An Effective Co-support Guided Analysis Model for Multi-Contrast MRI Reconstruction.
IEEE journal of biomedical and health informatics(2023)
摘要
Multi-contrast magnetic resonance imaging (MRI) is widely used in clinical diagnosis. However, it is time-consuming to obtain MR data of multi-contrasts and the long scanning time may bring unexpected physiological motion artifacts. To obtain MR images of higher quality within limited acquisition time, we propose an effective model to reconstruct images from under-sampled k-space data of one contrast by utilizing another fully-sampled contrast of the same anatomy. Specifically, multiple contrasts from the same anatomical section exhibit similar structures. Enlightened by the fact that co-support of an image provides an appropriate characterization of morphological structures, we develop a similarity regularization of the co-supports across multi-contrasts. In this case, the guided MRI reconstruction problem is naturally formulated as a mixed integer optimization model consisting of three terms, the data fidelity of k-space, smoothness-enforcing regularization, and co-support regularization. An effective algorithm is developed to solve this minimization model alternatively. In the numerical experiments, T2-weighted images are used as the guidance to reconstruct T1-weighted/T2-weighted-Fluid-Attenuated Inversion Recovery (T2-FLAIR) images and PD-weighted images are used as the guidance to reconstruct PDFS-weighted images, respectively, from their under-sampled k-space data. The experimental results demonstrate that the proposed model outperforms other state-of-the-art multi-contrast MRI reconstruction methods in terms of both quantitative metrics and visual performance at various sampling ratios.
更多查看译文
关键词
Multi-contrast MRI,guided reconstruction,co-support,cosparse analysis model
AI 理解论文
溯源树
样例
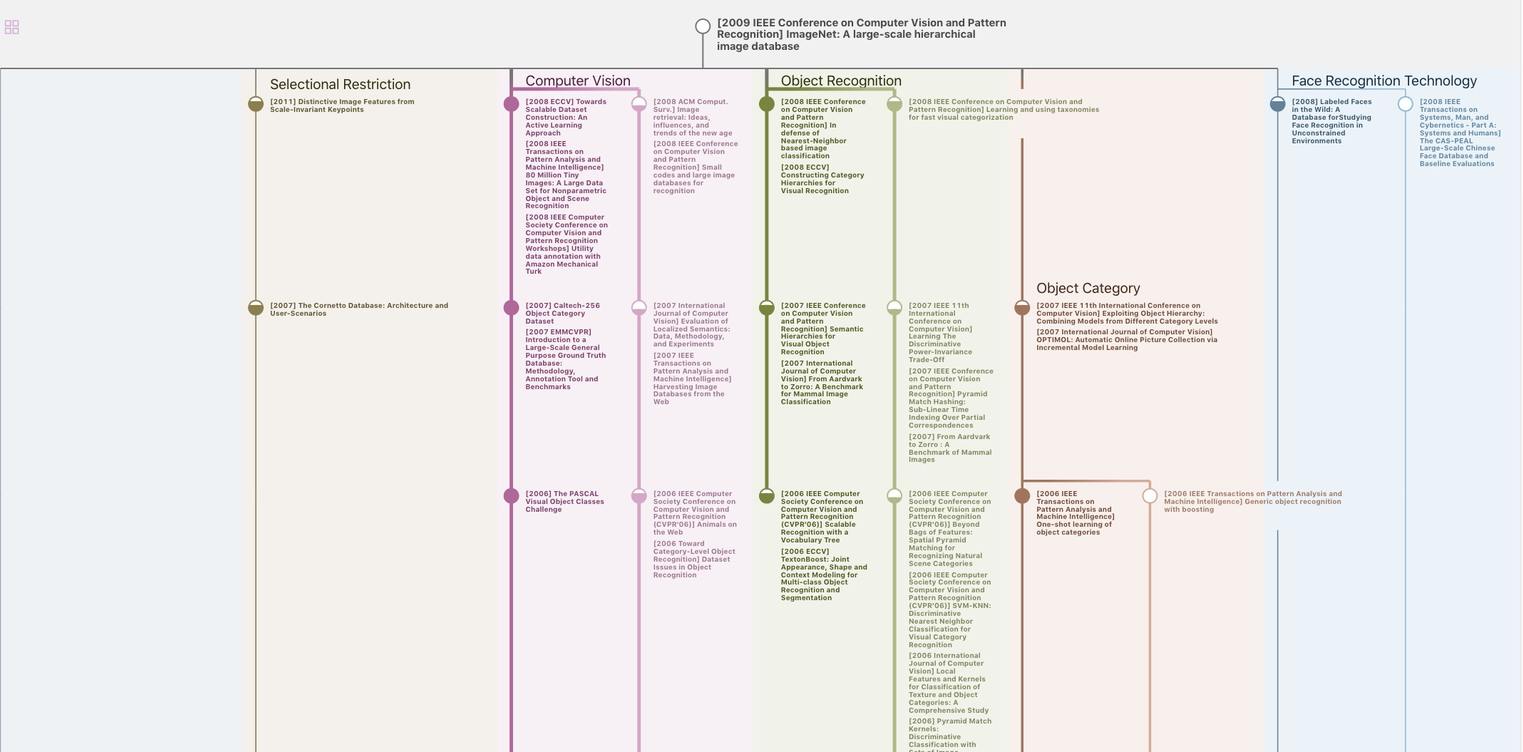
生成溯源树,研究论文发展脉络
Chat Paper
正在生成论文摘要