MCTransformer: Combining Transformers And Monte-Carlo Tree Search For Offline Reinforcement Learning
ICLR 2023(2023)
摘要
Recent studies explored the framing of reinforcement learning as a sequence modeling problem, and then using Transformers to generate effective solutions. In this study, we introduce MCTransformer, a framework that combines Monte-Carlo Tree Search (MCTS) with Transformers. Our approach uses an actor-critic setup, where the MCTS component is responsible for navigating previously-explored states, aided by input from the Transformer. The Transformer controls the exploration and evaluation of new states, enabling an effective and efficient evaluation of various strategies. In addition to the development of highly effective strategies, our setup enables the use of more efficient sampling compared to existing MCTS-based solutions. MCTransformer is therefore able to perform a small number of evaluations for each newly-explored node, and to do so without degrading its performance. Our evaluation, conducted on the challenging and well-known problem of SameGame, shows that our approach outperforms both Transformer-based and MCTS-based solutions.
更多查看译文
关键词
Transformer,Monte Carlo Tree Search,Offline Reinforcement Learning,SameGame
AI 理解论文
溯源树
样例
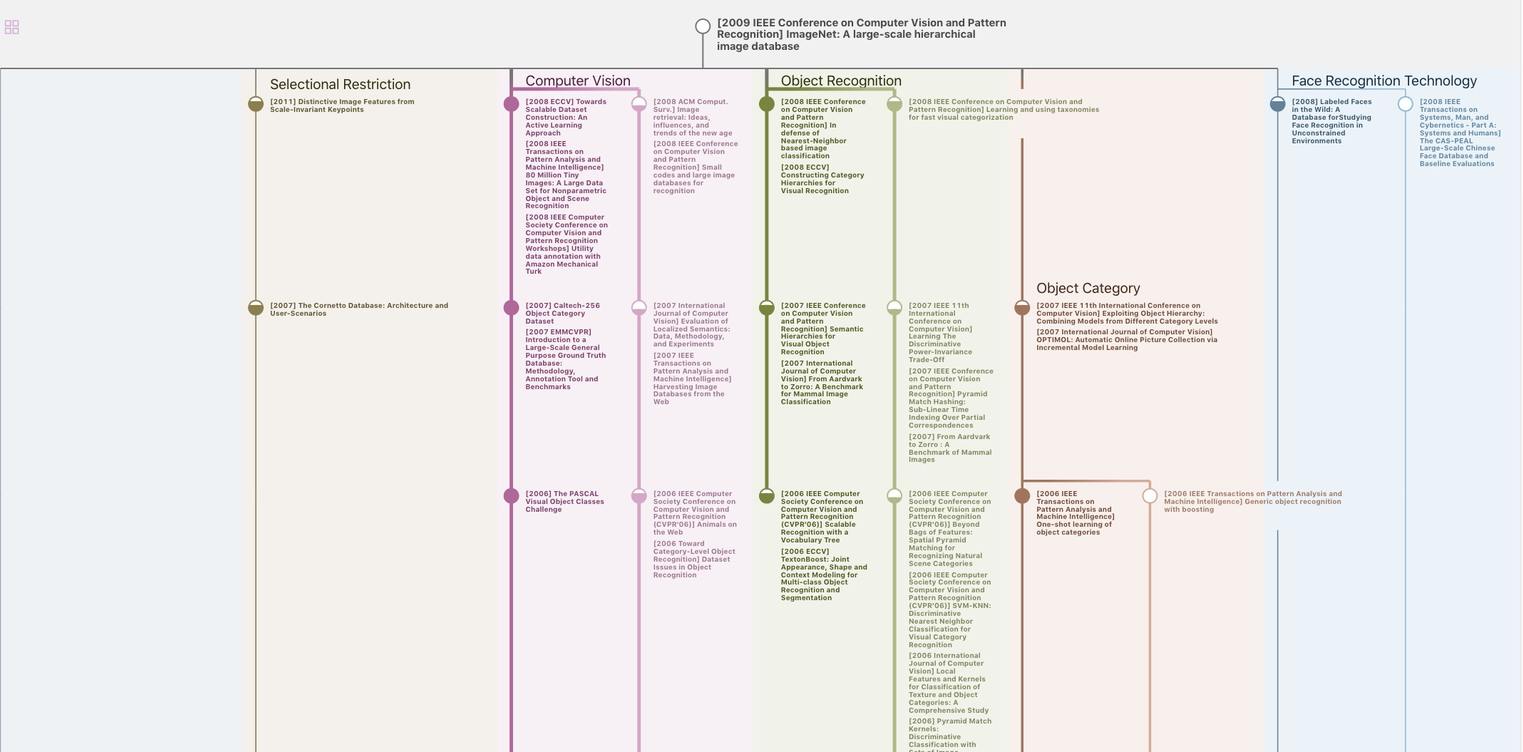
生成溯源树,研究论文发展脉络
Chat Paper
正在生成论文摘要