DSVAE: Interpretable Disentangled Representation for Synthetic Speech Detection
CoRR(2023)
摘要
Tools to generate high quality synthetic speech signal that is perceptually indistinguishable from speech recorded from human speakers are easily available. Several approaches have been proposed for detecting synthetic speech. Many of these approaches use deep learning methods as a black box without providing reasoning for the decisions they make. This limits the interpretability of these approaches. In this paper, we propose Disentangled Spectrogram Variational Auto Encoder (DSVAE) which is a two staged trained variational autoencoder that processes spectrograms of speech using disentangled representation learning to generate interpretable representations of a speech signal for detecting synthetic speech. DSVAE also creates an activation map to highlight the spectrogram regions that discriminate synthetic and bona fide human speech signals. We evaluated the representations obtained from DSVAE using the ASVspoof2019 dataset. Our experimental results show high accuracy (>98%) on detecting synthetic speech from 6 known and 10 out of 11 unknown speech synthesizers. We also visualize the representation obtained from DSVAE for 17 different speech synthesizers and verify that they are indeed interpretable and discriminate bona fide and synthetic speech from each of the synthesizers.
更多查看译文
关键词
synthetic speech,interpretable disentangled representation
AI 理解论文
溯源树
样例
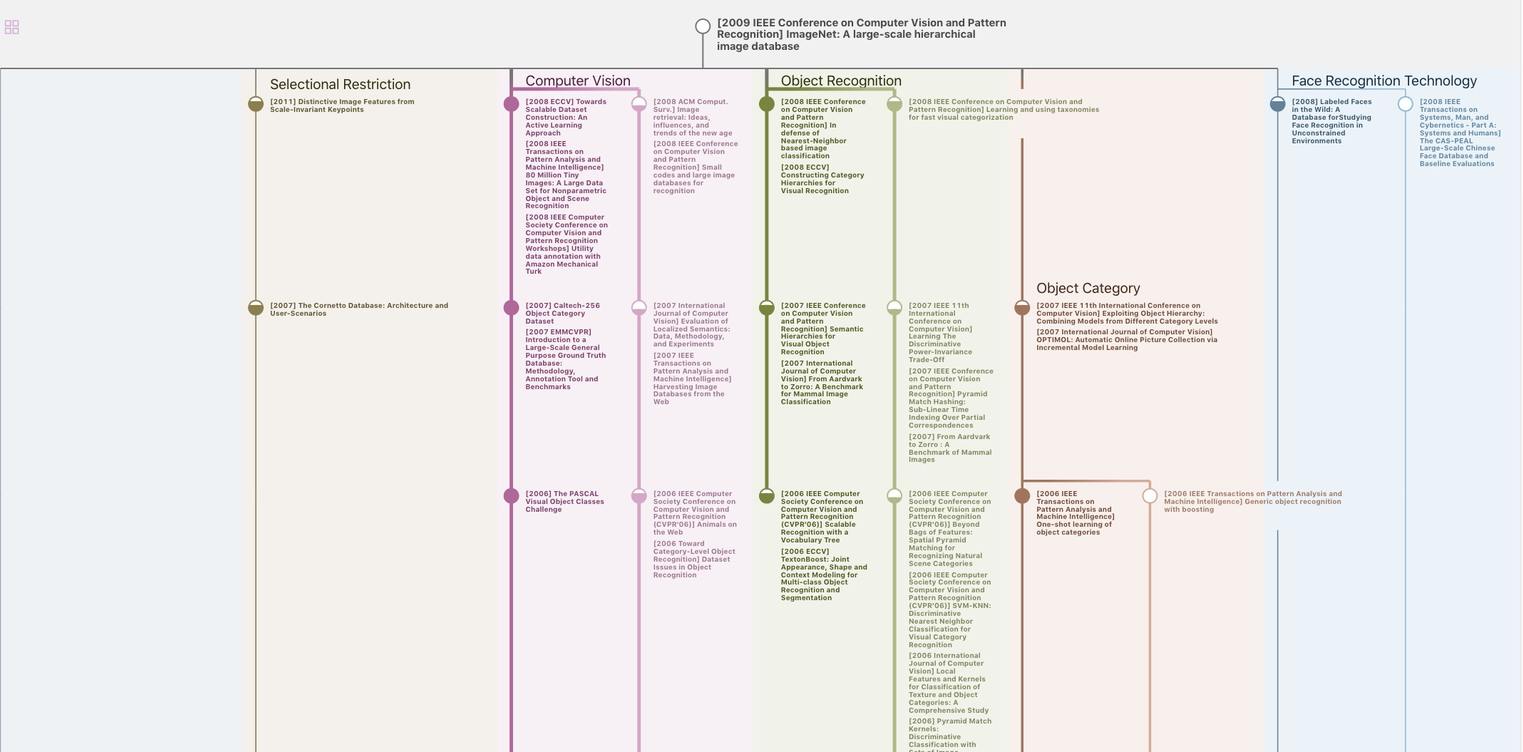
生成溯源树,研究论文发展脉络
Chat Paper
正在生成论文摘要