RED-PSM: Regularization by Denoising of Partially Separable Models for Dynamic Imaging
CoRR(2023)
摘要
Dynamic imaging addresses the recovery of a time-varying 2D or 3D object at each time instant using its undersampled measurements. In particular, in the case of dynamic tomography, only a single projection at a single view angle may be available at a time, making the problem severely ill-posed. In this work, we propose an approach, RED-PSM, which combines for the first time two powerful techniques to address this challenging imaging problem. The first, are partially separable models, which have been used to efficiently introduce a low-rank prior for the spatio-temporal object. The second is the recent Regularization by Denoising (RED), which provides a flexible framework to exploit the impressive performance of state-of-the-art image denoising algorithms, for various inverse problems. We propose a partially separable objective with RED and an optimization scheme with variable splitting and ADMM, and prove convergence of our objective to a value corresponding to a stationary point satisfying the first order optimality conditions. Convergence is accelerated by a particular projection-domain-based initialization. We demonstrate the performance and computational improvements of our proposed RED-PSM with a learned image denoiser by comparing it to a recent deep-prior-based method TD-DIP.
更多查看译文
关键词
denoising,dynamic imaging,regularization,partially separable models,red-psm
AI 理解论文
溯源树
样例
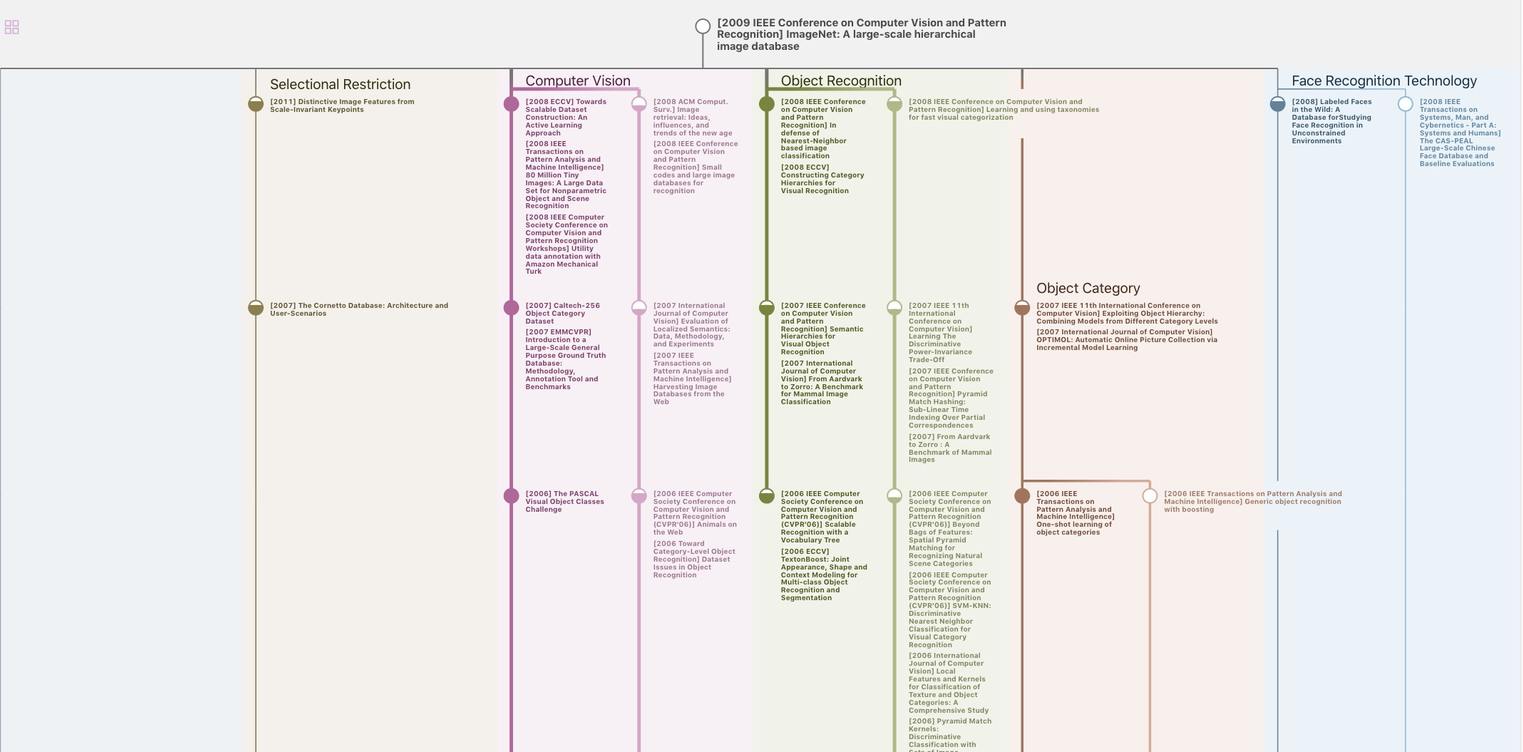
生成溯源树,研究论文发展脉络
Chat Paper
正在生成论文摘要