Towards Efficient Gradient-Based Meta-Learning in Heterogenous Environments
ICLR 2023(2023)
摘要
A challenging problem for machine learning is few-shot learning, as its models usually require many training samples. Since meta-learning models have strong fine-tuning capabilities for the distribution of tasks, many of them have been applied to few-shot learning. Model-agnostic meta-learning (MAML) is one of the most popular ones. Recent studies showed that MAML-trained models tend to reuse learned features and do not perform strong adaption, especially in the earlier layers. This paper presents an in-detail analysis of this phenomenon by analyzing MAML's components of different variants. Our results show an interesting relationship between the importance of fine-tuning earlier layers and the difference in the distribution between training and testing. As a result, we determine a fundamental weakness of existing MAML variants when the task distribution is heterogeneous, e.g., the numbers of classes do not match during testing and training. We propose a novel nonparametric version of MAML that overcomes these issues while still being able to perform cross-domain adaption.
更多查看译文
关键词
few-shot learning,heterogeneous datasets,cross-domain adaptation
AI 理解论文
溯源树
样例
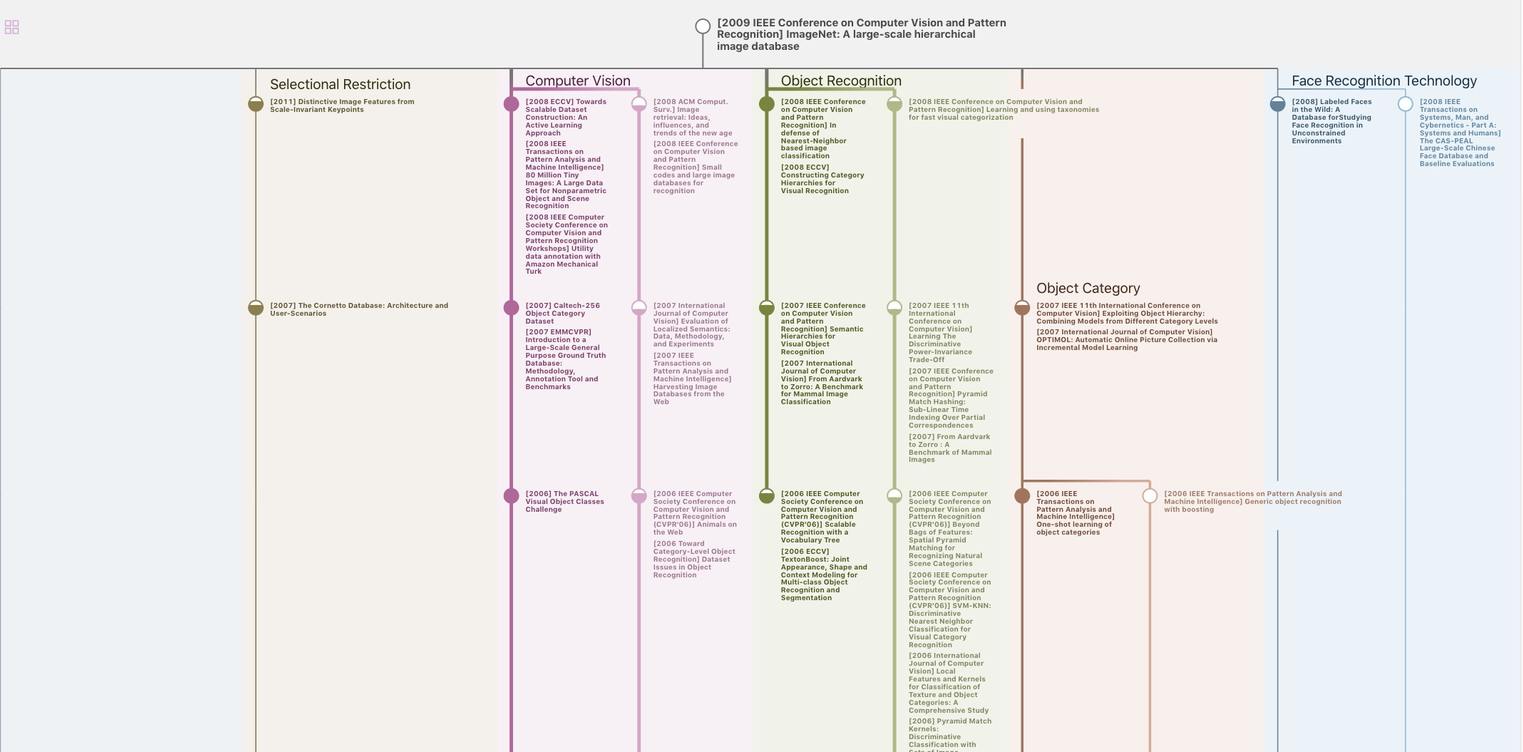
生成溯源树,研究论文发展脉络
Chat Paper
正在生成论文摘要