CrystalBox: Efficient Model-Agnostic Explanations for Deep RL Controllers
ICLR 2023(2023)
摘要
Practical adoption of Reinforcement Learning (RL) controllers is hindered by a lack of explainability. Particularly, in input-driven environments such as computer systems where the state dynamics are affected by external processes, explainability can serve as a key towards increased real-world deployment of RL controllers. In this work, we propose a novel framework, CrystalBox, for generating black-box post-hoc explanations for RL controllers in input-driven environments. CrystalBox is built on the principle of separation between policy learning and explanation computation. As the explanations are generated completely outside the training loop, CrystalBox is generalizable to a large family of input-driven RL controllers.To generate explanations, CrystalBox combines the natural decomposability of reward functions in systems environments with the explanatory power of decomposed returns. CrystalBox predicts these decomposed future returns using on policy Q-function approximations. Our design leverages two complementary approaches for this computation: sampling- and learning-based methods. We evaluate CrystalBox with RL controllers in real-world settings and demonstrate that it generates high-fidelity explanations.
更多查看译文
关键词
explainability,reinforcement learning
AI 理解论文
溯源树
样例
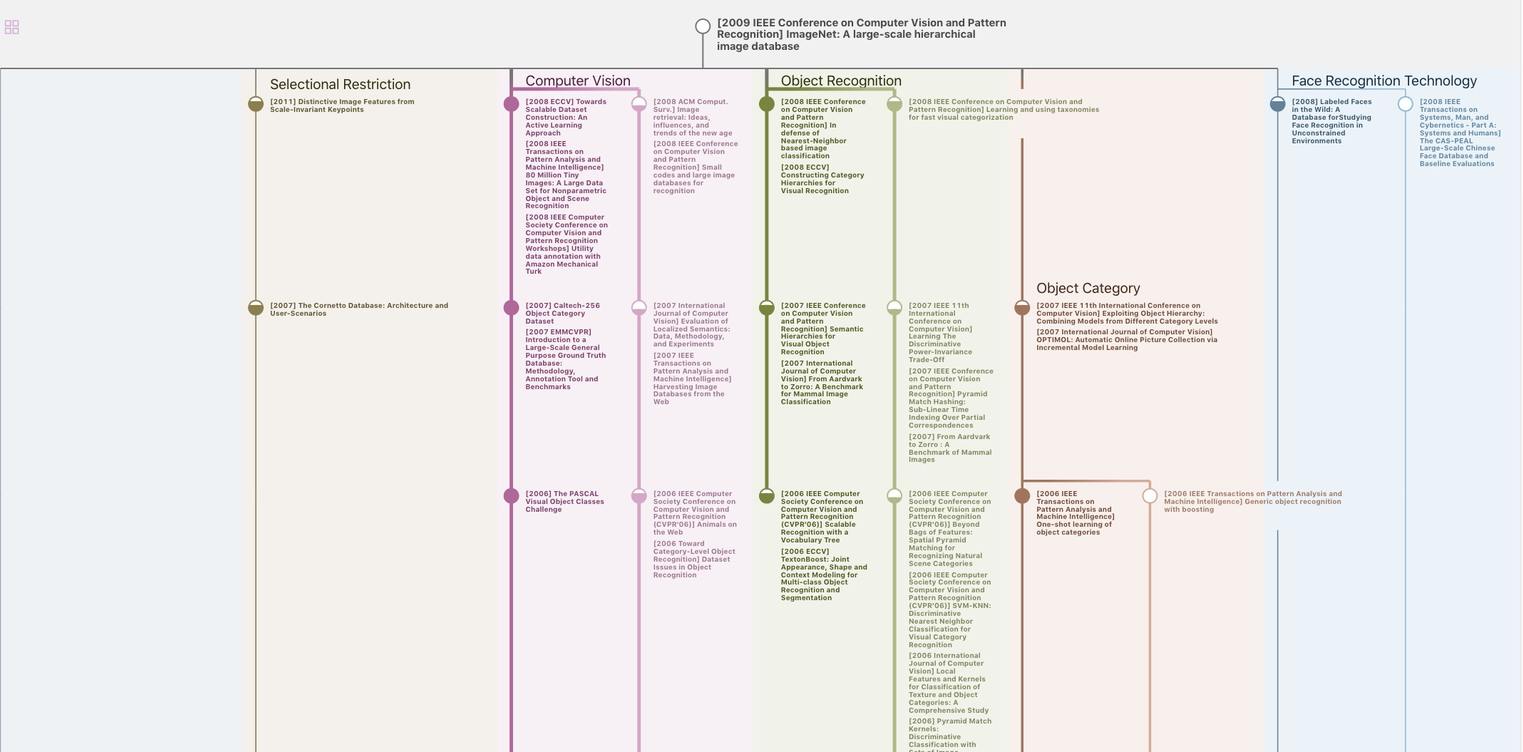
生成溯源树,研究论文发展脉络
Chat Paper
正在生成论文摘要