A Score-Based Model for Learning Neural Wavefunctions
ICLR 2023(2023)
摘要
Quantum Monte Carlo coupled with neural network wavefunctions has shown success in finding the ground state of quantum many-body systems. The existing optimization approaches compute the energy by sampling local energy from an explicit probability distribution given by the wavefunction. In this work, we provide a new optimization framework for obtaining properties of quantum many-body ground state using score-based neural networks. This new framework does not require explicit probability distribution and performs the sampling via Langevin dynamics. Our method is based on the key observation that the local energy is directly related to the score, defined as the gradient of the logarithmic wavefunction. Inspired by the score matching and the diffusion Monte Carlo methods, we derive a weighted score matching objective, which guides our score-based models to correctly converge to the ground state. We first validate our approach with experiments on quantum harmonic traps, and further results show that it can accurately learn the ground states of atomic systems. By implicitly modeling the high-dimensional data distribution, our work paves the way toward a more efficient representation of quantum systems.
更多查看译文
关键词
Neural wavefunction,Quantum Monte Carlo,Score-based method
AI 理解论文
溯源树
样例
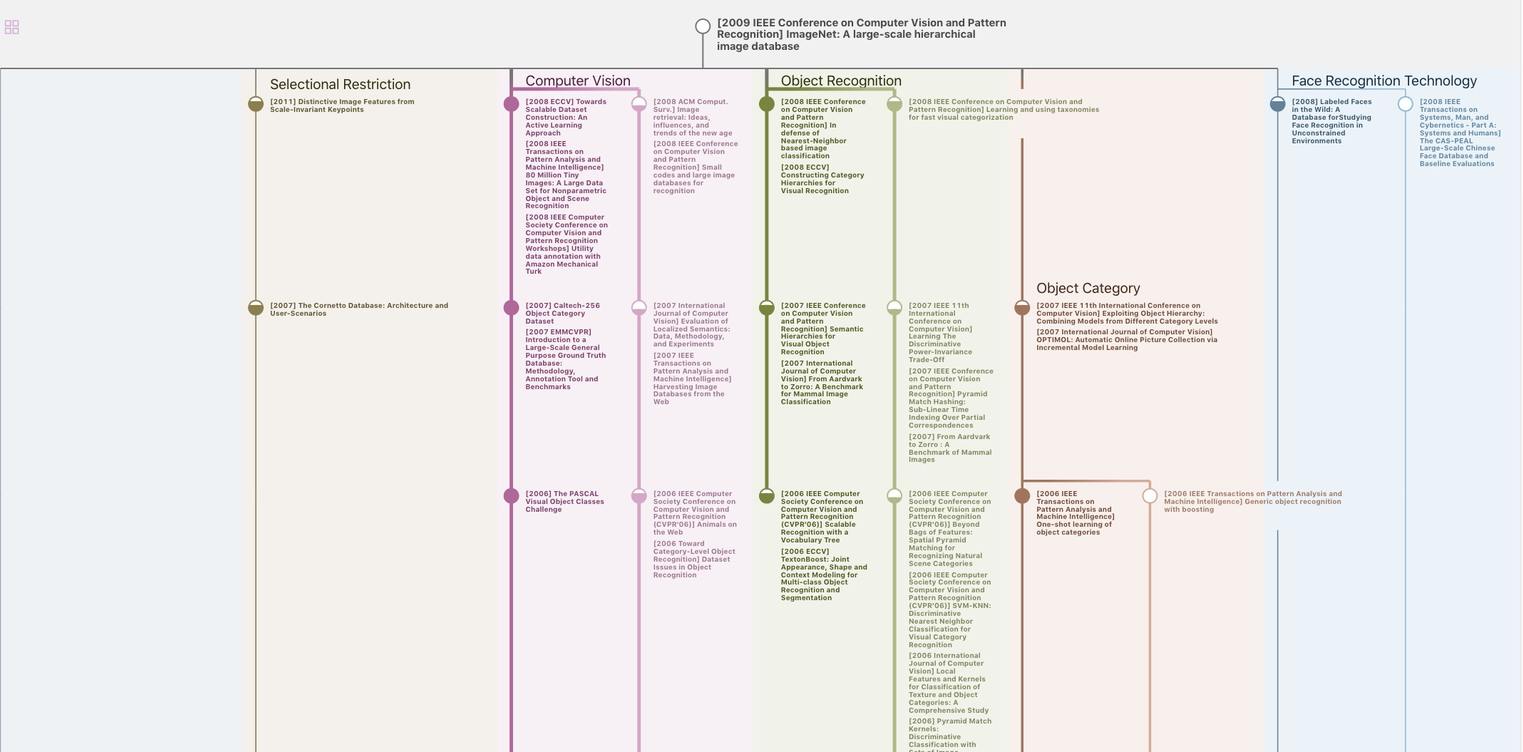
生成溯源树,研究论文发展脉络
Chat Paper
正在生成论文摘要