Learning-Based Multi-UAV Flocking Control With Limited Visual Field and Instinctive Repulsion
IEEE TRANSACTIONS ON CYBERNETICS(2024)
摘要
This article explores deep reinforcement learning (DRL) for the flocking control of unmanned aerial vehicle (UAV) swarms. The flocking control policy is trained using a centralized-learning-decentralized-execution (CTDE) paradigm, where a centralized critic network augmented with additional information about the entire UAV swarm is utilized to improve learning efficiency. Instead of learning inter-UAV collision avoidance capabilities, a repulsion function is encoded as an inner-UAV "instinct. " In addition, the UAVs can obtain the states of other UAVs through onboard sensors in communication-denied environments, and the impact of varying visual fields on flocking control is analyzed. Through extensive simulations, it is shown that the proposed policy with the repulsion function and limited visual field has a success rate of 93.8% in training environments, 85.6% in environments with a high number of UAVs, 91.2% in environments with a high number of obstacles, and 82.2% in environments with dynamic obstacles. Furthermore, the results indicate that the proposed learning-based methods are more suitable than traditional methods in cluttered environments.
更多查看译文
关键词
Autonomous aerial vehicles,Optimization,Collision avoidance,Visualization,Training,Sensors,Reinforcement learning,Deep reinforcement learning (DRL),flocking control,inter-unmanned aerial vehicle (UAV) collision avoidance,limited visual field,UAVs
AI 理解论文
溯源树
样例
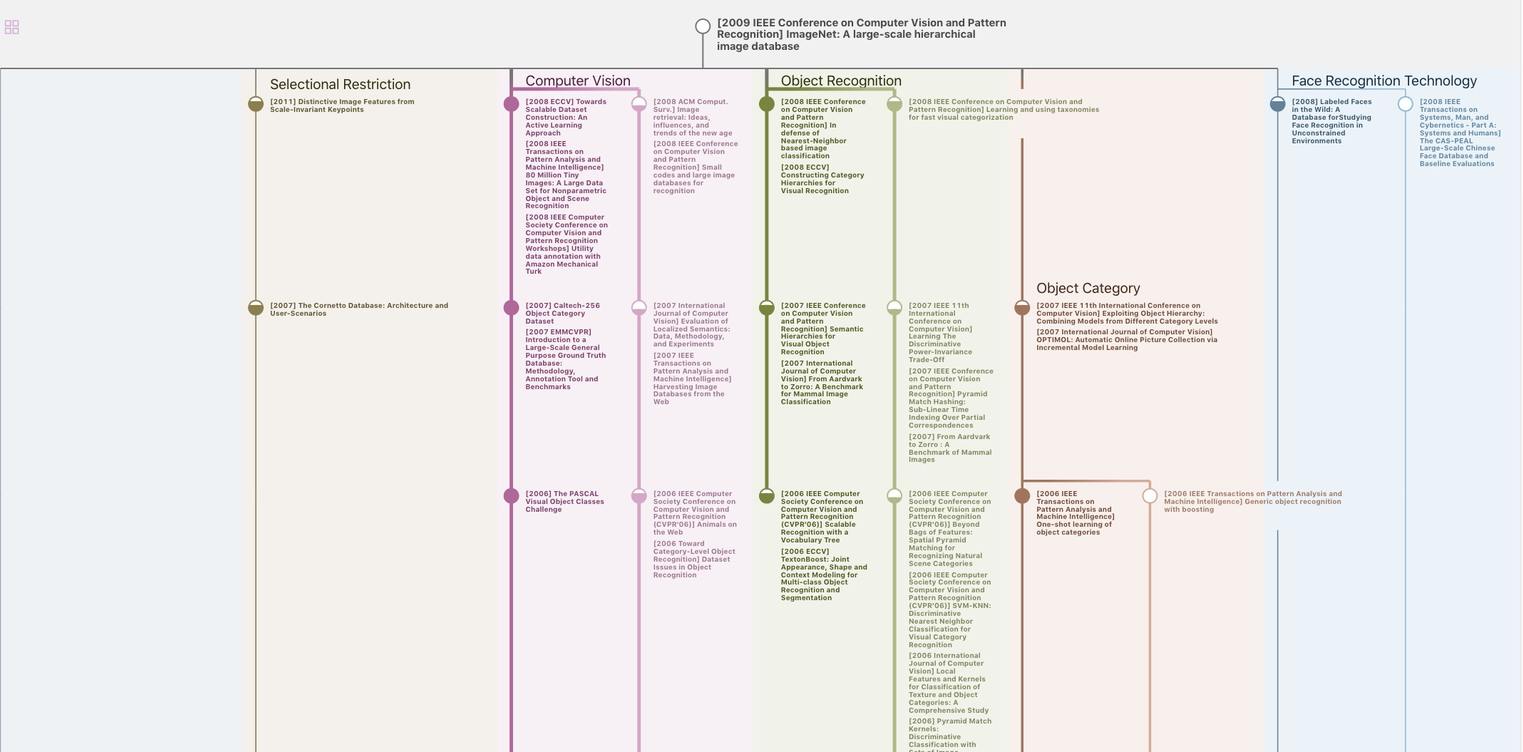
生成溯源树,研究论文发展脉络
Chat Paper
正在生成论文摘要