Homotopy Learning of Parametric Solutions to Constrained Optimization Problems
ICLR 2023(2023)
摘要
Building deep learning (DL) alternatives to constrained optimization problems has been proposed as a cheaper solution approach than classical constrained optimization solvers. However, these approximate learning-based solutions still suffer from constraint violations. From this perspective, reaching a reliable convergence remains an open challenge to DL models even with state-of-the-art methods to impose constraints, especially when facing a large set of nonlinear constraints forming a non-convex feasible set. In this paper, we propose the use of homotopy meta-optimization heuristics which creates a continuous transformation of the objective and constraints during training, to promote a more reliable convergence where the solution feasibility can be further improved. The method developed in this work includes 1) general-purpose homotopy heuristics based on the relaxation of objectives and constraint bounds to enlarge the basin of attraction and 2) physics-informed transformation of domain problem leading to trivial starting points lying within the basin of attraction. Experimentally, we demonstrate the efficacy of the proposed method on a set of abstract constrained optimization problems and real-world power grid optimal power flow problems with increasing complexity. Results show that constrained deep learning models with homotopy heuristics can improve the feasibility of the resulting solutions while achieving near-optimal objective values when compared with non-homotopy counterparts.
更多查看译文
关键词
homotopy,deep learning,constrained optimization,nonlinear programming,constrained deep learning,differentiable parametric programming
AI 理解论文
溯源树
样例
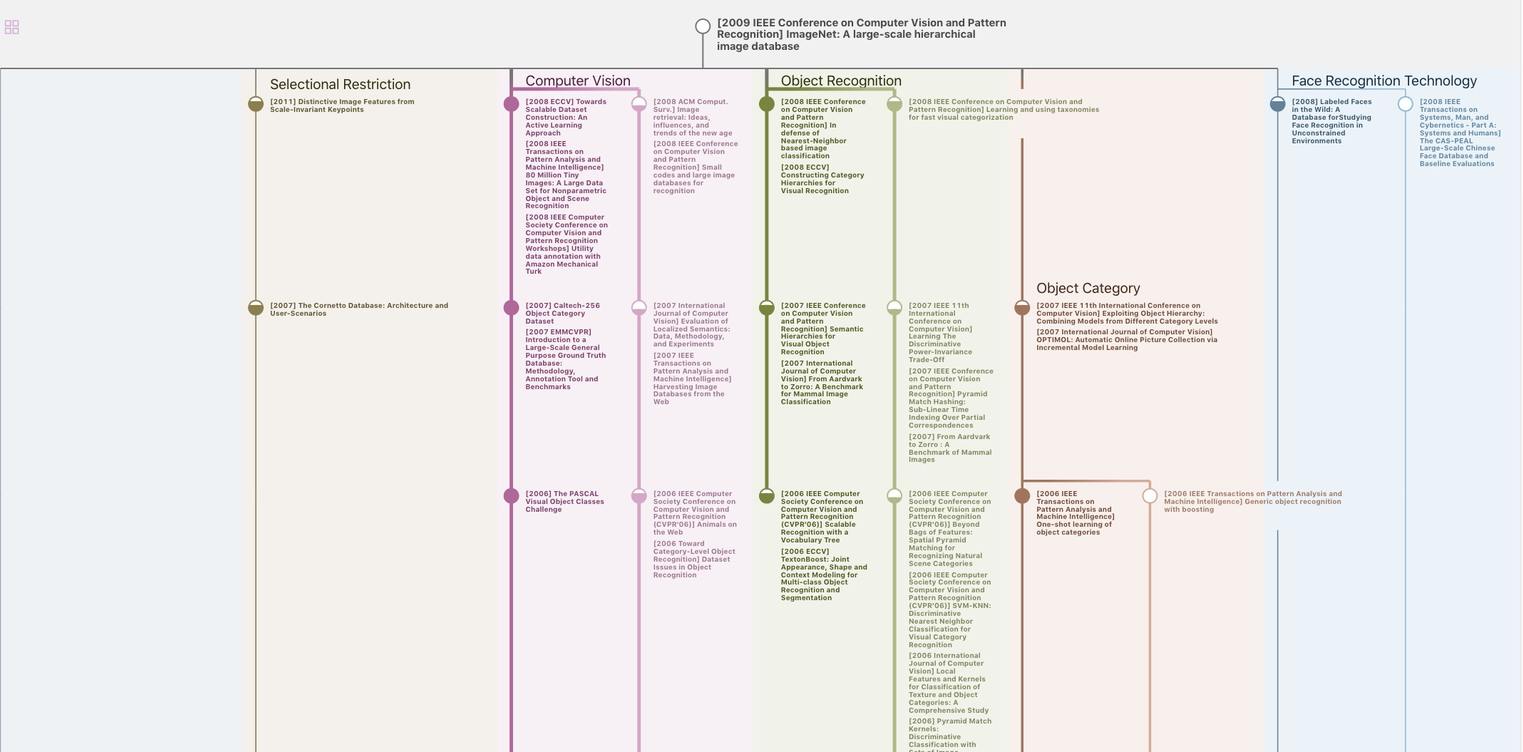
生成溯源树,研究论文发展脉络
Chat Paper
正在生成论文摘要