Implicit Posteriori Parameter Distribution Optimization in Reinforcement Learning
IEEE TRANSACTIONS ON CYBERNETICS(2024)
摘要
Efficient and intelligent exploration remains a major challenge in the field of deep reinforcement learning (DRL). Bayesian inference with a distributional representation is usually an effective way to improve the exploration ability of the RL agent. However, when optimizing Bayesian neural networks (BNNs), most algorithms need to specify an explicit parameter distribution such as a multivariate Gaussian distribution. This may reduce the flexibility of model representation and affect the algorithm performance. Therefore, to improve sample efficiency and exploration based on Bayesian methods, we propose a novel implicit posteriori parameter distribution optimization (IPPDO) algorithm. First, we adopt a distributional perspective on the parameter and model it with an implicit distribution, which is approximated by generative models. Each model corresponds to a learned latent space, providing structured stochasticity for each layer in the network. Next, to make it possible to optimize an implicit posteriori parameter distribution, we build an energy-based model (EBM) with value function to represent the implicit distribution which is not constrained by any analytic density function. Then, we design a training algorithm based on amortized Stein variational gradient descent (SVGD) to improve the model learning efficiency. We compare IPPDO with other prevailing DRL algorithms on the OpenAI Gym, MuJoCo, and Box2D platforms. Experiments on various tasks demonstrate that the proposed algorithm can represent the parameter uncertainty implicitly for a learned policy and can consistently outperform competing approaches.
更多查看译文
关键词
Bayes methods,Uncertainty,Artificial neural networks,Task analysis,Mathematical models,Inference algorithms,Generators,Bayesian inference,exploration,parameter distribution,reinforcement learning (RL)
AI 理解论文
溯源树
样例
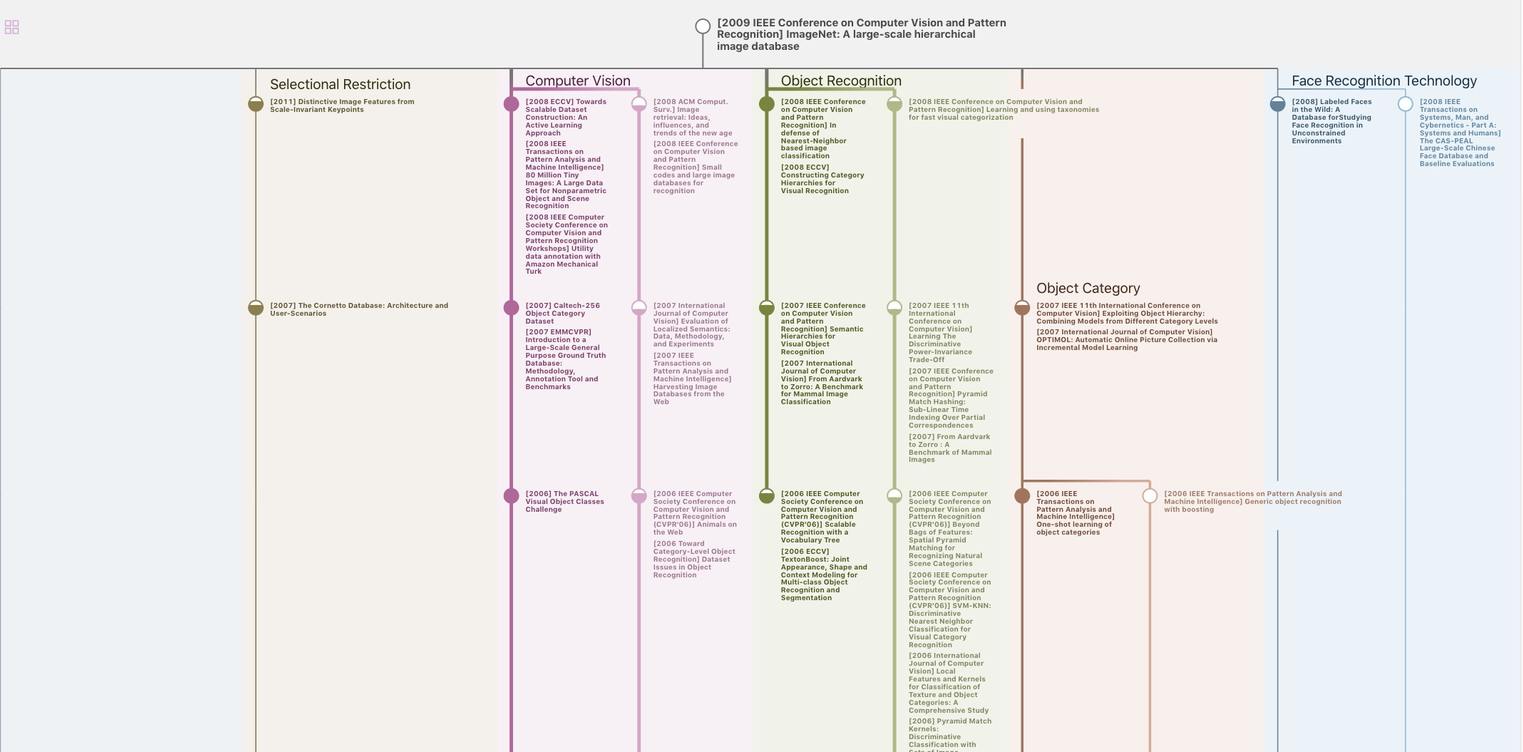
生成溯源树,研究论文发展脉络
Chat Paper
正在生成论文摘要