The guide and the explorer: smart agents for resource-limited iterated batch reinforcement learning
ICLR 2023(2023)
摘要
Iterated (a.k.a growing) batch reinforcement learning (RL) is a growing subfield fueled by the demand from systems engineers for intelligent control solutions that they can apply within their technical and organizational constraints. Model-based RL (MBRL) suits this scenario well for its sample efficiency and modularity. Recent MBRL techniques combine efficient neural system models with classical planning (like model predictive control; MPC). In this paper we add two components to this classical setup. The first is a Dyna-style policy learned on the system model using model-free techniques. We call it the guide since it guides the planner. The second component is the explorer, a strategy to expand the limited knowledge of the guide during planning. Through a rigorous ablation study we show that combination of these two ingredients is crucial for optimal performance and better data efficiency. We apply this approach with an off-policy guide and a heating explorer to improve the state of the art of benchmark systems addressing both discrete and continuous action spaces.
更多查看译文
关键词
Model-based reinforcement learning,Dyna,exploration,planning,offline,growing batch,iterated batch
AI 理解论文
溯源树
样例
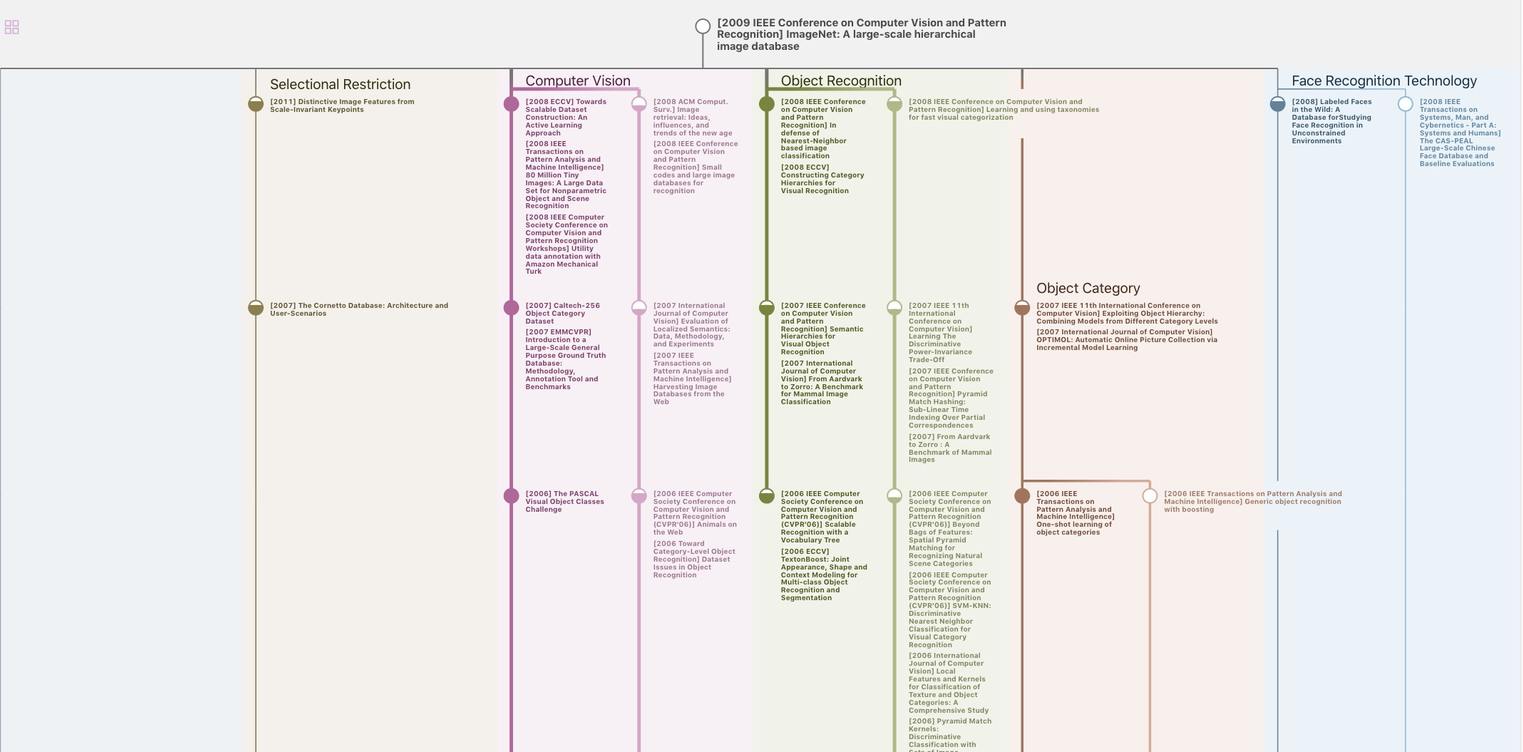
生成溯源树,研究论文发展脉络
Chat Paper
正在生成论文摘要