Solar Photovoltaic Systems Metadata Inference and Differentially Private Publication
CoRR(2023)
摘要
Stakeholders in electricity delivery infrastructure are amassing data about their system demand, use, and operations. Still, they are reluctant to share them, as even sharing aggregated or anonymized electric grid data risks the disclosure of sensitive information. This paper highlights how applying differential privacy to distributed energy resource production data can preserve the usefulness of that data for operations, planning, and research purposes without violating privacy constraints. Differentially private mechanisms can be optimized for queries of interest in the energy sector, with provable privacy and accuracy trade-offs, and can help design differentially private databases for further analysis and research. In this paper, we consider the problem of inference and publication of solar photovoltaic systems' metadata. Metadata such as nameplate capacity, surface azimuth and surface tilt may reveal personally identifiable information regarding the installation behind-the-meter. We describe a methodology to infer the metadata and propose a mechanism based on Bayesian optimization to publish the inferred metadata in a differentially private manner. The proposed mechanism is numerically validated using real-world solar power generation data.
更多查看译文
关键词
differentially private
AI 理解论文
溯源树
样例
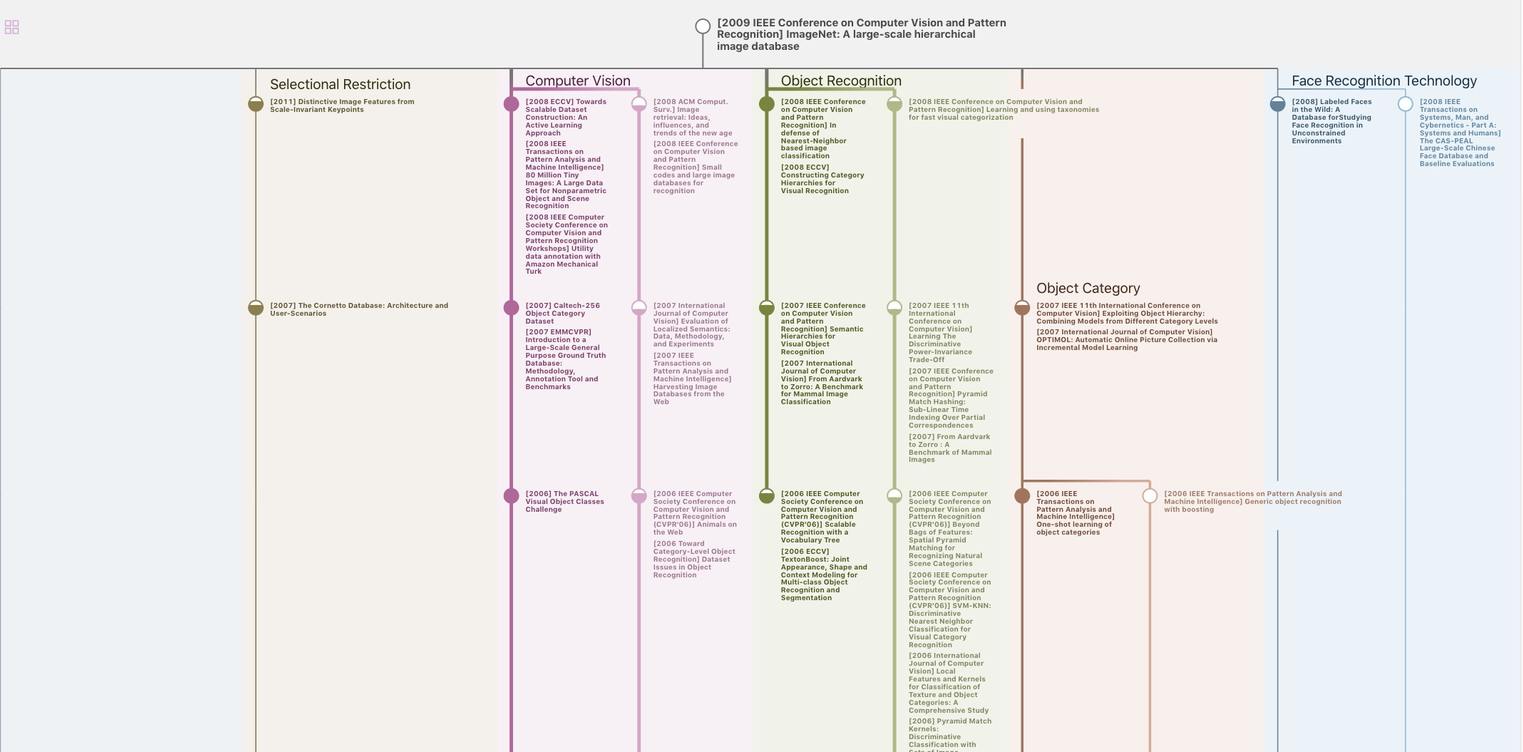
生成溯源树,研究论文发展脉络
Chat Paper
正在生成论文摘要