Unsupervised Non-Parametric Signal Separation Using Bayesian Neural Networks
ICLR 2023(2023)
摘要
Bayesian neural networks (BNN) take the best from two worlds: the one of flexible and scalable neural networks and the one of probabilistic graphical models, the latter allowing for probabilistic interpretation of inference results.
We make one extra step towards unification of these two domains and render BNN as an elementary unit of abstraction in the framework of probabilistic modeling, which allows us to promote well-known distributions to distribution fields.
We use transformations to obtain field versions of several popular distributions and demonstrate the utility of our approach on the problem of signal/background separation.
Starting from prior knowledge that a certain region of space contains predominantly one of the components, in an unsupervised and non-parametric manner, we recover the representation of both previously unseen components as well as their proportions.
更多查看译文
关键词
bayesian neural networks,probabilistic graphical models,signal disaggregation
AI 理解论文
溯源树
样例
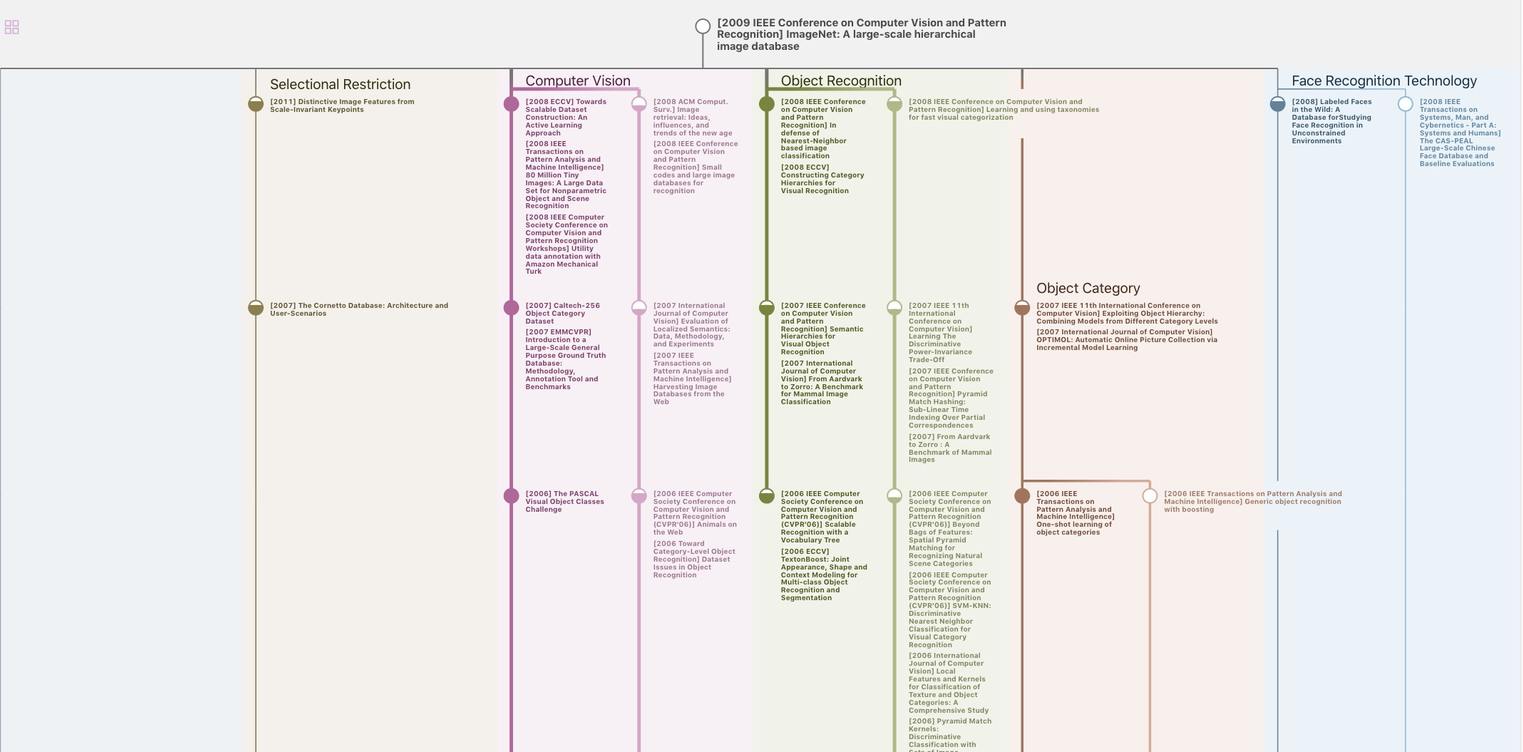
生成溯源树,研究论文发展脉络
Chat Paper
正在生成论文摘要