Efficient Bayesian Optimization with Deep Kernel Learning and Transformer Pre-trained on Multiple Heterogeneous Datasets
ICLR 2023(2023)
摘要
Bayesian optimization (BO) is widely adopted in black-box optimization problems and it relies on a surrogate model to approximate the black-box response function. With the increasing number of black-box optimization tasks solved and even more to solve, the ability to learn from multiple prior tasks to jointly pre-train a surrogate model is long-awaited to further boost optimization efficiency. In this paper, we propose a simple approach to pre-train a surrogate, which is a Gaussian process (GP) with a kernel defined on deep features learned from a Transformer-based encoder, using datasets from prior tasks with possibly heterogeneous input spaces. In addition, we provide a simple yet effective mix-up initialization strategy for input tokens corresponding to unseen input variables and therefore accelerate new tasks' convergence. Experiments on both synthetic and real benchmark problems demonstrate the effectiveness of our proposed pre-training and transfer BO strategy over existing methods.
更多查看译文
关键词
efficient bayesian optimization,deep kernel learning,multiple heterogeneous datasets,pre-trained
AI 理解论文
溯源树
样例
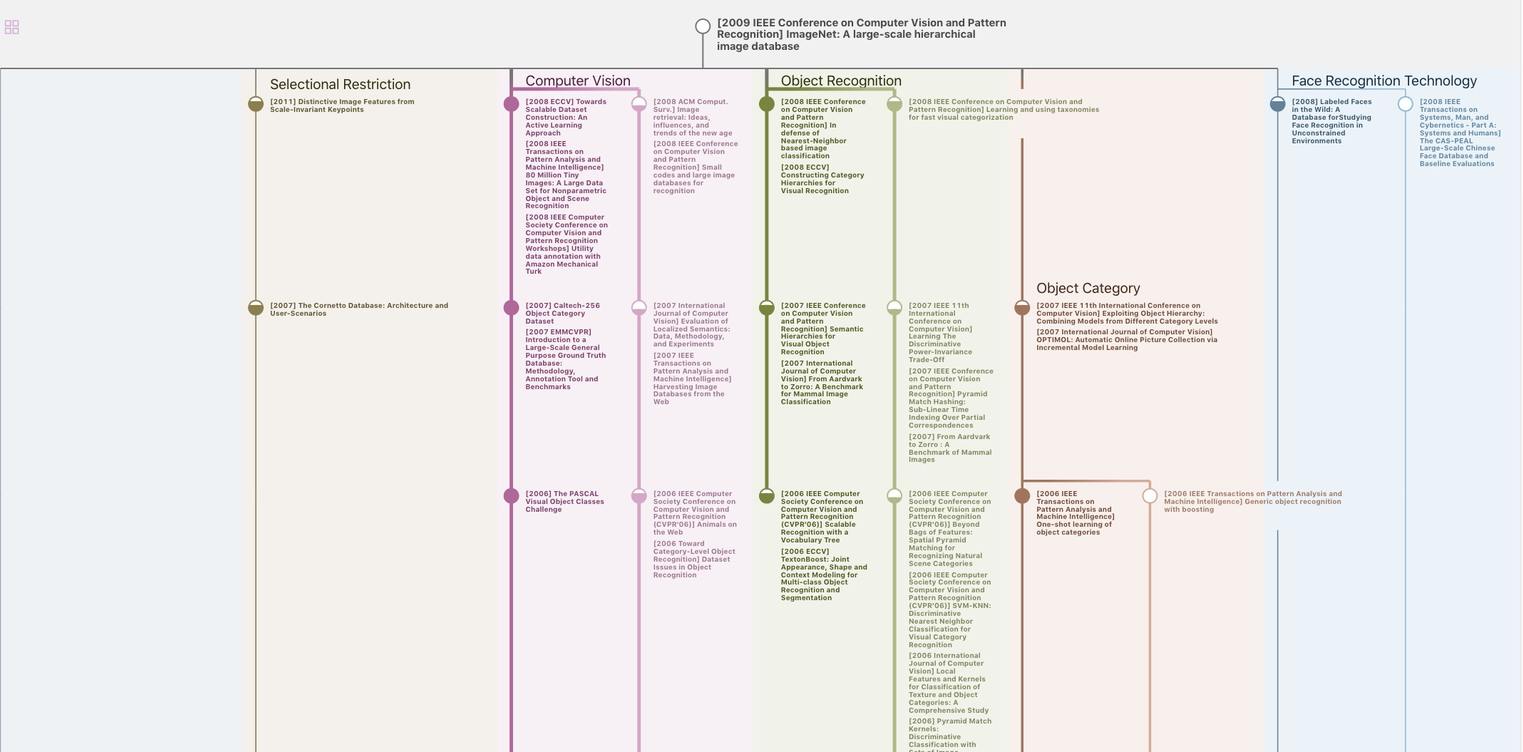
生成溯源树,研究论文发展脉络
Chat Paper
正在生成论文摘要