Progressive Data Dropout: An Adaptive Training Strategy for Large-Scale Supervised Learning
ICLR 2023(2023)
摘要
Common training strategies for deep neural networks are computationally expensive, continuing to redundantly train and evaluate on classes already well-understood by the model. A common strategy to diminish this cost is to reduce data used in training, however this often comes at the expense of the model's accuracy or an additional computational cost in training. We propose progressive data dropout (PDD), an adaptive training strategy which performs class-level data dropout from the training set as the network develops an understanding for each class. Our experiments on large-scale image classification demonstrate PDD reduces the total number of datapoints needed to train the network by a factor of 10, reducing the overall training time without significantly impacting accuracy or modifying the model architecture. We additionally demonstrate improvements via experiments and ablations on computer vision benchmarks, including MNIST, Fashion-MNIST, SVHN, CIFAR, and ImageNet datasets.
更多查看译文
关键词
data dropout,training optimization,adaptive training,classification,large-scale
AI 理解论文
溯源树
样例
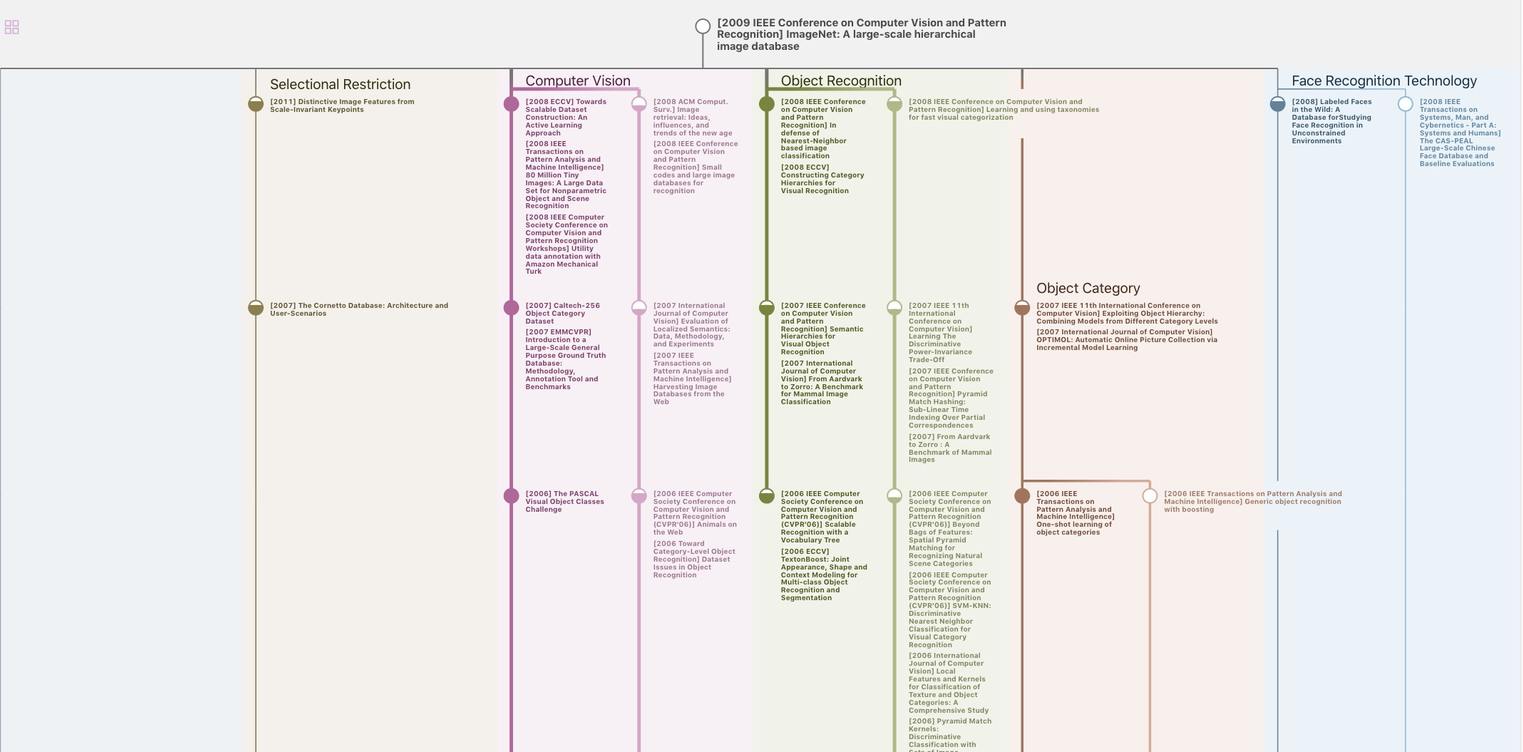
生成溯源树,研究论文发展脉络
Chat Paper
正在生成论文摘要