Modal-Regression-Based Broad Learning System for Robust Regression and Classification.
IEEE transactions on neural networks and learning systems(2023)
摘要
A novel neural network, namely, broad learning system (BLS), has shown impressive performance on various regression and classification tasks. Nevertheless, most BLS models may suffer serious performance degradation for contaminated data, since they are derived under the least-squares criterion which is sensitive to noise and outliers. To enhance the model robustness, in this article we proposed a modal-regression-based BLS (MRBLS) to tackle the regression and classification tasks of data corrupted by noise and outliers. Specifically, modal regression is adopted to train the output weights instead of the minimum mean square error (MMSE) criterion. Moreover, the l -norm-induced constraint is used to encourage row sparsity of the connection weight matrix and achieve feature selection. To effectively and efficiently train the network, the half-quadratic theory is used to optimize MRBLS. The validity and robustness of the proposed method are verified on various regression and classification datasets. The experimental results demonstrate that the proposed MRBLS achieves better performance than the existing state-of-the-art BLS methods in terms of both accuracy and robustness.
更多查看译文
关键词
Robustness,Noise measurement,Feature extraction,Data models,Standards,Learning systems,Time series analysis,Broad learning system (BLS),data regression,modal regression,pattern classification,sparsity prior
AI 理解论文
溯源树
样例
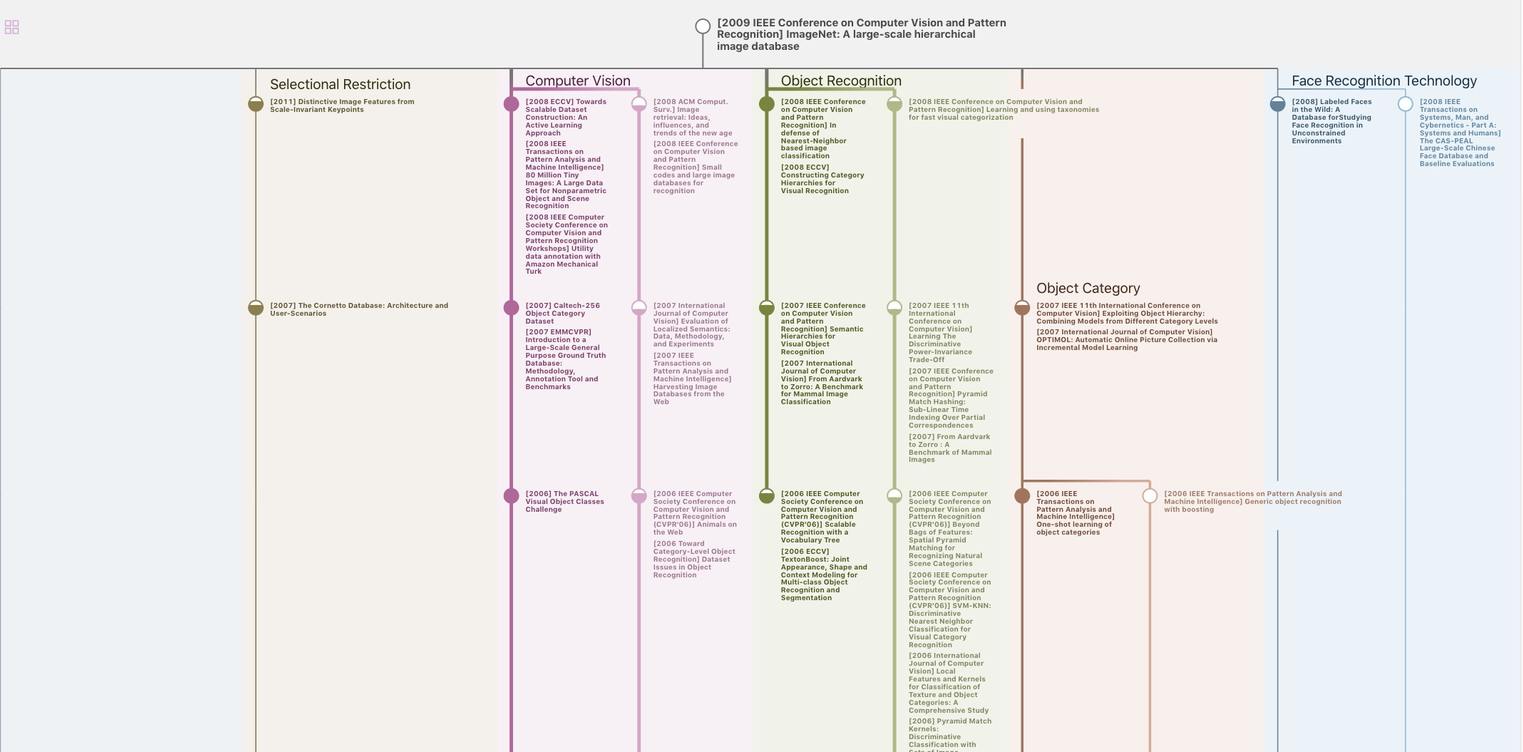
生成溯源树,研究论文发展脉络
Chat Paper
正在生成论文摘要