Adversarial Algorithm Unrolling Network for Interpretable Mechanical Anomaly Detection.
IEEE transactions on neural networks and learning systems(2023)
摘要
In mechanical anomaly detection, algorithms with higher accuracy, such as those based on artificial neural networks, are frequently constructed as black boxes, resulting in opaque interpretability in architecture and low credibility in results. This article proposes an adversarial algorithm unrolling network (AAU-Net) for interpretable mechanical anomaly detection. AAU-Net is a generative adversarial network (GAN). Its generator, composed of an encoder and a decoder, is mainly produced by algorithm unrolling of a sparse coding model, which is specially designed for feature encoding and decoding of vibration signals. Thus, AAU-Net has a mechanism-driven and interpretable network architecture. In other words, it is ad hoc interpretable. Moreover, a multiscale feature visualization approach for AAU-Net is introduced to verify that meaningful features are encoded by AAU-Net, helping users to trust the detection results. The feature visualization approach enables the results of AAU-Net to be interpretable, i.e., post hoc interpretable. To verify AAU-Net's capability of feature encoding and anomaly detection, we designed and performed simulations and experiments. The results show that AAU-Net can learn signal features that match the dynamic mechanism of the mechanical system. Considering the excellent feature learning ability, unsurprisingly, AAU-Net achieves the best overall anomaly detection performance compared with other algorithms.
更多查看译文
关键词
Adversarial training,algorithm unrolling,anomaly detection,interpretable neural network,representation learning
AI 理解论文
溯源树
样例
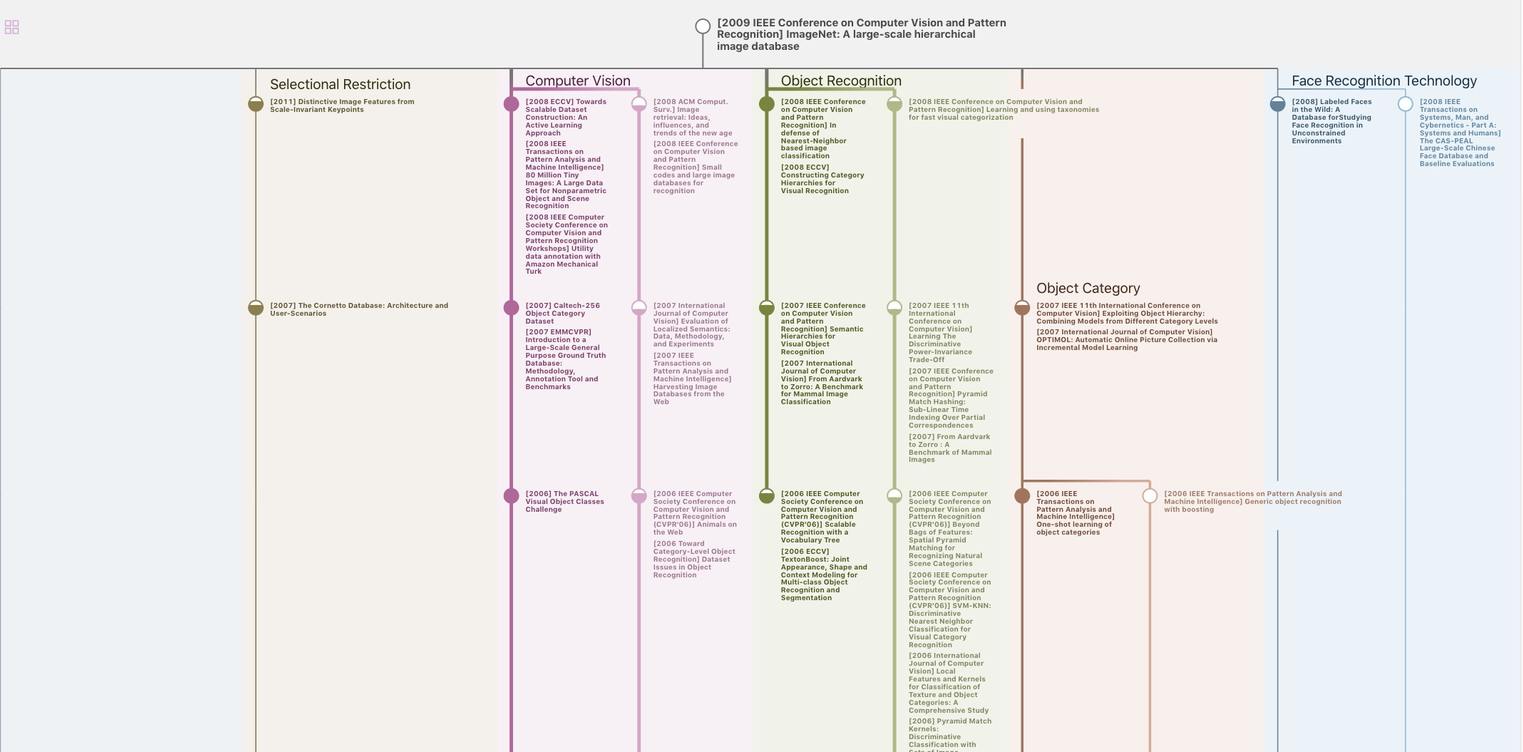
生成溯源树,研究论文发展脉络
Chat Paper
正在生成论文摘要