Consformer: Consciousness Detection Using Transformer Networks With Correntropy-Based Measures
IEEE transactions on neural systems and rehabilitation engineering : a publication of the IEEE Engineering in Medicine and Biology Society(2023)
摘要
Consciousness detection is important in diagnosis and treatment of disorders of consciousness (DOC). Recent studies have demonstrated that electroencephalography (EEG) signals contain effective information for consciousness state evaluation. We propose two novel EEG measures: the spatiotemporal correntropy and the neuromodulation intensity, to reflect the temporal-spatial complexity in brain signals for consciousness detection. Then, we build a pool of EEG measures with different spectral, complexity and connectivity features, and propose Consformer, a transformer network to learn an adaptive optimization of features for different subjects with the attention mechanism. Experiments are carried out using a large dataset of 280 resting-state EEG recordings of DOC patients. Consformer discriminates minimally conscious state (MCS) from vegetative state (VS) with an accuracy of 85.73% and an F1-score of 86.95%, which achieves the state-of-the-art performance.
更多查看译文
关键词
Consciousness detection, electroencephalogram, transformer, correntropy, machine learning
AI 理解论文
溯源树
样例
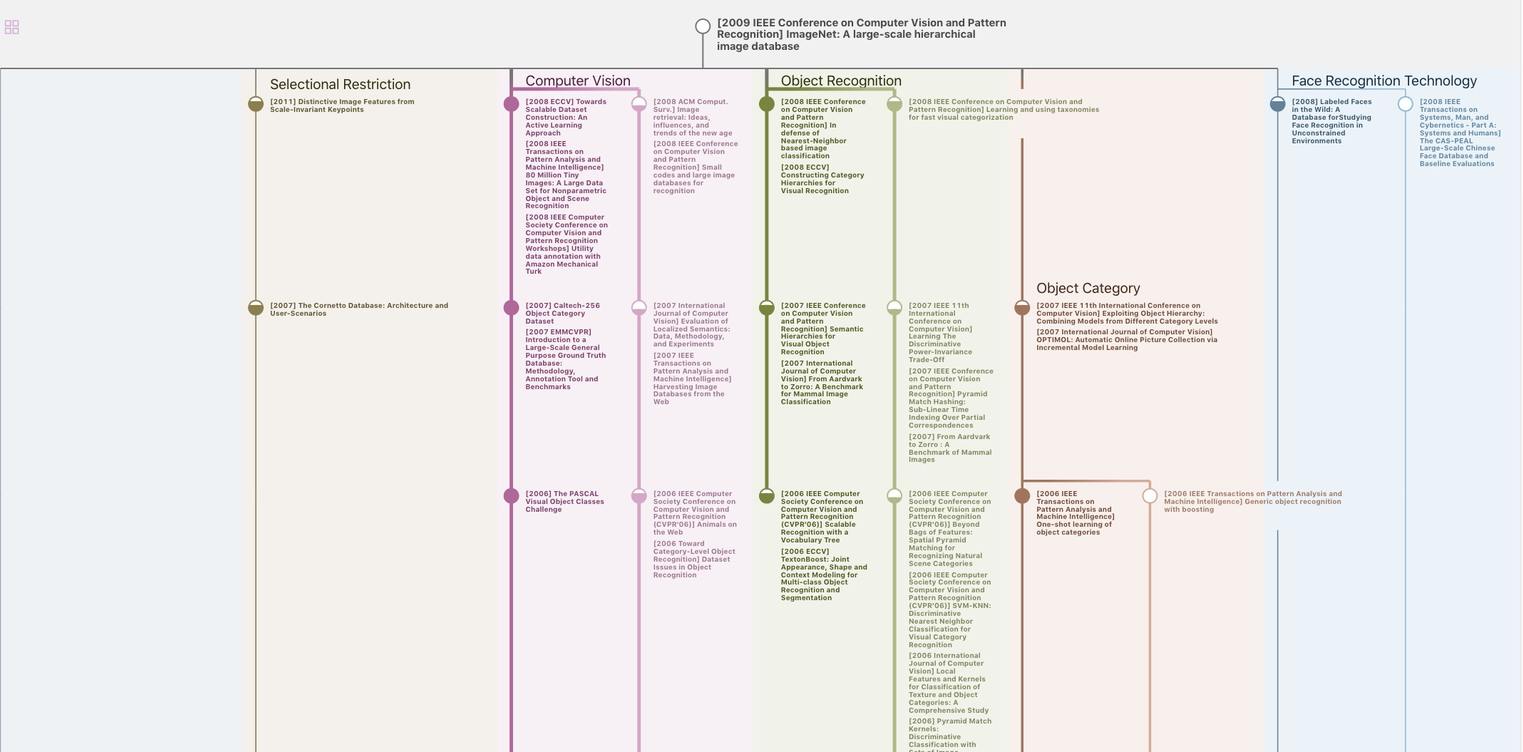
生成溯源树,研究论文发展脉络
Chat Paper
正在生成论文摘要