Taylor Neural Network for Real-World Image Super-Resolution.
IEEE transactions on image processing : a publication of the IEEE Signal Processing Society(2023)
摘要
Due to the difficulty of collecting paired Low-Resolution (LR) and High-Resolution (HR) images, the recent research on single image Super-Resolution (SR) has often been criticized for the data bottleneck of the synthetic image degradation between LRs and HRs. Recently, the emergence of real-world SR datasets, e.g., RealSR and DRealSR, promotes the exploration of Real-World image Super-Resolution (RWSR). RWSR exposes a more practical image degradation, which greatly challenges the learning capacity of deep neural networks to reconstruct high-quality images from low-quality images collected in realistic scenarios. In this paper, we explore Taylor series approximation in prevalent deep neural networks for image reconstruction, and propose a very general Taylor architecture to derive Taylor Neural Networks (TNNs) in a principled manner. Our TNN builds Taylor Modules with Taylor Skip Connections (TSCs) to approximate the feature projection functions, following the spirit of Taylor Series. TSCs introduce the input connected directly with each layer at different layers, to sequentially produces different high-order Taylor maps to attend more image details, and then aggregate the different high-order information from different layers. Only via simple skip connections, TNN is compatible with various existing neural networks to effectively learn high-order components of the input image with little increase of parameters. Furthermore, we have conducted extensive experiments to evaluate our TNNs in different backbones on two RWSR benchmarks, which achieve a superior performance in comparison with existing baseline methods.
更多查看译文
关键词
Image super-resolution,real-world super-resolution,high-order information,Taylor neural network,Taylor attention map,Taylor skip connection
AI 理解论文
溯源树
样例
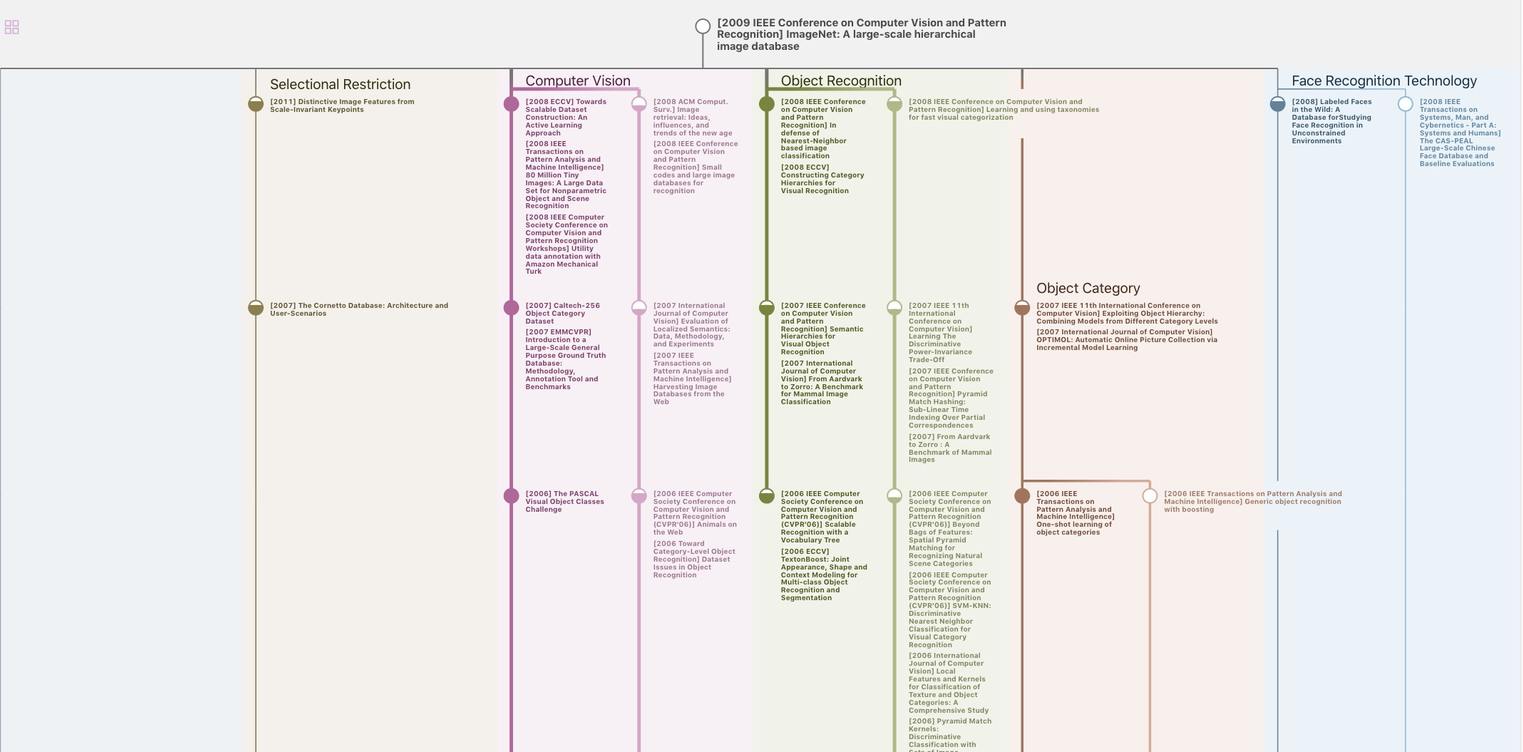
生成溯源树,研究论文发展脉络
Chat Paper
正在生成论文摘要