Unleash Model Capacity for Universal Dense Retrieval by Task Specialty Optimization
ICLR 2023(2023)
摘要
Universal dense retrieval, with one unified representation space to empower various retrieval scenarios, has many appealing advantages in simplicity, efficiency, and potential to break echo chambers with cross-scenario information access. However, standard multi-task trained dense retrievers often fail to meet the accuracy of scenario-specific models. In this paper, we analyze the multi-task learning in universal retrieval and show that the model capacity is not the main bottleneck. It is the optimization failed to fully utilize the network parameters to capture task-specific signals. This motivated our development of TACO-DR, which conducts multi-task learning for universal retrieval with TAsk speCialty Optimization. TACO-DR dynamically adjusts the learning rate for each parameter regrading each task based on its task-specific sensitivity, to encourage parameters to better capture task specific signals. On the KILT benchmark, TACO-DR outperforms various multi-task learning methods and achieves better overall accuracy than single-task models. Our analysis shows that TACO-DR better utilizes the model capacity with more task-specific parameters. Our code and model checkpoints will be open-sourced.
更多查看译文
关键词
Dense Retrieval,Multi-task,Parameter sensitivity
AI 理解论文
溯源树
样例
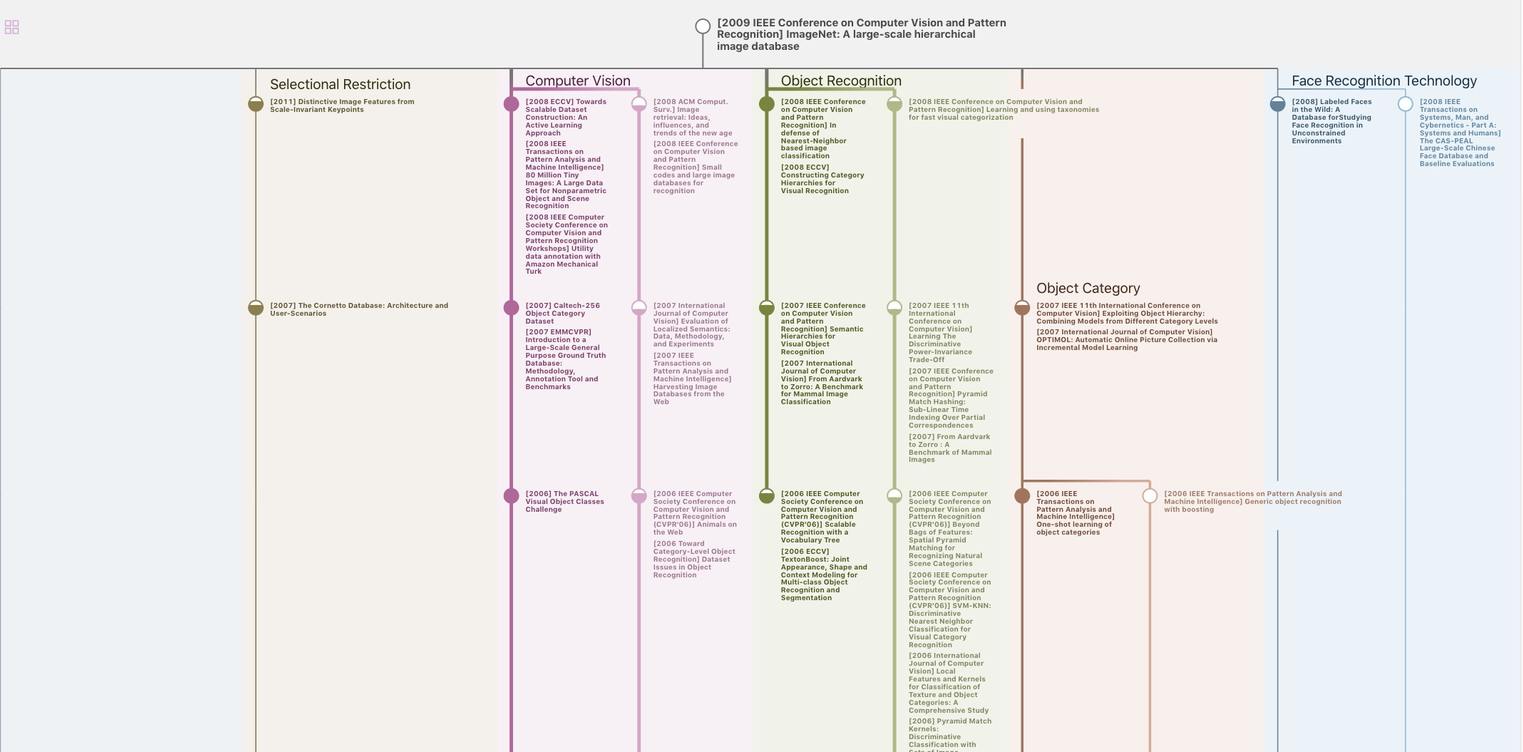
生成溯源树,研究论文发展脉络
Chat Paper
正在生成论文摘要