Learning Mixture Models with Simultaneous Data Partitioning and Parameter Estimation
ICLR 2023(2023)
摘要
We study a new framework of learning mixture models via data partitioning called PRESTO, wherein we optimize a joint objective function on the model parameters and the partitioning, with each model tailored to perform well on its specific partition. We connect PRESTO to a number of past works in data partitioning, mixture models, and clustering, and show that PRESTO generalizes several loss functions including the k-means and Bregman clustering objective, the Gaussian mixture model objective, mixtures of support vector machines, and mixtures of linear regression. We then propose a new joint discrete-continuous optimization algorithm which achieves a bounded approximation guarantee for any general loss function, thereby achieving guarantees for the afore-mentioned problems as well. We study PRESTO in the context of resource efficient deep learning, where we train smaller resource constrained models on each partition and show that it outperforms existing data partitioning and model pruning/knowledge distillation approaches, which in contrast to PRESTO, require large initial (teacher) models.
更多查看译文
关键词
mixture models,resource constrained learning
AI 理解论文
溯源树
样例
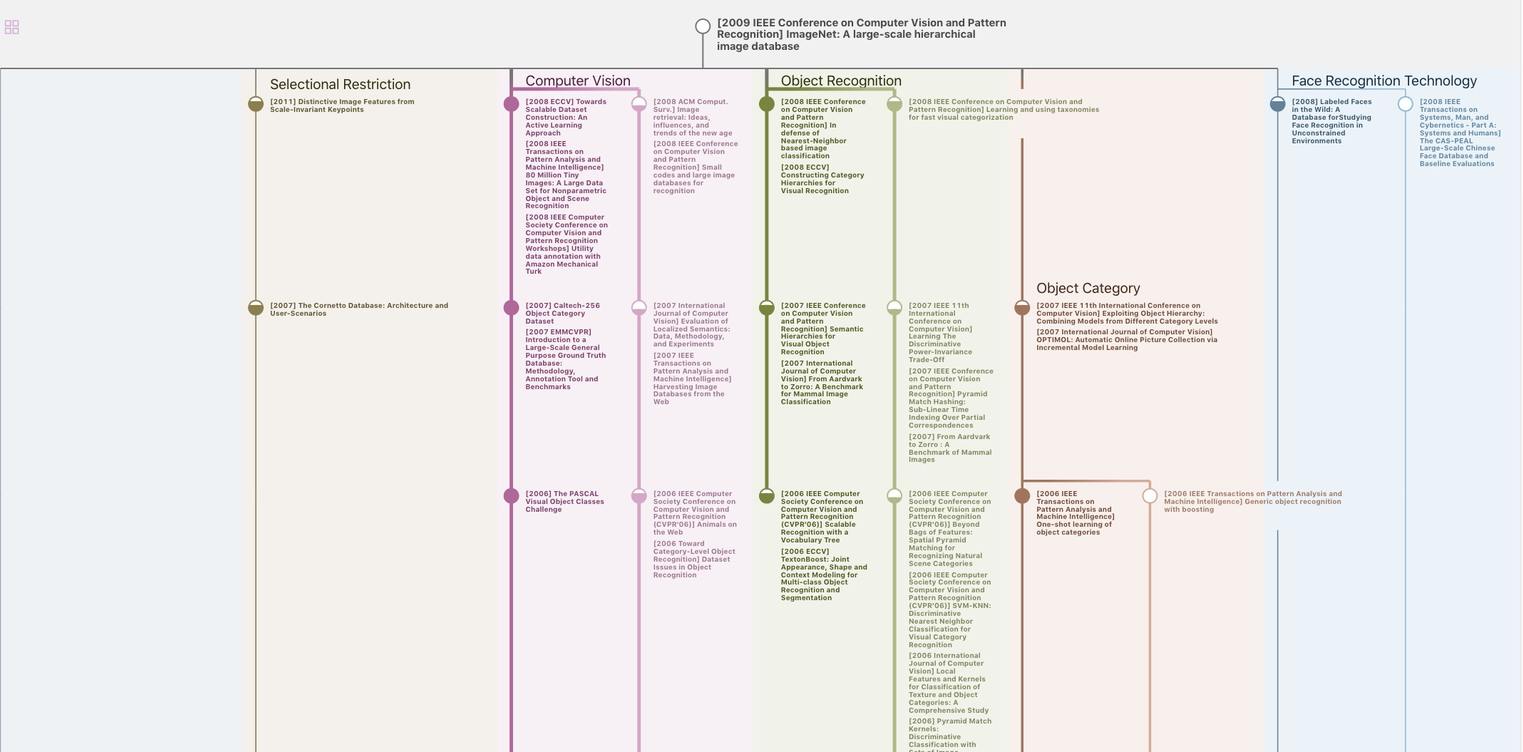
生成溯源树,研究论文发展脉络
Chat Paper
正在生成论文摘要