Do Not Blindly Imitate the Teacher: Loss Perturbation for Knowledge Distillation
ICLR 2023(2023)
Abstract
Knowledge distillation (KD) is a popular model compression technique to transfer knowledge from large teacher models to a small student model. Typically, the student learns to imitate the teacher by minimizing the KL divergence of its output distribution with the teacher's output distribution. We argue that such a learning objective is sub-optimal because there exists a discrepancy between the teacher's output distribution and the ground truth label distribution, and forcing the student to blindly imitate the unreliable teacher output distribution leads to inferior performance. To this end, we propose a novel knowledge distillation objective PTLoss by first representing the vanilla KL-based distillation loss function via a Maclaurin series and then perturbing the leading-order terms in this series. This perturbed loss improves the student generalizability by effectively distilling knowledge from a shifted distribution closer to the ground truth data. We also propose a method to compute this shifted teacher distribution, named Proxy Teacher, which enables us to select the perturbation coefficients in PTLoss. We theoretically show the perturbed loss reduces the deviation from the true population risk compared to the vanilla KL-based distillation loss functions. Experiments on three tasks with teachers of different scales show that our method significantly outperforms vanilla distillation loss functions and other perturbation methods.
MoreTranslated text
Key words
distillation,loss function,natural language processing
AI Read Science
Must-Reading Tree
Example
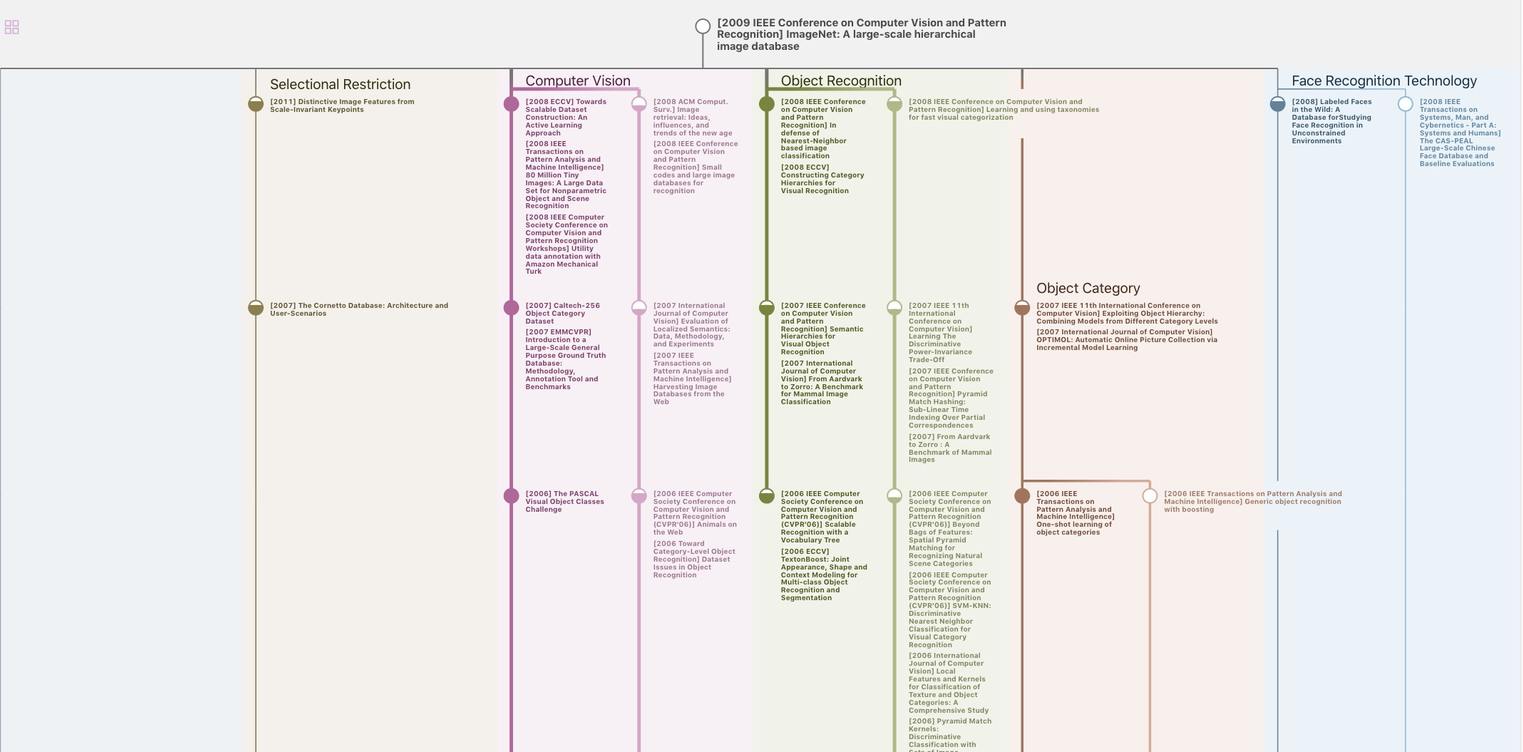
Generate MRT to find the research sequence of this paper
Chat Paper
Summary is being generated by the instructions you defined