Towards scalable and non-IID robust Hierarchical Federated Learning via Label-driven Knowledge Aggregator
ICLR 2023(2023)
摘要
In real-world applications, Federated Learning (FL) meets two challenges: (1) scalability, especially when applied to massive IoT networks, and (2) how to be robust against an environment with heterogeneous data. Realizing the first problem, we aim to design a novel FL framework named Full-stack FL (F2L). More specifically, F2L utilizes a hierarchical network architecture, making extending the FL network accessible without reconstructing the whole network system. Moreover, leveraging the advantages of hierarchical network design, we propose a new label-driven knowledge distillation (LKD) technique at the global server to address the second problem. As opposed to current knowledge distillation techniques, LKD is capable of training a student model, which consists of good knowledge from all teachers' models. Therefore, our proposed algorithm can effectively extract the knowledge of the regions' data distribution (i.e., the regional aggregated models) to reduce the divergence between clients' models when operating under the FL system with non-independent identically distributed data. Extensive experiment results reveal that: (i) our F2L method can significantly improve the overall FL efficiency in all global distillations, and (ii) F2L rapidly achieves convergence as global distillation stages occur instead of increasing on each communication cycle.
更多查看译文
关键词
Federated Learning,Knowledge Distillation,non-IID
AI 理解论文
溯源树
样例
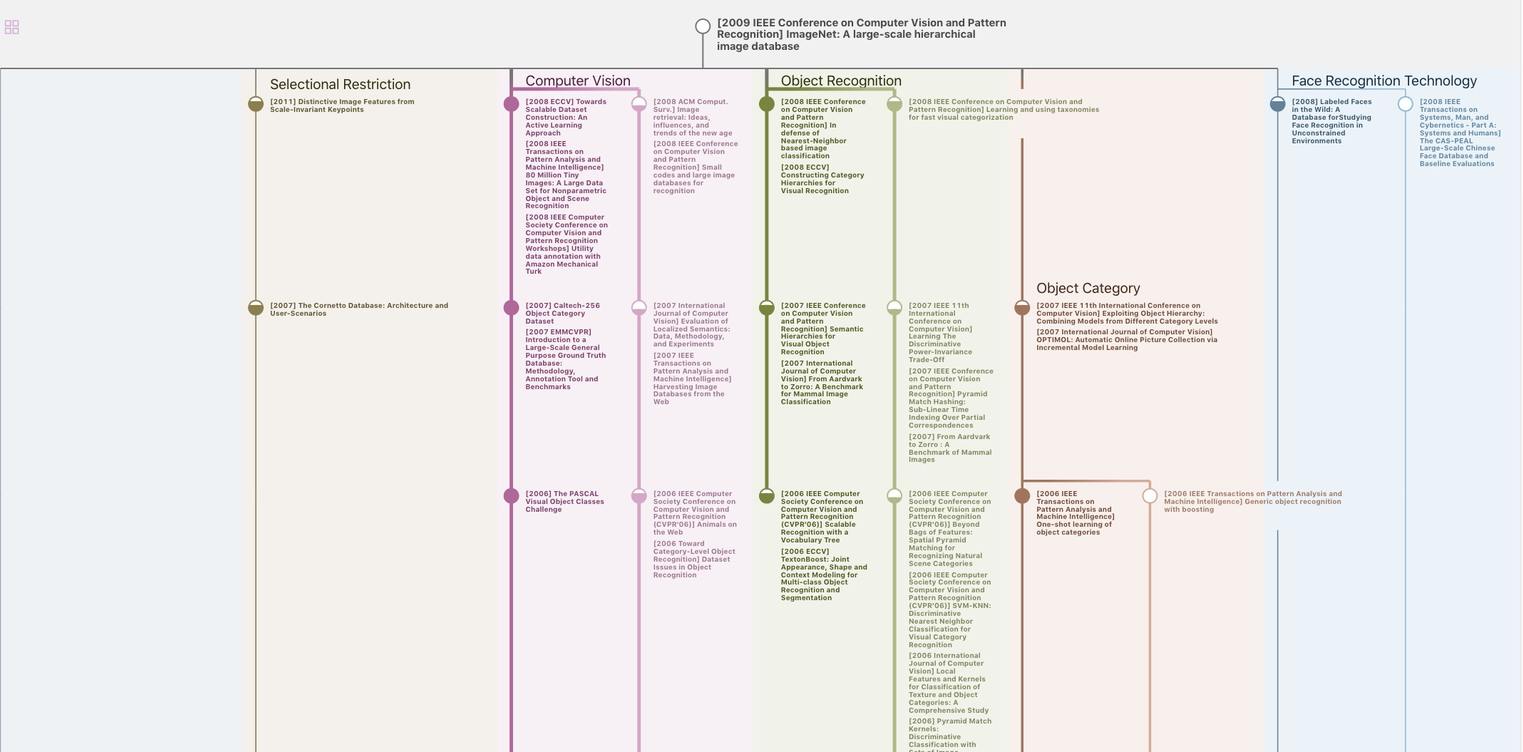
生成溯源树,研究论文发展脉络
Chat Paper
正在生成论文摘要