Machine learning from the CARDAMON trial identifies a carfilzomib-specific mutational response signature
medRxiv (Cold Spring Harbor Laboratory)(2023)
摘要
Precision medicine holds great promise to improve outcomes in cancer, including haematological malignancies. However, there are few biomarkers that influence choice of chemotherapy in clinical practice. In particular, multiple myeloma requires an individualized approach as there exist several active therapies, but little agreement on how and when they should be used and combined. We have previously shown that a transcriptomic signature can identify specific bortezomib- and lenalidomide-sensitivity. However, gene expression signatures are challenging to implement clinically. We reasoned that signatures based on the presence or absence of gene mutations would be more tractable in the clinical setting, though examples of such signatures are rare. We performed whole exome sequencing as part of the CARDAMON trial, which employed carfilzomib-based therapy. We applied advanced machine learning approaches to discover mutational patterns predictive of treatment outcome. The resulting model accurately predicted progression-free survival (PFS) both in CARDAMON patients and in an external validation set of patients from the CoMMpass study who had received carfilzomib. The signature was specific for carfilzomib therapy and was strongly driven by genes on chromosome 1p36. Importantly, patients predicted to be carfilzomib-sensitive had a longer PFS when treated with carfilzomib/lenadlidomide/dexamethasone than with bortezomib/carfilzomib/dexamethasone. However, in those predicted to be carfilzomib-insensitive, the latter therapy may have been capable of eradicating carfilzomib-resistant clones. We propose that the signature can be used to make rational therapeutic decisions and could be incorporated into future clinical trials.
更多查看译文
关键词
cardamon trial,machine learning,carfilzomib-specific
AI 理解论文
溯源树
样例
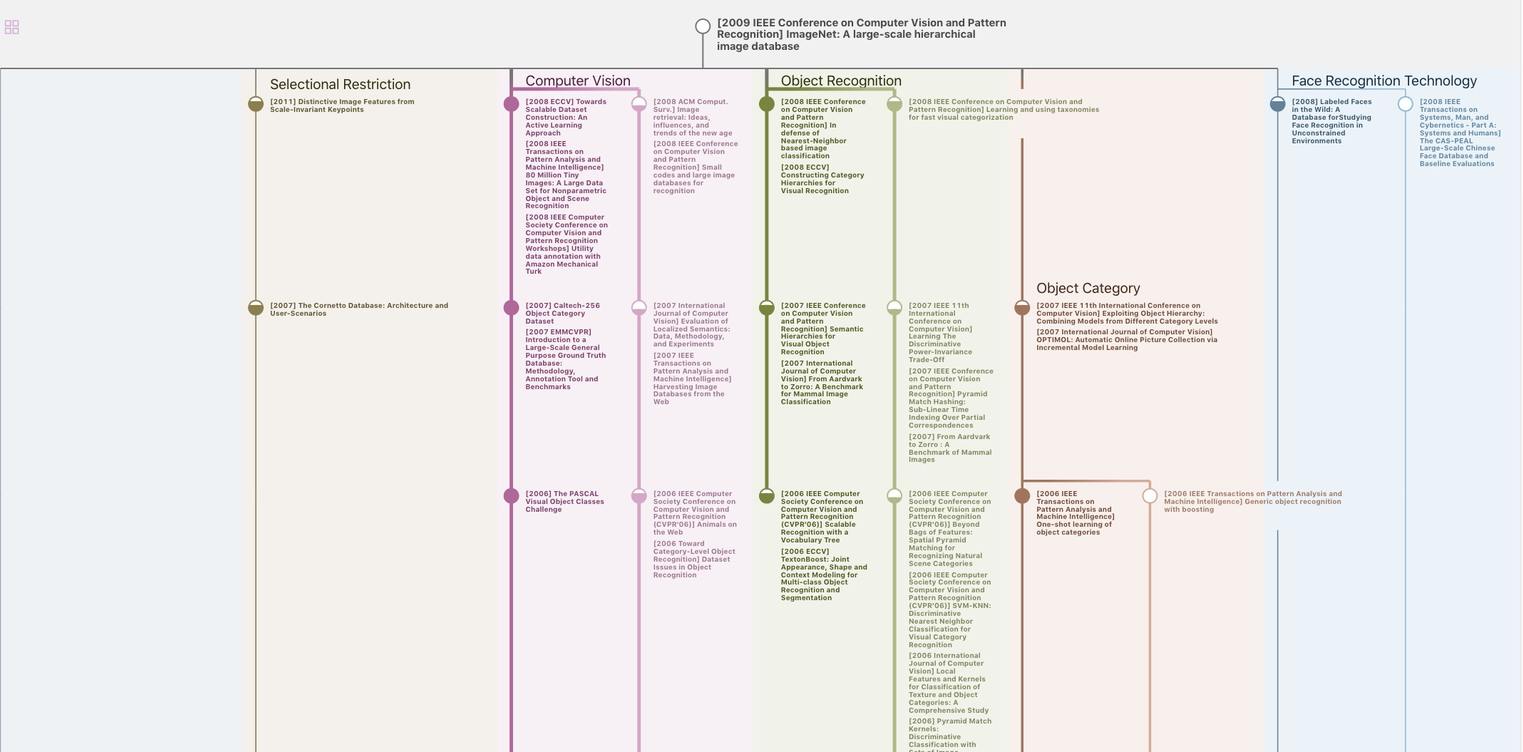
生成溯源树,研究论文发展脉络
Chat Paper
正在生成论文摘要