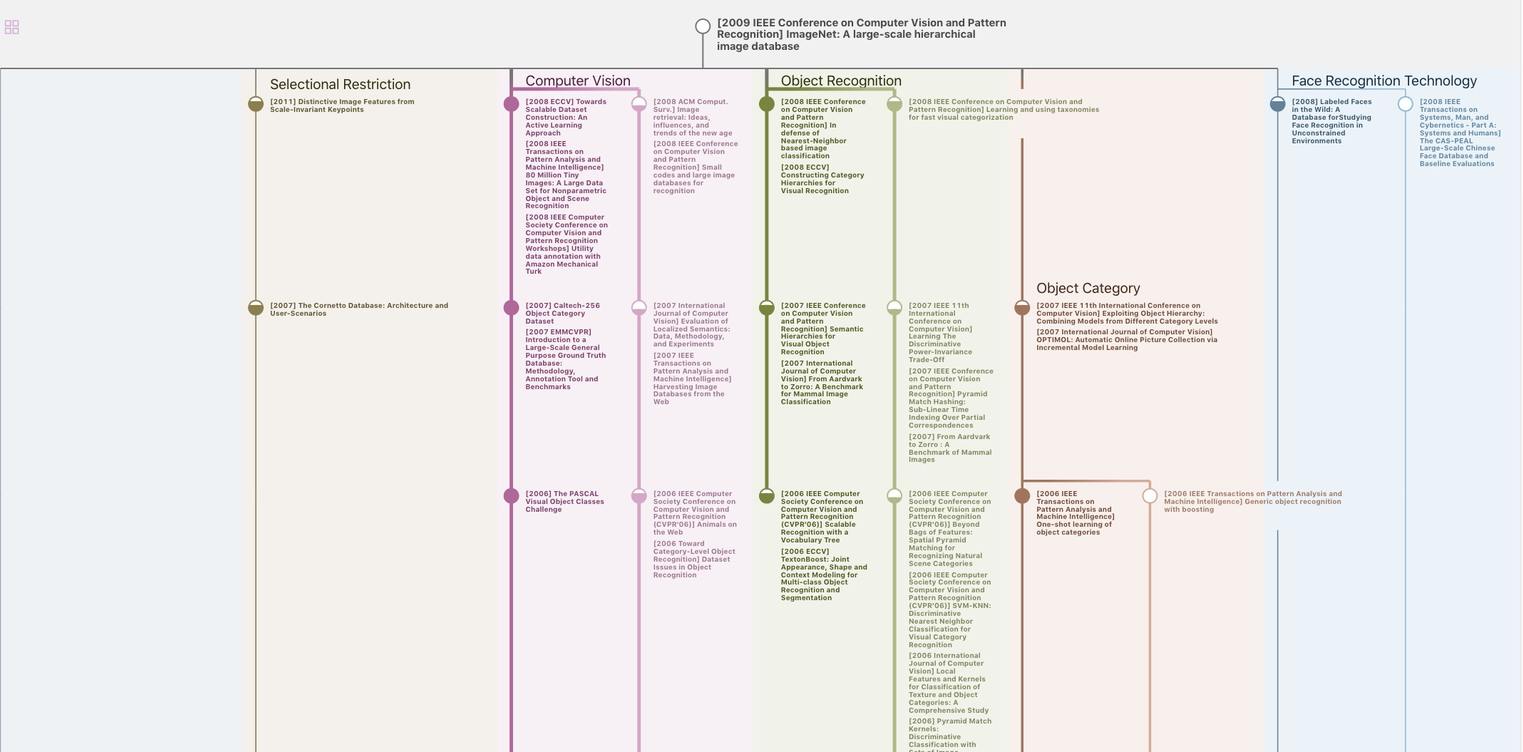
生成溯源树,研究论文发展脉络
Chat Paper
正在生成论文摘要