Robust and Privacy-Preserving Decentralized Deep Federated Learning Training: Focusing on Digital Healthcare Applications.
IEEE/ACM transactions on computational biology and bioinformatics(2023)
摘要
Federated learning of deep neural networks has emerged as an evolving paradigm for distributed machine learning, gaining widespread attention due to its ability to update parameters without collecting raw data from users, especially in digital healthcare applications. However, the traditional centralized architecture of federated learning suffers from several problems (e.g., single point of failure, communication bottlenecks, etc.), especially malicious servers inferring gradients and causing gradient leakage. To tackle the above issues, we propose a robust and privacy-preserving decentralized deep federated learning (RPDFL) training scheme. Specifically, we design a novel ring FL structure and a Ring-Allreduce-based data sharing scheme to improve the communication efficiency in RPDFL training. Furthermore, we improve the process of distributing parameters of the Chinese residual theorem to update the execution process of the threshold secret sharing, supporting healthcare edge to drop out during the training process without causing data leakage, and ensuring the robustness of the RPDFL training under the Ring-Allreduce-based data sharing scheme. Security analysis indicates that RPDFL is provable secure. Experiment results show that RPDFL is significantly superior to standard FL methods in terms of model accuracy and convergence, and is suitable for digital healthcare applications.
更多查看译文
关键词
Privacy-Preserving Federated Learning,Deep Learning,Robust Federated Learning,Decentralized Training,Digital Healthcare
AI 理解论文
溯源树
样例
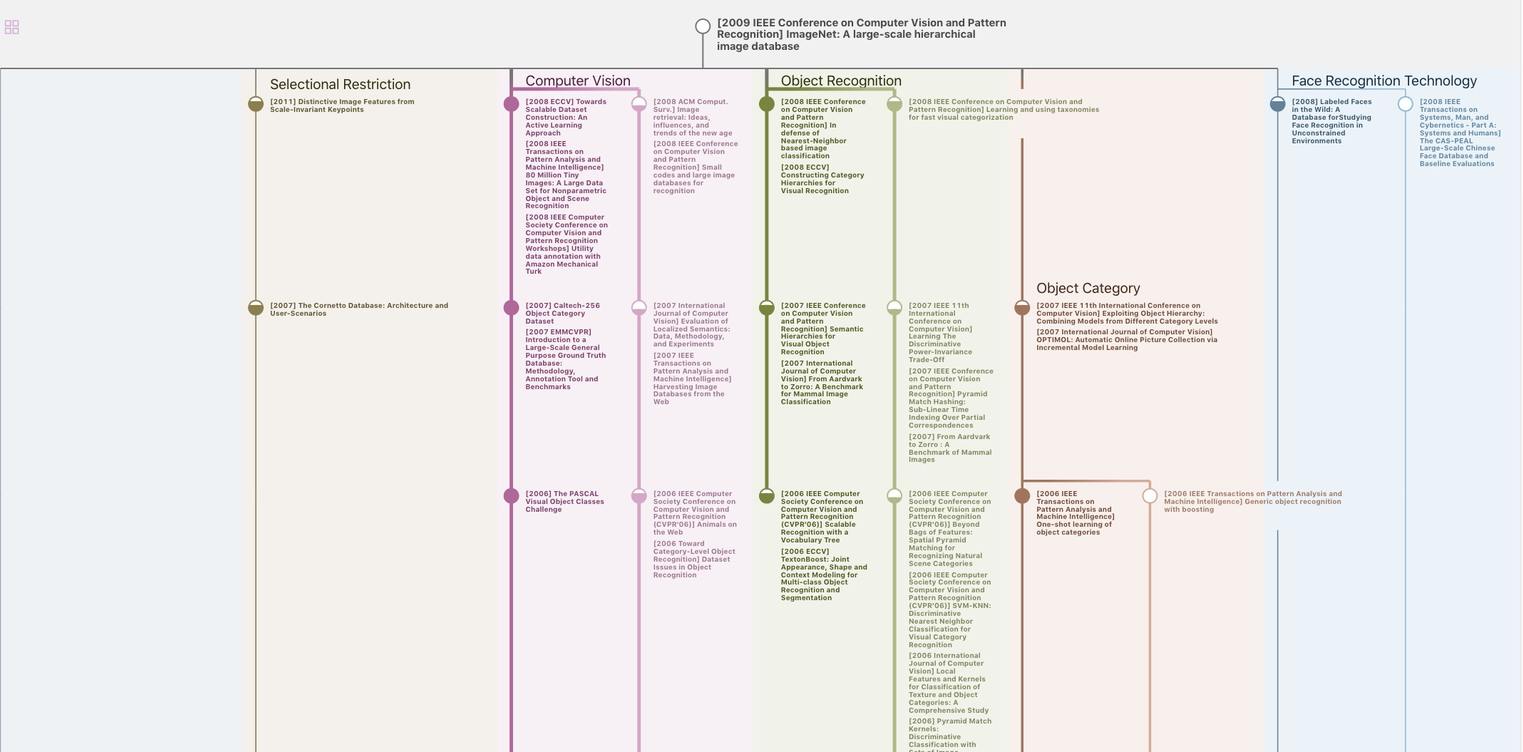
生成溯源树,研究论文发展脉络
Chat Paper
正在生成论文摘要