Untangling Effect and Side Effect: Consistent Causal Inference in Non-Targeted Trials
ICLR 2023(2023)
摘要
A treatment is usually appropriate for some group (the ``sick" group) on whom it has an effect, but it can also have a side-effect when given to subjects from another group (the ``healthy" group). In a non-targeted trial both sick and healthy subjects may be treated, producing
heterogeneous effects within the treated group. Inferring the correct treatment effect on the sick population is then
difficult, because the effect and side-effect are tangled. We propose an efficient nonparametric approach to untangling the effect and side-effect, called PCM (pre-cluster and merge). We prove its asymptotic consistency in a general setting and
show, on synthetic data,
more than a 10x improvement in accuracy over existing state-of-the-art.
更多查看译文
关键词
Causal Inference,Non Targeted Trials,Machine Learning,Heterogeneous Treatment Effects
AI 理解论文
溯源树
样例
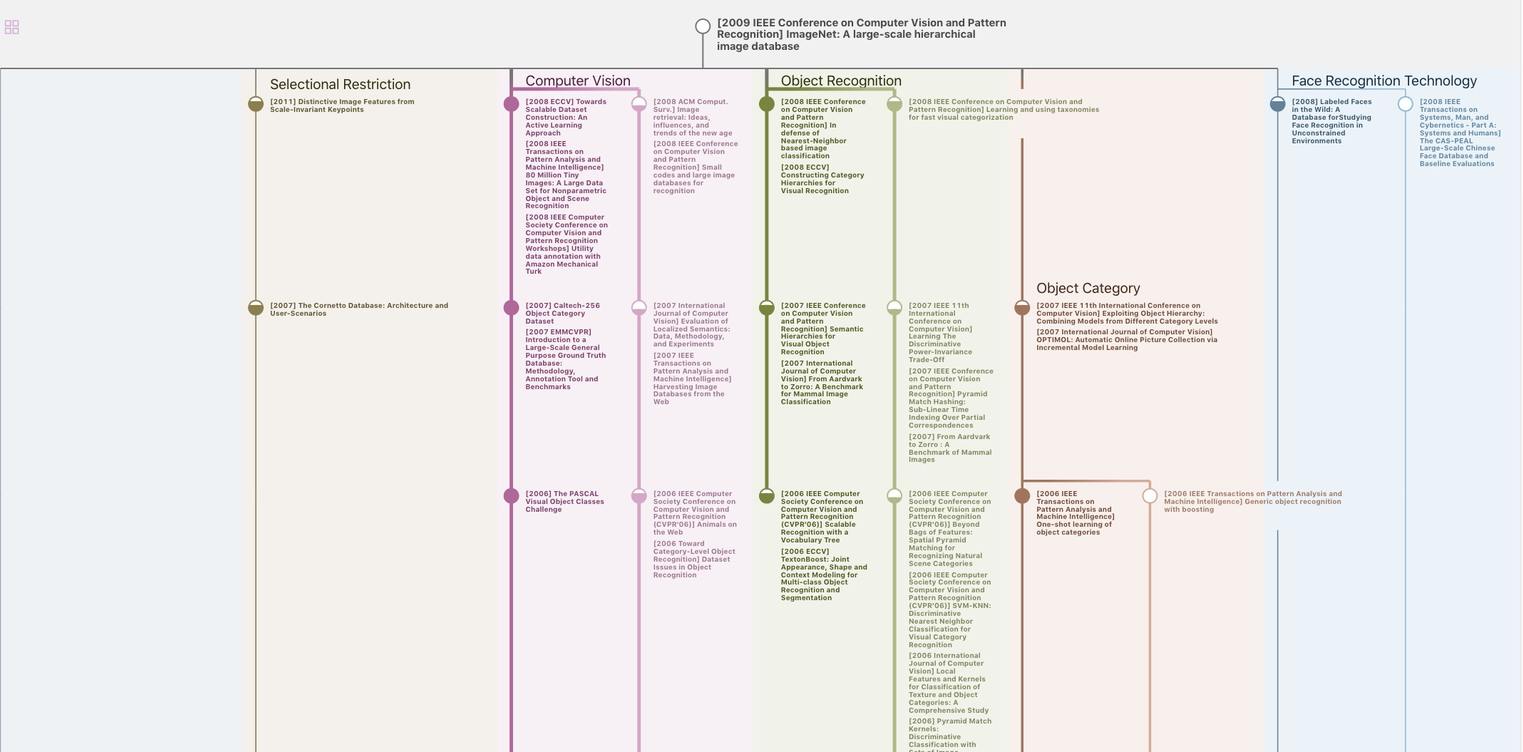
生成溯源树,研究论文发展脉络
Chat Paper
正在生成论文摘要