Domain Adaptive Multiple Instance Learning for Instance-Level Prediction of Pathological Images
CoRR(2023)
摘要
Pathological image analysis is an important process for detecting abnormalities such as cancer from cell images. However, since the image size is generally very large, the cost of providing detailed annotations is high, which makes it difficult to apply machine learning techniques. One way to improve the performance of identifying abnormalities while keeping the annotation cost low is to use only labels for each slide, or to use information from another dataset that has already been labeled. However, such weak supervisory information often does not provide sufficient performance. In this paper, we proposed a new task setting to improve the classification performance of the target dataset without increasing annotation costs. And to solve this problem, we propose a pipeline that uses multiple instance learning (MIL) and domain adaptation (DA) methods. Furthermore, in order to combine the supervisory information of both methods effectively, we propose a method to create pseudo-labels with high confidence. We conducted experiments on the pathological image dataset we created for this study and showed that the proposed method significantly improves the classification performance compared to existing methods.
更多查看译文
关键词
Pathology, Multiple Instance Learning, Domain Adaptation
AI 理解论文
溯源树
样例
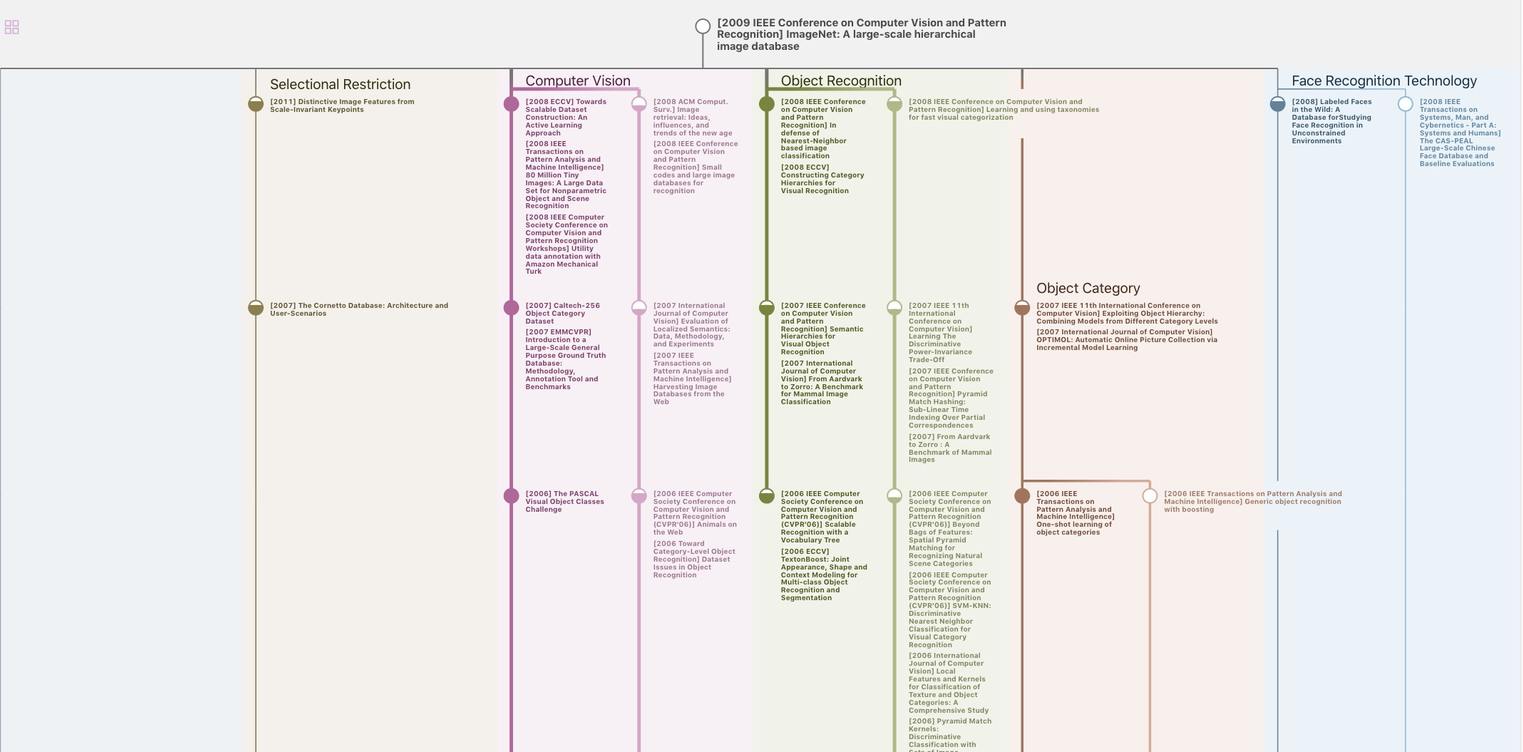
生成溯源树,研究论文发展脉络
Chat Paper
正在生成论文摘要