Stability and Replicability in Learning
2023 IEEE 64TH ANNUAL SYMPOSIUM ON FOUNDATIONS OF COMPUTER SCIENCE, FOCS(2023)
摘要
Replicability is essential in science as it allows us to validate and verify research findings. Impagliazzo, Lei, Pitassi and Sorrell ('22) recently initiated the study of replicability in machine learning. A learning algorithm is replicable if it typically produces the same output when applied on two i.i.d. inputs using the same internal randomness. We study a variant of replicability that does not involve fixing the randomness. An algorithm satisfies this form of replicability if it typically produces the same output when applied on two i.i.d. inputs (without fixing the internal randomness). This variant is called global stability and was introduced by Bun, Livni and Moran ('20) in the context of differential privacy. Impagliazzo et al. showed how to boost any replicable algorithm so that it produces the same output with probability arbitrarily close to 1. In contrast, we demonstrate that for numerous learning tasks, global stability can only be accomplished weakly, where the same output is produced only with probability bounded away from 1. To overcome this limitation, we introduce the concept of list replicability, which is equivalent to global stability. Moreover, we prove that list replicability can be boosted so that it is achieved with probability arbitrarily close to 1. We also describe basic relations between standard learning-theoretic complexity measures and list replicable numbers. Our results, in addition, imply that besides trivial cases, replicable algorithms (in the sense of Impagliazzo et al.) must be randomized. The proof of the impossibility result is based on a topological fixed-point theorem. For every algorithm, we are able to locate a "hard input distribution by applying the Poincare-Miranda theorem in a related topological setting. The equivalence between global stability and list replicability is algorithmic.
更多查看译文
关键词
Learning theory,replicability,stability,topology
AI 理解论文
溯源树
样例
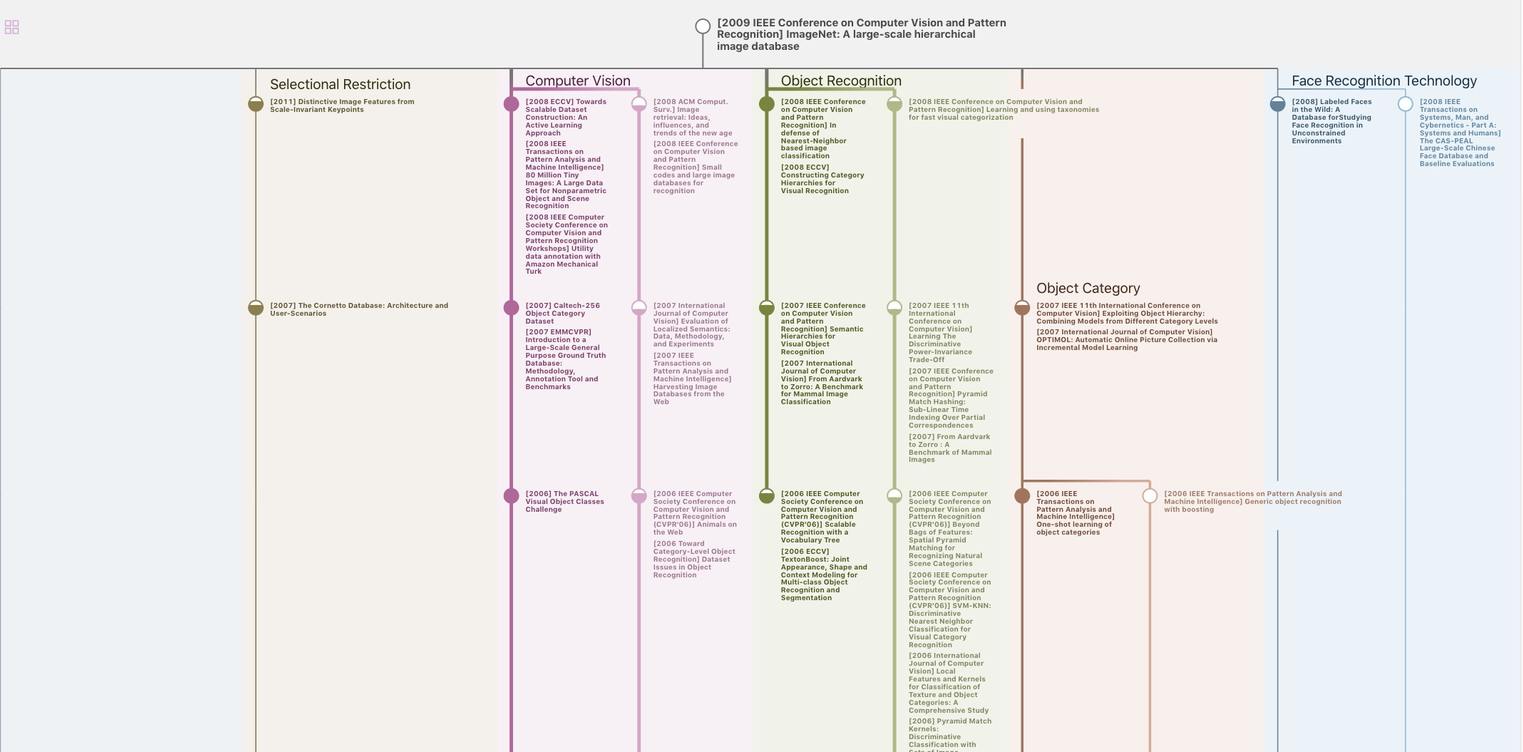
生成溯源树,研究论文发展脉络
Chat Paper
正在生成论文摘要