A Simulation-based Framework for Robust Federated Learning to Training-time Attacks
ICLR 2023(2023)
摘要
Well-known robust aggregation schemes in federated learning (FL) are shown to be vulnerable to an informed adversary who can tailor training-time attacks [Fang et al., Xie et al.]. We frame robust distributed learning problem as a game between a server and an adversary that is able to optimize strong training-time attacks. We introduce RobustTailor, a simulation-based framework that prevents the adversary from being omniscient. The simulated game we propose enjoys theoretical guarantees through a regret analysis. RobustTailor improves robustness to training-time attacks significantly while preserving almost the same privacy guarantees as standard robust aggregation schemes in FL. Empirical results under challenging attacks show that RobustTailor performs similar to an upper bound with perfect knowledge of honest clients.
更多查看译文
关键词
Robust federated learning,training-time attacks,game theory
AI 理解论文
溯源树
样例
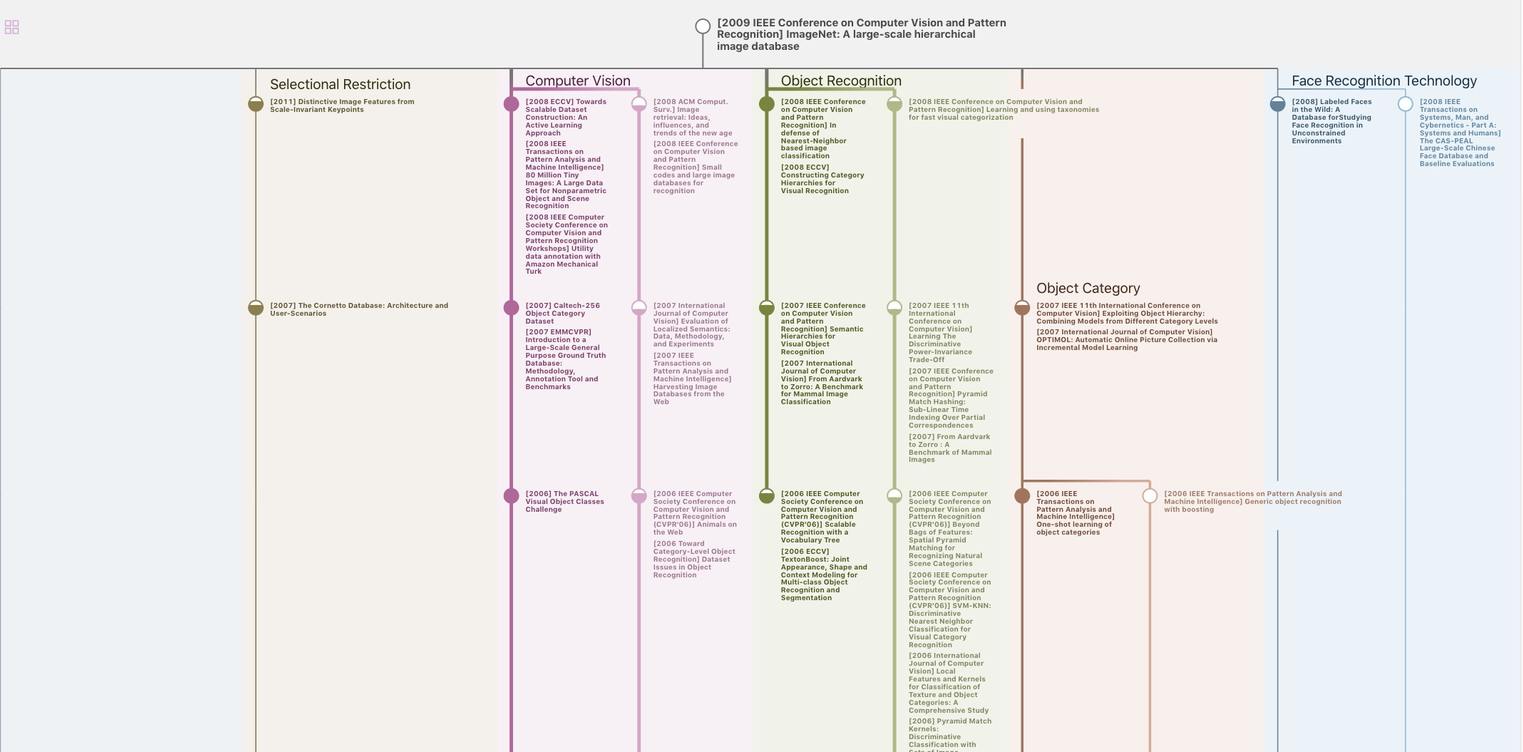
生成溯源树,研究论文发展脉络
Chat Paper
正在生成论文摘要