Segmentation of Arm Ultrasound Images in Breast Cancer-Related Lymphedema: A Database and Deep Learning Algorithm
IEEE transactions on bio-medical engineering(2023)
摘要
Objective: Breast cancer treatment often causes the removal of or damage to lymph nodes of the patient's lymphatic drainage system. This side effect is the origin of Breast Cancer-Related Lymphedema (BCRL), referring to a noticeable increase in excess arm volume. Ultrasound imaging is a preferred modality for the diagnosis and progression monitoring of BCRL because of its low cost, safety, and portability. As the affected and unaffected arms look similar in B-mode ultrasound images, the thickness of the skin, subcutaneous fat, and muscle have been shown to be important biomarkers for this task. The segmentation masks are also helpful in monitoring the longitudinal changes in morphology and mechanical properties of tissue layers. Methods: For the first time, a publicly available ultrasound dataset containing the Radio-Frequency (RF) data of 39 subjects and manual segmentation masks by two experts, are provided. Inter- and intra-observer reproducibility studies performed on the segmentation maps show a high Dice Score Coefficient (DSC) of 0.94 +/- 0.08 and 0.92 +/- 0.06, respectively. Gated Shape Convolutional Neural Network (GSCNN) is modified for precise automatic segmentation of tissue layers, and its generalization performance is improved by the CutMix augmentation strategy. Results: We got an average DSC of 0.87 +/- 0.11 on the test set, which confirms the high performance of the method. Conclusion: Automatic segmentation can pave the way for convenient and accessible staging of BCRL, and our dataset can facilitate development and validation of those methods. Significance: Timely diagnosis and treatment of BCRL have crucial importance in preventing irreversible damage.
更多查看译文
关键词
Breast cancer,lymphedema,medical ultrasound imaging,deep learning,segmentation
AI 理解论文
溯源树
样例
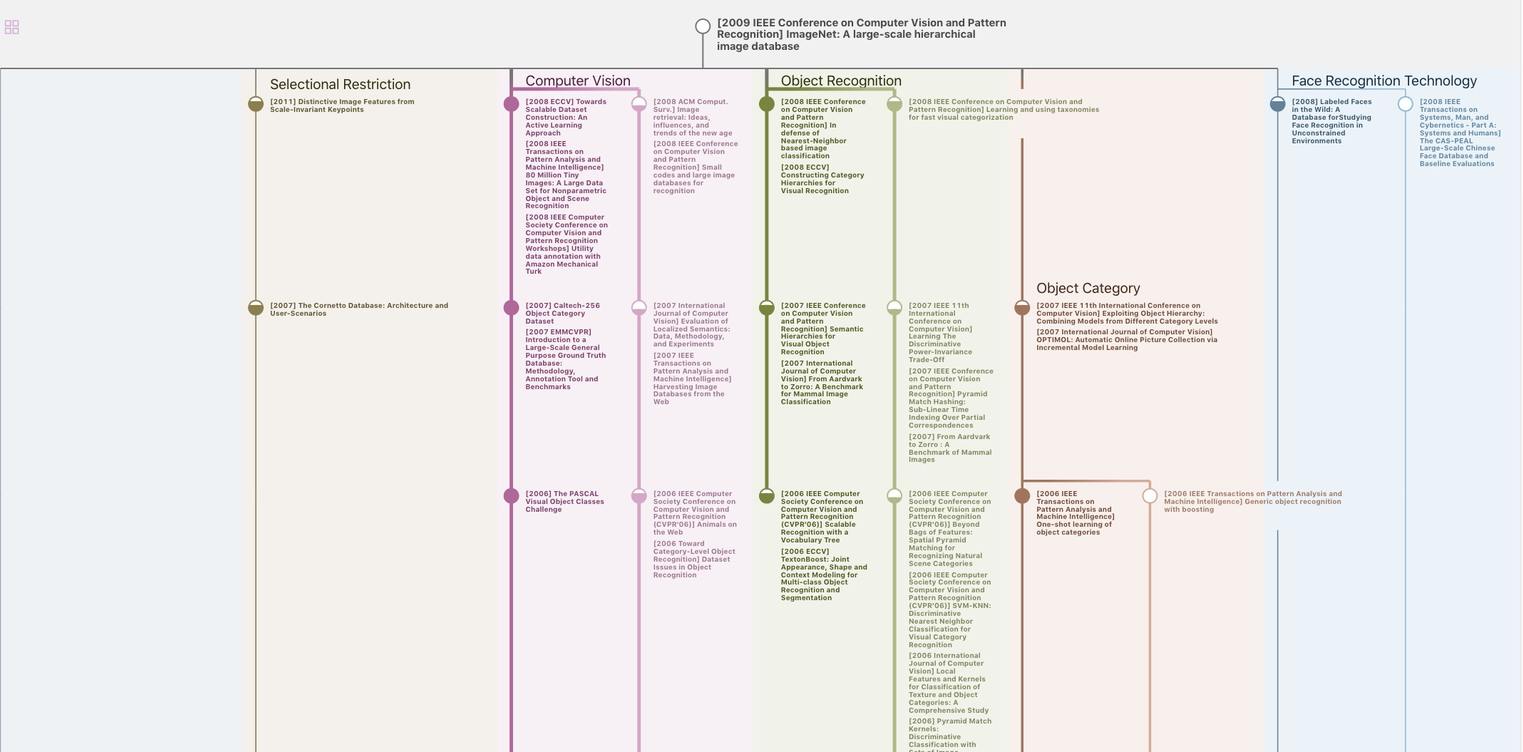
生成溯源树,研究论文发展脉络
Chat Paper
正在生成论文摘要