Progressive Knowledge Distillation: Constructing Ensembles for Efficient Inference
ICLR 2023(2023)
摘要
Knowledge distillation is commonly used to compress an ensemble of models into a single model. In this work we study the problem of progressive distillation: Given a large, pretrained teacher model $g$, we seek to decompose the model into an ensemble of smaller, low-inference cost student models $f_i$. The resulting ensemble allows for flexibly tuning accuracy vs. inference cost, which can be useful for a multitude of applications in efficient inference. Our method, B-DISTIL, uses a boosting procedure that allows function composition based aggregation rules to construct expressive ensembles with similar performance as $g$ using much smaller student models. We demonstrate the effectiveness of B-DISTIL by decomposing pretrained models across a variety of image, speech, and sensor datasets. Our method comes with strong theoretical guarantees in terms of convergence as well as generalization.
更多查看译文
关键词
progressive distillation,anytime inference,efficient inference,resource constrained ML,devices
AI 理解论文
溯源树
样例
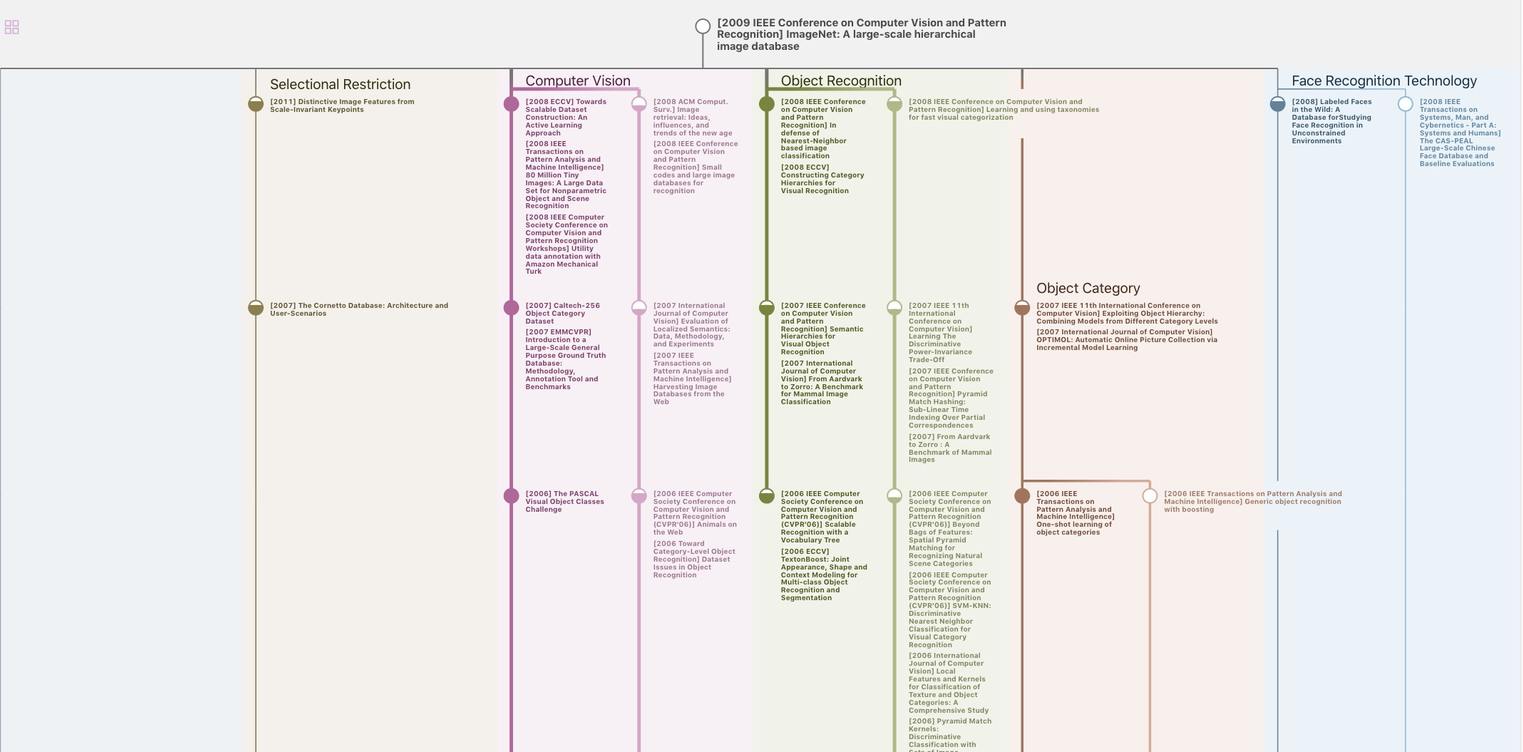
生成溯源树,研究论文发展脉络
Chat Paper
正在生成论文摘要