Local Boundary Fuzzified Rough K-Means-Based Information Granulation Algorithm Under the Principle of Justifiable Granularity
IEEE TRANSACTIONS ON CYBERNETICS(2024)
摘要
Information granularity and information granules are fundamental concepts that permeate the entire area of granular computing. With this regard, the principle of justifiable granularity was proposed by Pedrycz, and subsequently a general two-phase framework of designing information granules based on Fuzzy C-means clustering was successfully developed. This design process leads to information granules that are likely to intersect each other in substantially overlapping clusters, which inevitably leads to some ambiguity and misperception as well as loss of semantic clarity of information granules. This limitation is largely due to imprecise description of boundary-overlapping data in the existing algorithms. To address this issue, the rough k-means clustering is introduced in an innovative way into Pedrycz's two-phase information granulation framework, together with the proposed local boundary fuzzy metric. To further strengthen the characteristics of support and inhibition of boundary-overlapping data, an augmented parametric version of the principle is refined. On this basis, a local boundary fuzzified rough k-means-based information granulation algorithm is developed. In this manner, the generated granules are unique and representative whilst ensuring clearer boundaries. The validity and performance of this algorithm are demonstrated through the results of comparative experiments.
更多查看译文
关键词
Information granularity,local boundary fuzzy metric,overlapping data,principle of justifiable granularity,rough k-means clustering
AI 理解论文
溯源树
样例
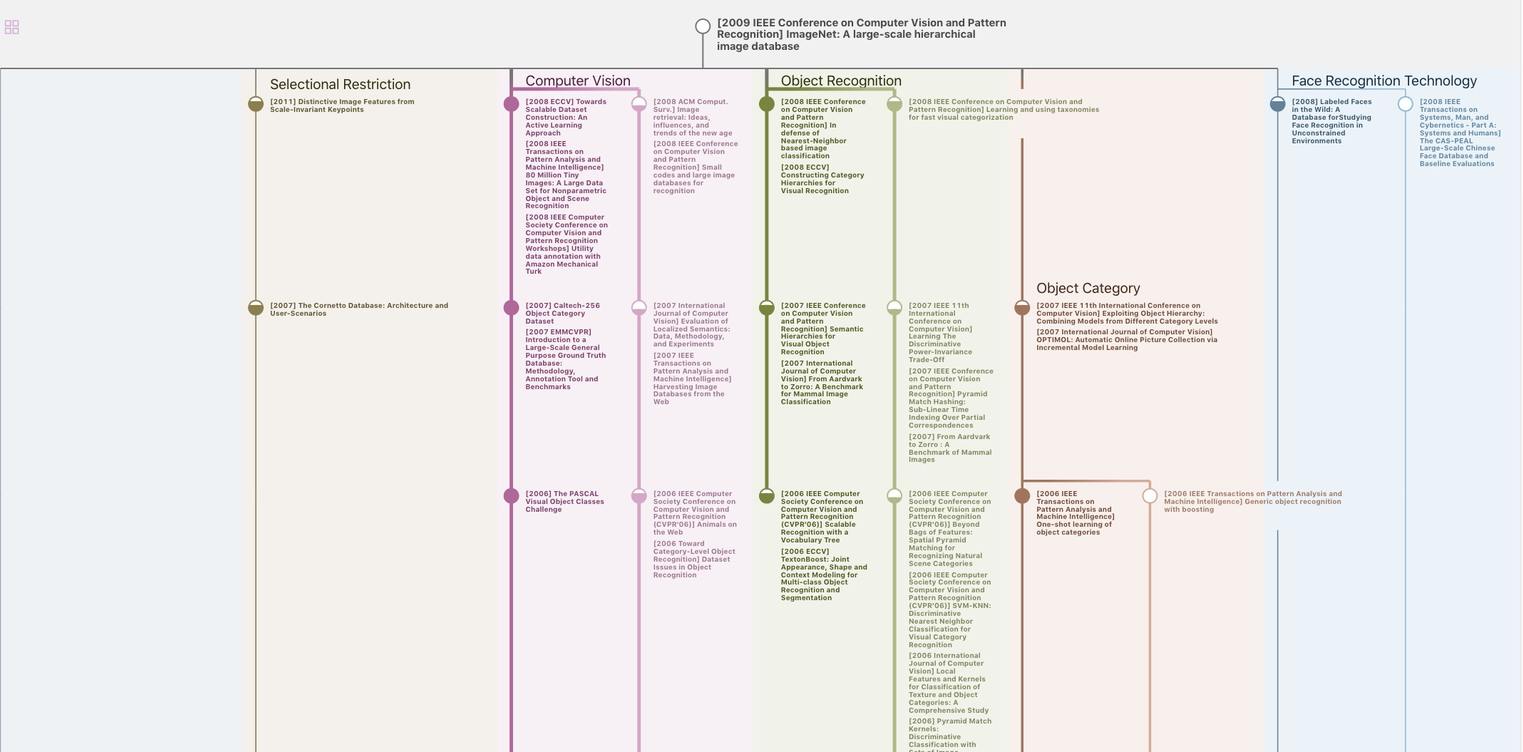
生成溯源树,研究论文发展脉络
Chat Paper
正在生成论文摘要