Designing Efficient Bit-Level Sparsity-Tolerant Memristive Networks.
IEEE transactions on neural networks and learning systems(2023)
摘要
With the rapid progress of deep neural network (DNN) applications on memristive platforms, there has been a growing interest in the acceleration and compression of memristive networks. As an emerging model optimization technique for memristive platforms, bit-level sparsity training (with the fixed-point quantization) can significantly reduce the demand for analog-to-digital converters (ADCs) resolution, which is critical for energy and area consumption. However, the bit sparsity and the fixed-point quantization will inevitably lead to a large performance loss. Different from the existing training and optimization techniques, this work attempts to explore more sparsity-tolerant architectures to compensate for performance degradation. We first empirically demonstrate that in a certain search space (e.g., 4-bit quantized DARTS space), network architectures differ in bit-level sparsity tolerance. It is reasonable and necessary to search the architectures for efficient deployment on memristive platforms by the neural architecture search (NAS) technology. We further introduce bit-level sparsity-tolerant NAS (BST-NAS), which encapsulates low-precision quantization and bit-level sparsity training into the differentiable NAS, to explore the optimal bit-level sparsity-tolerant architectures. Experimentally, with the same degree of sparsity and experiment settings, our searched architectures obtain a promising performance, which outperform the normal NAS-based DARTS-series architectures (about 5.8% higher than that of DARTS-V2 and 2.7% higher than that of PC-DARTS) on CIFAR10.
更多查看译文
关键词
Computer architecture,Quantization (signal),Training,Neural networks,Optimization,Hardware,Performance evaluation,Memristor,neural architecture search (NAS),neural network,quantization,sparsity-tolerant
AI 理解论文
溯源树
样例
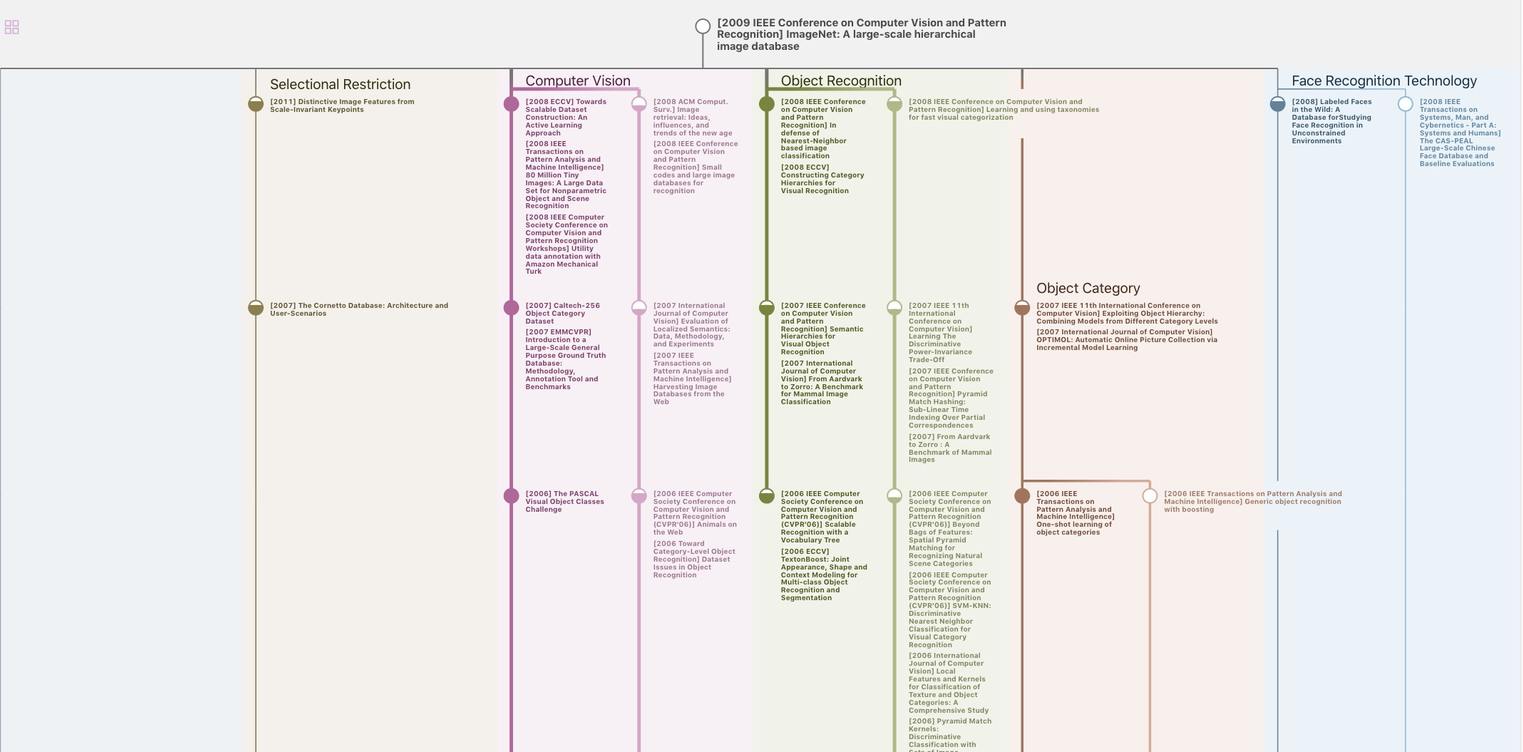
生成溯源树,研究论文发展脉络
Chat Paper
正在生成论文摘要